Automatic Multi-Catheter Detection using Deeply Supervised Convolutional Neural Network in MRI-guided HDR Prostate Brachytherapy.
MEDICAL PHYSICS(2020)
摘要
Purpose High-dose-rate (HDR) brachytherapy is an established technique to be used as monotherapy option or focal boost in conjunction with external beam radiation therapy (EBRT) for treating prostate cancer. Radiation source path reconstruction is a critical procedure in HDR treatment planning. Manually identifying the source path is labor intensive and time inefficient. In recent years, magnetic resonance imaging (MRI) has become a valuable imaging modality for image-guided HDR prostate brachytherapy due to its superb soft-tissue contrast for target delineation and normal tissue contouring. The purpose of this study is to investigate a deep-learning-based method to automatically reconstruct multiple catheters in MRI for prostate cancer HDR brachytherapy treatment planning. Methods Attention gated U-Net incorporated with total variation (TV) regularization model was developed for multi-catheter segmentation in MRI. The attention gates were used to improve the accuracy of identifying small catheter points, while TV regularization was adopted to encode the natural spatial continuity of catheters into the model. The model was trained using the binary catheter annotation images offered by experienced physicists as ground truth paired with original MRI images. After the network was trained, MR images of a new prostate cancer patient receiving HDR brachytherapy were fed into the model to predict the locations and shapes of all the catheters. Quantitative assessments of our proposed method were based on catheter shaft and tip errors compared to the ground truth. Results Our method detected 299 catheters from 20 patients receiving HDR prostate brachytherapy with a catheter tip error of 0.37 +/- 1.68 mm and a catheter shaft error of 0.93 +/- 0.50 mm. For detection of catheter tips, our method resulted in 87% of the catheter tips within an error of less than +/- 2.0 mm, and more than 71% of the tips can be localized within an absolute error of no >1.0 mm. For catheter shaft localization, 97% of catheters were detected with an error of <2.0 mm, while 63% were within 1.0 mm. Conclusions In this study, we proposed a novel multi-catheter detection method to precisely localize the tips and shafts of catheters in three-dimensional MRI images of HDR prostate brachytherapy. It paves the way for elevating the quality and outcome of MRI-guided HDR prostate brachytherapy.
更多查看译文
关键词
catheter detection,deep learning,magnetic resonance imaging,prostate brachytherapy,total variation regularization
AI 理解论文
溯源树
样例
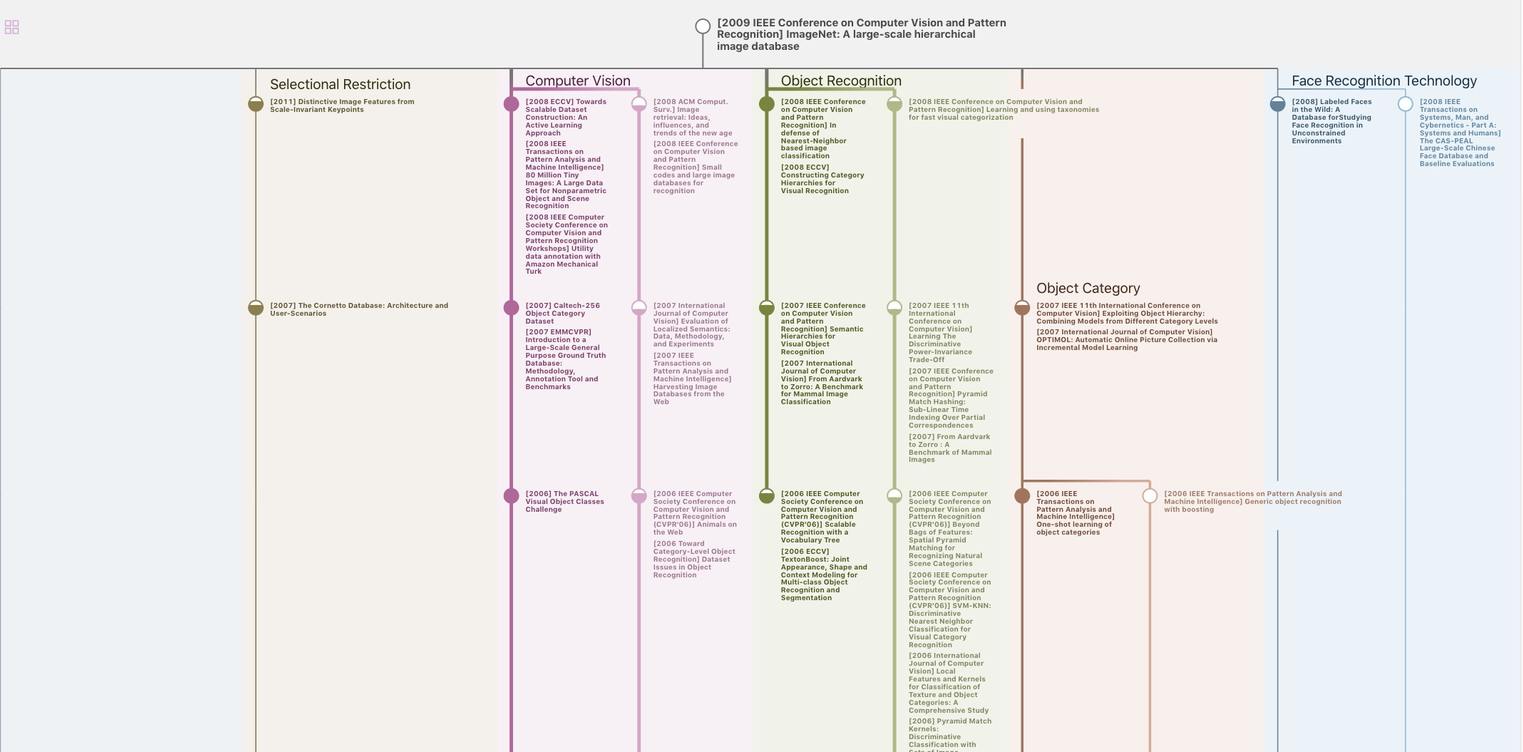
生成溯源树,研究论文发展脉络
Chat Paper
正在生成论文摘要