Self-supervised Structure-Sensitive Learning for Human Parsing
Human Centric Visual Analysis with Deep Learning(2020)
摘要
Human parsing has recently attracted much research interest due to its enormous application potential. In this chapter, we introduce a new benchmark,“Look into Person (LIP),” that makes a significant advance in terms of scalability, diversity, and difficulty, a contribution that we feel is crucial for future developments in human-centric analysis. Furthermore, in contrast to the existing efforts to improve feature discriminative capability, we solve human parsing by exploring a novel self-supervised structure-sensitive learning approach that imposes human pose structures on the parsing results without requiring extra supervision. Our self-supervised learning framework can be injected into any advanced neural network to help incorporate rich high-level knowledge regarding human joints from a global perspective and improve the parsing results (©[2019] IEEE., Reprinted, with permission, from [1]).
更多查看译文
AI 理解论文
溯源树
样例
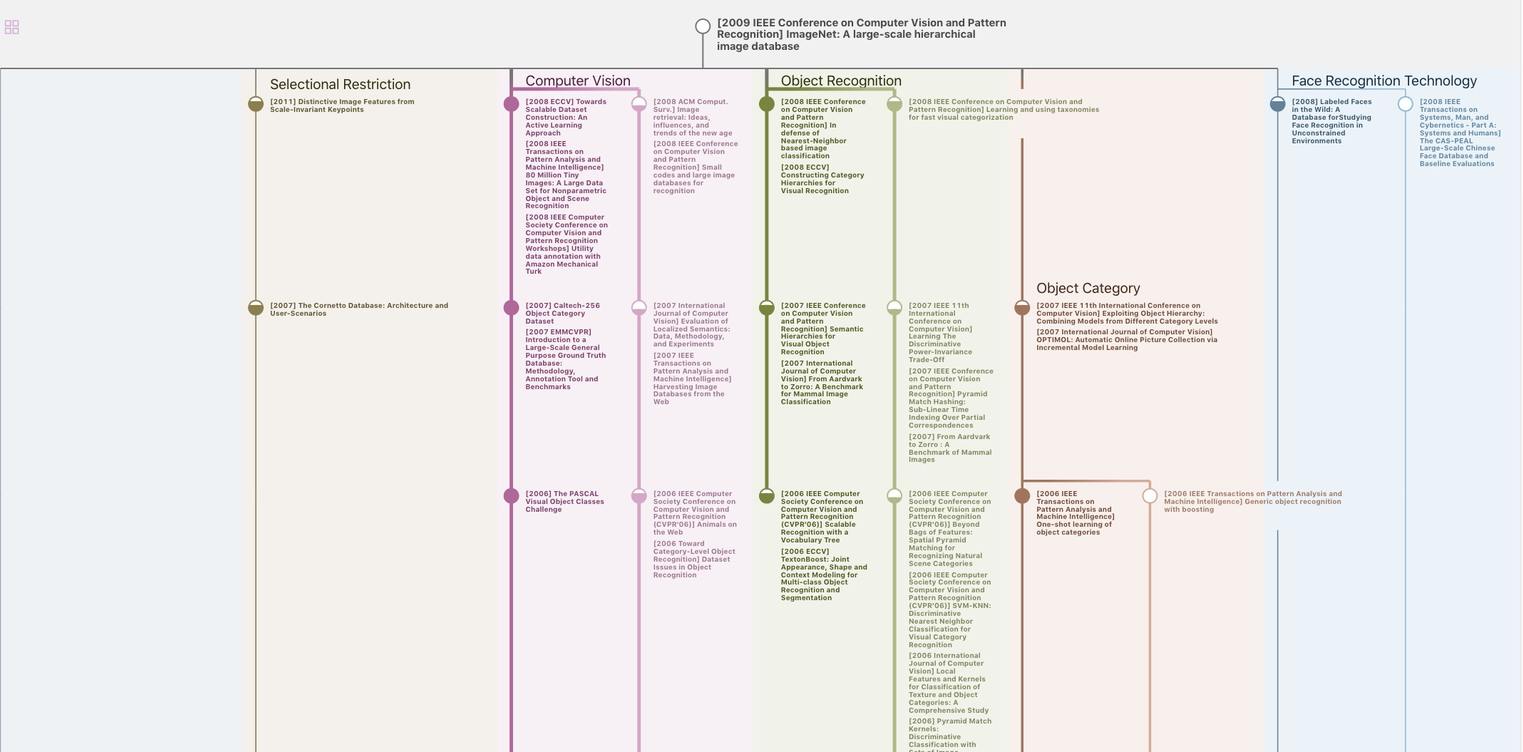
生成溯源树,研究论文发展脉络
Chat Paper
正在生成论文摘要