Counterfactual clinical prediction models could help to infer individualized treatment effects in randomized controlled trials-An illustration with the International Stroke Trial.
Journal of clinical epidemiology(2020)
摘要
OBJECTIVE:Causal treatment effects are estimated at the population level in randomized controlled trials, while clinical decision is often to be made at the individual level in practice. We aim to show how clinical prediction models used under a counterfactual framework may help to infer individualized treatment effects.
STUDY DESIGN AND SETTING:As an illustrative example, we reanalyze the International Stroke Trial. This large, multicenter trial enrolled 19,435 adult patients with suspected acute ischemic stroke from 36 countries, and reported a modest average benefit of aspirin (vs. no aspirin) on a composite outcome of death or dependency at 6 months. We derive and validate multivariable logistic regression models that predict the patient counterfactual risks of outcome with and without aspirin, conditionally on 23 predictors.
RESULTS:The counterfactual prediction models display good performance in terms of calibration and discrimination (validation c-statistics: 0.798 and 0.794). Comparing the counterfactual predicted risks on an absolute difference scale, we show that aspirin-despite an average benefit-may increase the risk of death or dependency at 6 months (compared with the control) in a quarter of stroke patients.
CONCLUSIONS:Counterfactual prediction models could help researchers and clinicians (i) infer individualized treatment effects and (ii) better target patients who may benefit from treatments.
更多查看译文
AI 理解论文
溯源树
样例
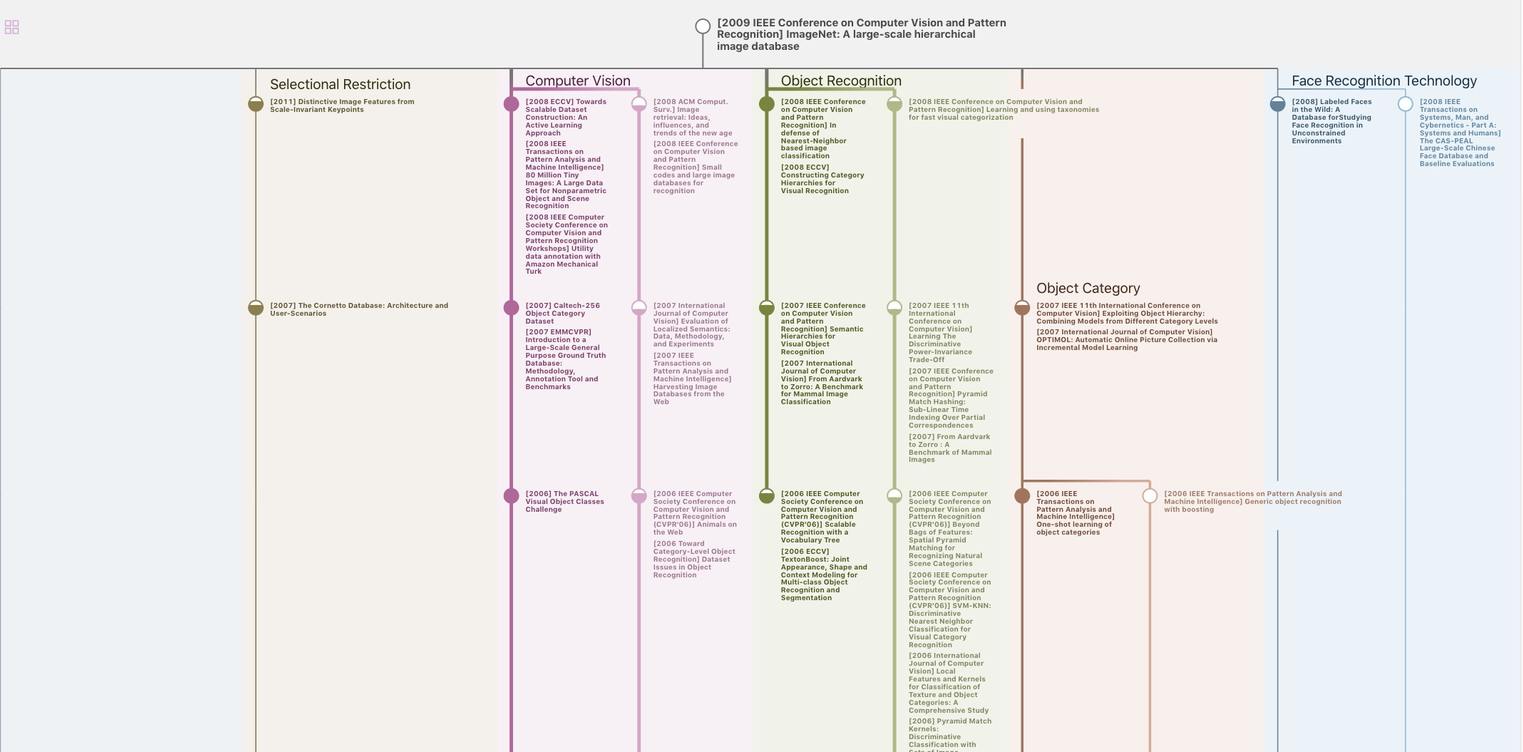
生成溯源树,研究论文发展脉络
Chat Paper
正在生成论文摘要