Compressive Subspace Learning With Antenna Cross-Correlations for Wideband Spectrum Sensing
IEEE Transactions on Communications(2020)
摘要
Compressive subspace learning (CSL) with the exploitation of space diversity has found a potential performance improvement for wideband spectrum sensing (WBSS). However, previous works mainly focus on either exploiting antenna auto-correlations or adopting a multiple-input multiple-output (MIMO) channel without considering the spatial correlations, which will degrade their performances. In this paper, we consider a spatially correlated MIMO channel and propose two CSL algorithms (i.e., mCSLSACC and vCSLACC) which exploit antenna cross-correlations, where the mCSLSACC utilizes an antenna averaging temporal decomposition, and the vCSLACC uses a spatial-temporal joint decomposition. For both algorithms, the conditions of statistical covariance matrices (SCMs) without noise corruption are derived. Through establishing the singular value relation of SCMs in statistical sense between the proposed and traditional CSL algorithms, we show the superiority of the proposed CSL algorithms. By further depicting the receiving correlation matrix of MIMO channel with the exponential correlation model, we give important closed-form expressions for the proposed CSL algorithms in terms of the amplification of singular values over traditional CSL algorithms. Such expressions provide a possibility to determine optimal algorithm parameters for high system performances in an analytical way. Simulations validate the correctness of this work and its performance improvement over existing works in terms of WBSS performance.
更多查看译文
关键词
Compressive subspace learning,wideband spectrum sensing,cognitive radio,MIMO,antenna cross-correlation
AI 理解论文
溯源树
样例
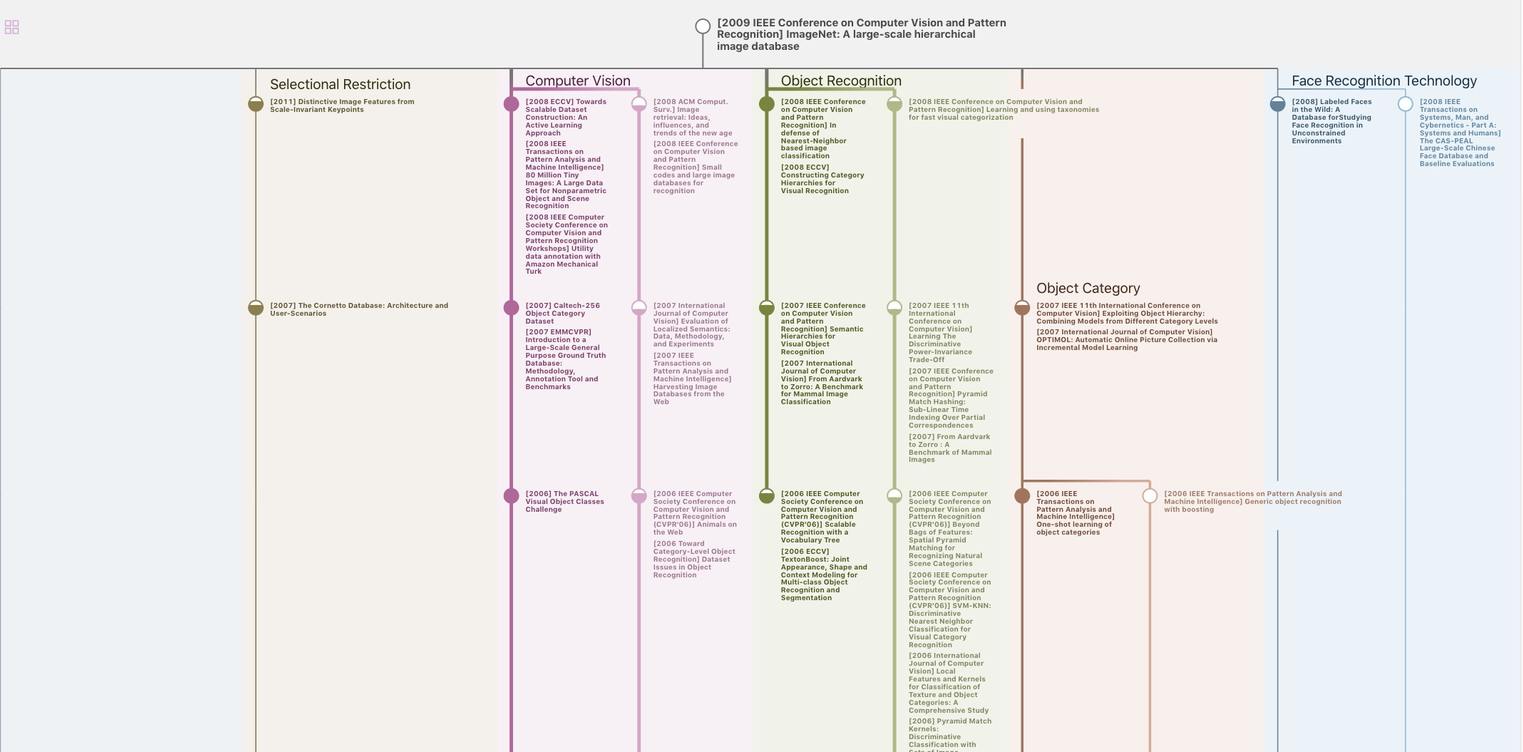
生成溯源树,研究论文发展脉络
Chat Paper
正在生成论文摘要