Towards Question-based Recommender Systems
SIGIR '20: The 43rd International ACM SIGIR conference on research and development in Information Retrieval Virtual Event China July, 2020(2020)
摘要
Conversational and question-based recommender systems have gained increasing attention in recent years, with users enabled to converse with the system and better control recommendations. Nevertheless, research in the field is still limited, compared to traditional recommender systems. In this work, we propose a novel Question-based recommendation method, Qrec, to assist users to find items interactively, by answering automatically constructed and algorithmically chosen questions. Previous conversational recommender systems ask users to express their preferences over items or item facets. Our model, instead, asks users to express their preferences over descriptive item features. The model is first trained offline by a novel matrix factorization algorithm, and then iteratively updates the user and item latent factors online by a closed-form solution based on the user answers. Meanwhile, our model infers the underlying user belief and preferences over items to learn an optimal question-asking strategy by using Generalized Binary Search, so as to ask a sequence of questions to the user. Our experimental results demonstrate that our proposed matrix factorization model outperforms the traditional Probabilistic Matrix Factorization model. Further, our proposed Qrec model can greatly improve the performance of state-of-the-art baselines, and it is also effective in the case of cold-start user and item recommendations.
更多查看译文
关键词
Conversational Recommender Systems, Question-based Recommender Systems, Matrix Factorization, Cold-start Problem
AI 理解论文
溯源树
样例
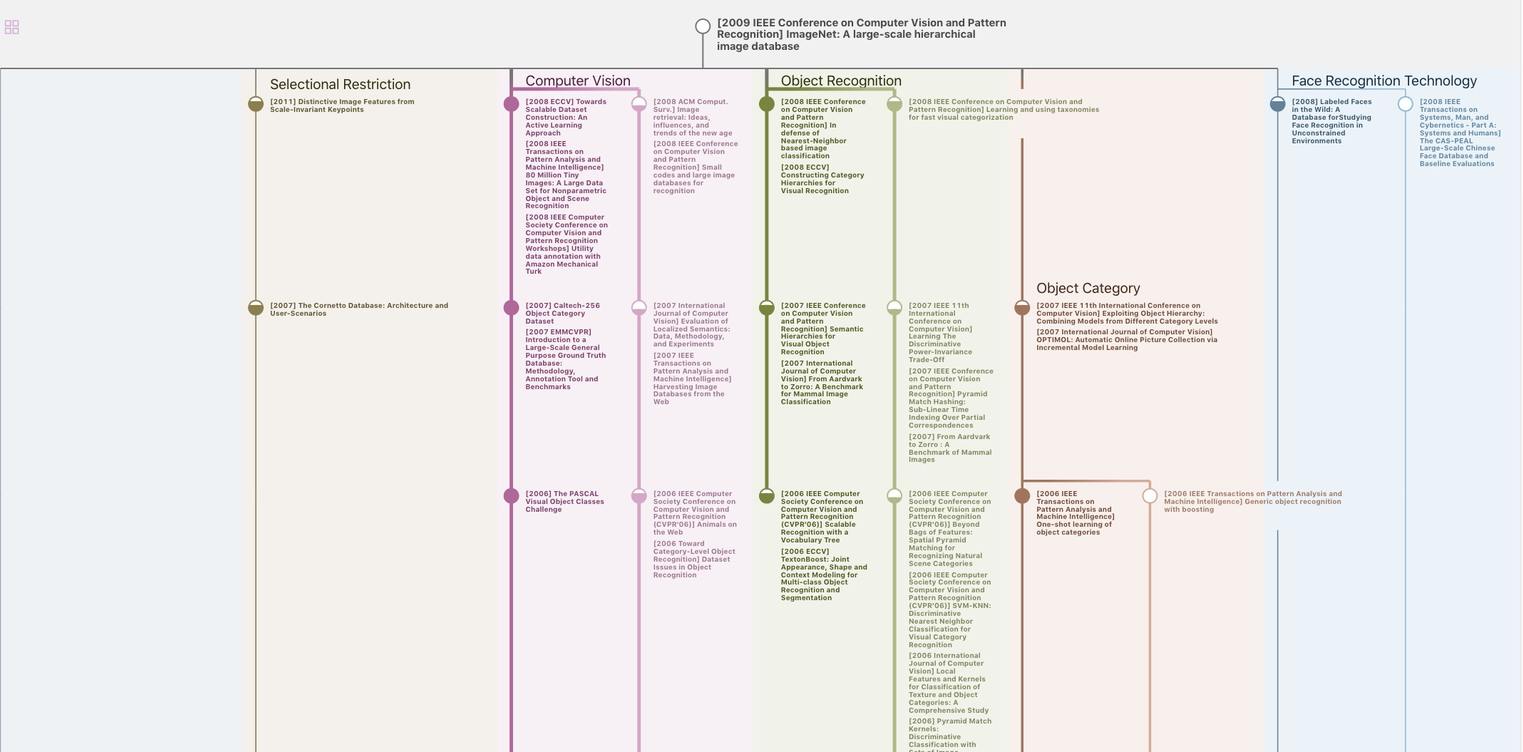
生成溯源树,研究论文发展脉络
Chat Paper
正在生成论文摘要