Fast Enhancement for Non-Uniform Illumination Images using Light-weight CNNs
MM '20: The 28th ACM International Conference on Multimedia Seattle WA USA October, 2020(2020)
摘要
This paper proposes a new light-weight convolutional neural network (~5k params) for non-uniform illumination image enhancement to handle color, exposure, contrast, noise and artifacts, etc., simultaneously and effectively. More concretely, the input image is first enhanced using Retinex model from dual different aspects (enhancing under-exposure and suppressing over-exposure), respectively. Then, these two enhanced results and the original image are fused to obtain an image with satisfactory brightness, contrast and details. Finally, the extra noise and compression artifacts are removed to get the final result. To train this network, we propose a semi-supervised retouching solution and construct a new dataset (~82k images) that contains various scenes and light conditions. Our model can enhance 0.5 mega-pixel (like 600×800) images in real-time (~50 fps), which is faster than existing enhancement methods. Extensive experiments show that our solution is fast and effective to deal with non-uniform illumination images.
更多查看译文
关键词
Non-uniform Illumination, Fast Enhancement, Light-weight CNNs
AI 理解论文
溯源树
样例
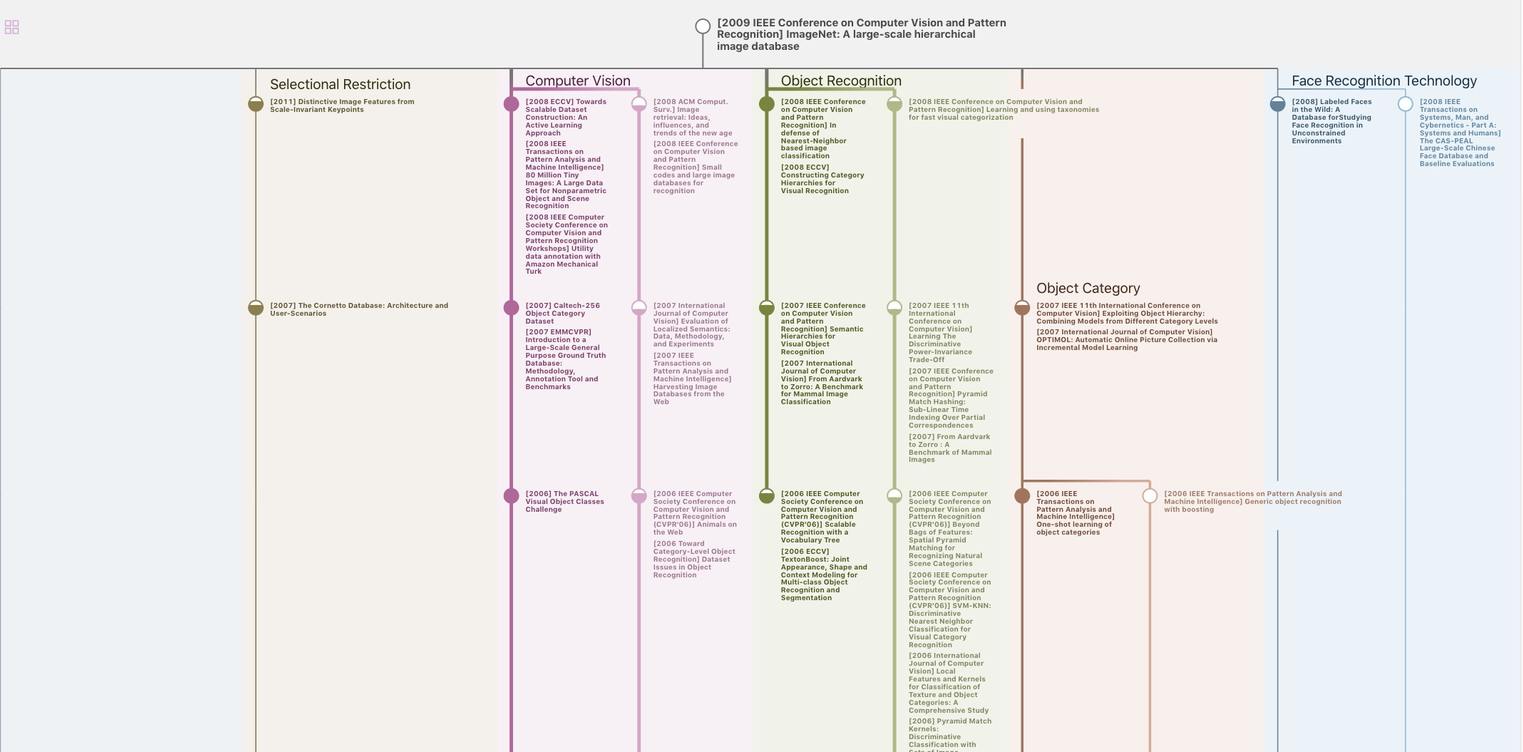
生成溯源树,研究论文发展脉络
Chat Paper
正在生成论文摘要