A comparative study of 2D image segmentation algorithms for traumatic brain lesions using CT data from the ProTECTIII multicenter clinical trial
Proceedings of SPIE(2020)
摘要
Automated segmentation of medical imaging is of broad interest to clinicians and machine learning researchers alike. The goal of segmentation is to increase efficiency and simplicity of visualization and quantification of regions of interest within a medical image. Image segmentation is a difficult task because of multiparametric heterogeneity within the images, an obstacle that has proven especially challenging in efforts to automate the segmentation of brain lesions from non-contrast head computed tomography (CT). In this research, we have experimented with multiple available deep learning architectures to segment different phenotypes of hemorrhagic lesions found after moderate to severe traumatic brain injury (TBI). These include: intraparenchymal hemorrhage (IPH), subdural hematoma (SDH), epidural hematoma (EDH), and traumatic contusions. We were able to achieve an optimal Dice Coefficient score of 0.94 using UNet++ 2D Architecture with Focal Tversky Loss Function, an increase from 0.85 using UNet 2D with Binary Cross-Entropy Loss Function in intraparenchymal hemorrhage (IPH) cases. Furthermore, using the same setting, we were able to achieve the Dice Coefficient score of 0.90 and 0.86 in cases of Extra-Axial bleeds and Traumatic contusions, respectively.
更多查看译文
关键词
Automated segmentation,traumatic brain injury,non-contrast head computed tomography,ProTECTIII,methods comparison
AI 理解论文
溯源树
样例
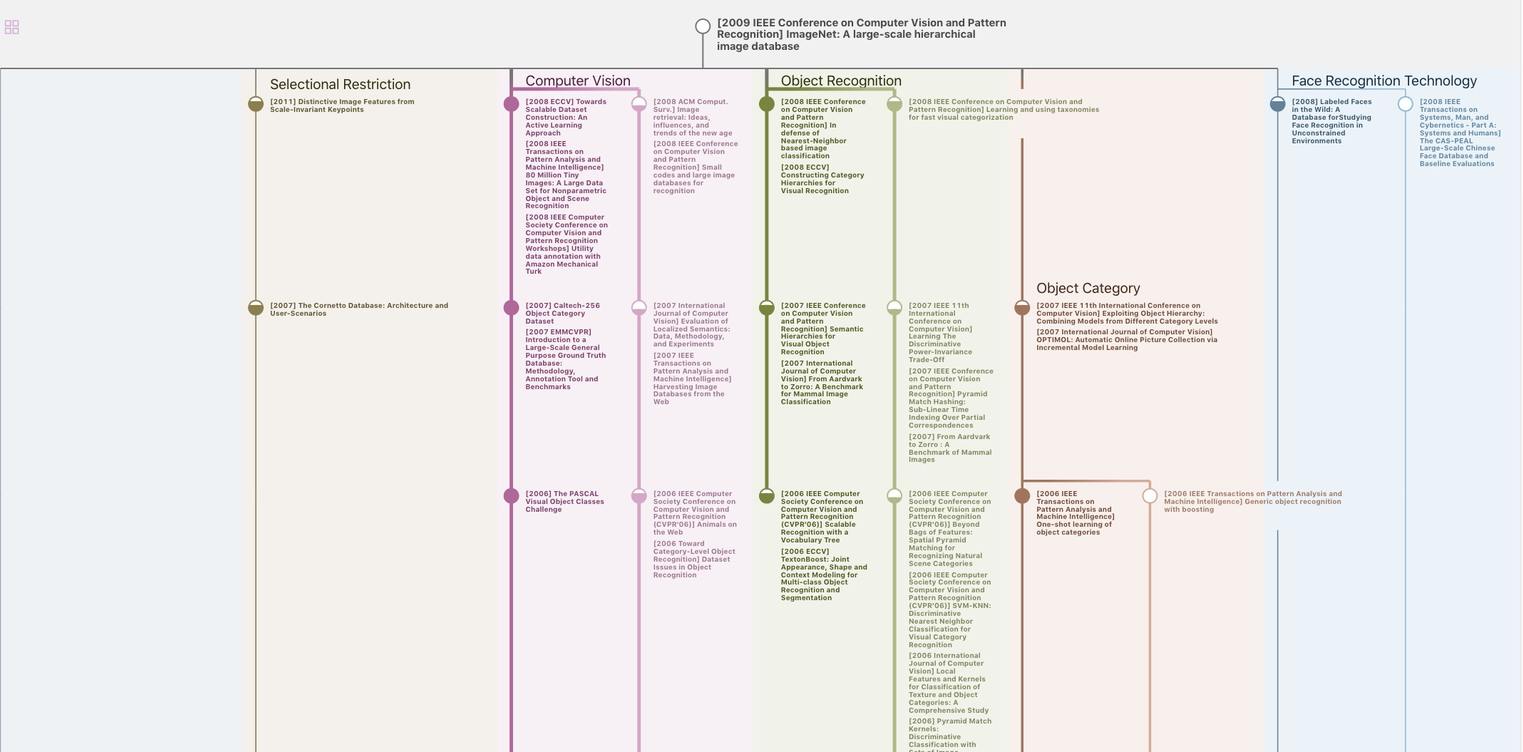
生成溯源树,研究论文发展脉络
Chat Paper
正在生成论文摘要