Incentive Mechanism Design for Resource Sharing in Collaborative Edge Learning
arxiv(2020)
摘要
In 5G and Beyond networks, Artificial Intelligence applications are expected to be increasingly ubiquitous. This necessitates a paradigm shift from the current cloud-centric model training approach to the Edge Computing based collaborative learning scheme known as edge learning, in which model training is executed at the edge of the network. In this article, we first introduce the principles and technologies of collaborative edge learning. Then, we establish that a successful, scalable implementation of edge learning requires the communication, caching, computation, and learning resources (3C-L) of end devices and edge servers to be leveraged jointly in an efficient manner. However, users may not consent to contribute their resources without receiving adequate compensation. In consideration of the heterogeneity of edge nodes, e.g., in terms of available computation resources, we discuss the challenges of incentive mechanism design to facilitate resource sharing for edge learning. Furthermore, we present a case study involving optimal auction design using Deep Learning to price fresh data contributed for edge learning. The performance evaluation shows the revenue maximizing properties of our proposed auction over the benchmark schemes.
更多查看译文
关键词
collaborative edge learning,resource sharing
AI 理解论文
溯源树
样例
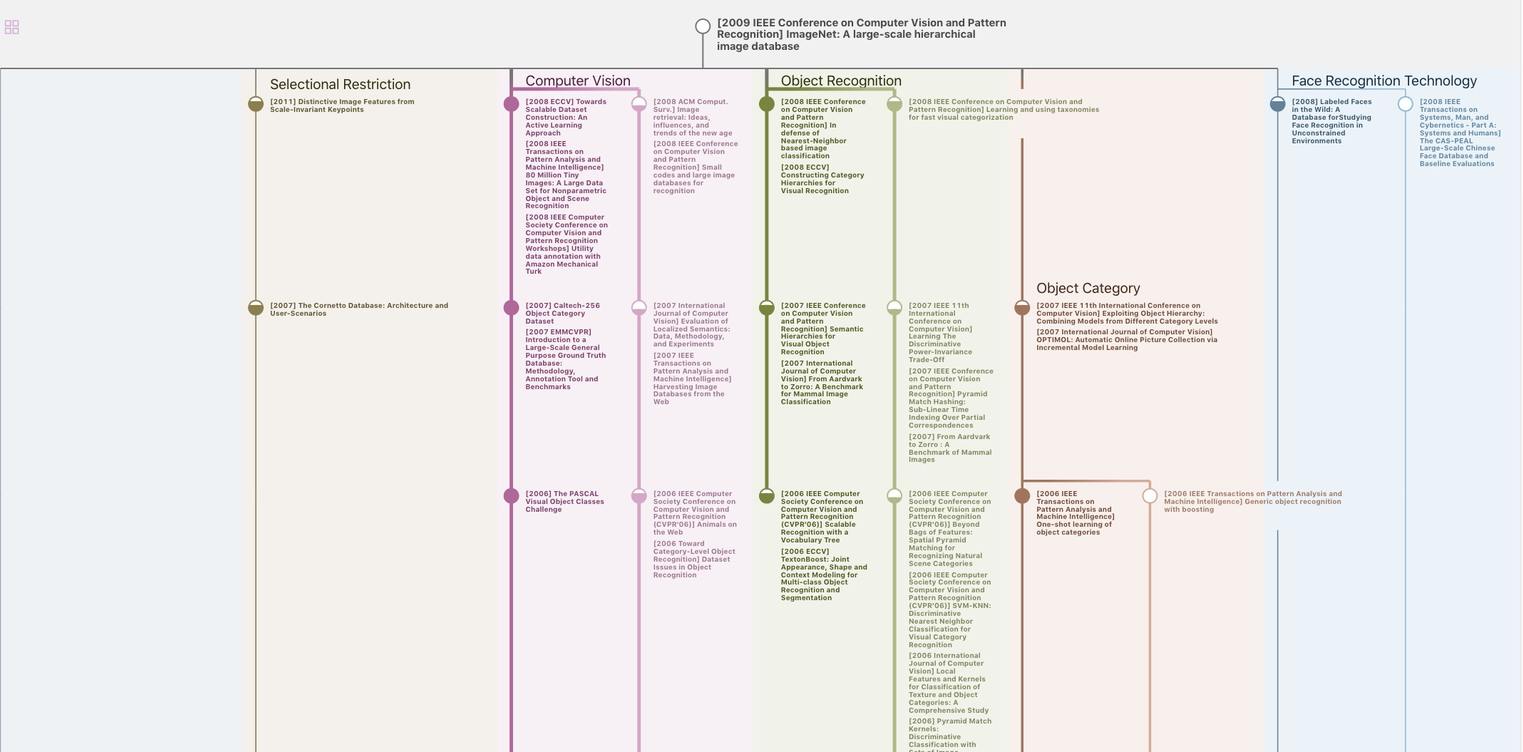
生成溯源树,研究论文发展脉络
Chat Paper
正在生成论文摘要