Filling Missing Values On Wearable-Sensory Time Series Data
PROCEEDINGS OF THE 2020 SIAM INTERNATIONAL CONFERENCE ON DATA MINING (SDM)(2020)
摘要
Missing data points is a common problem associated with data collected from wearables. This problem is particularly compounded if different subjects have different aspects of missingness associated with them {that is varying degrees of compliance behavior of individuals (participants) with respect to wearables as well as personal changes in lifestyle and health impacting heart rate. Moreover, despite the varying degree of compliance behavior, the wearable in itself might have glitches that lead to observations being dropped. Thus, any missing value imputation in such data has to not only generalize to the wearable behavior but also to the participant behavior. In this paper, we present a deep learning based approach for imputing missing values in heart rate time series data collected from a participant's wearable. In particular, for each participant, we first leverage his/her historical heart rate records as a reference set to extract the underlying personalized characteristics, and then impute the missing heart rate values by considering both contextual information of the current observations and the user's features learned from previous records. Adversarial training is applied to guide the learning process, which imputed more reasonable heart rate series with the consideration of human health conditions, e.g., heart rate fluctuations. Extensive experiments are conducted on two real-world data to show the superiority of our proposed method over state-of-the-art baselines.
更多查看译文
AI 理解论文
溯源树
样例
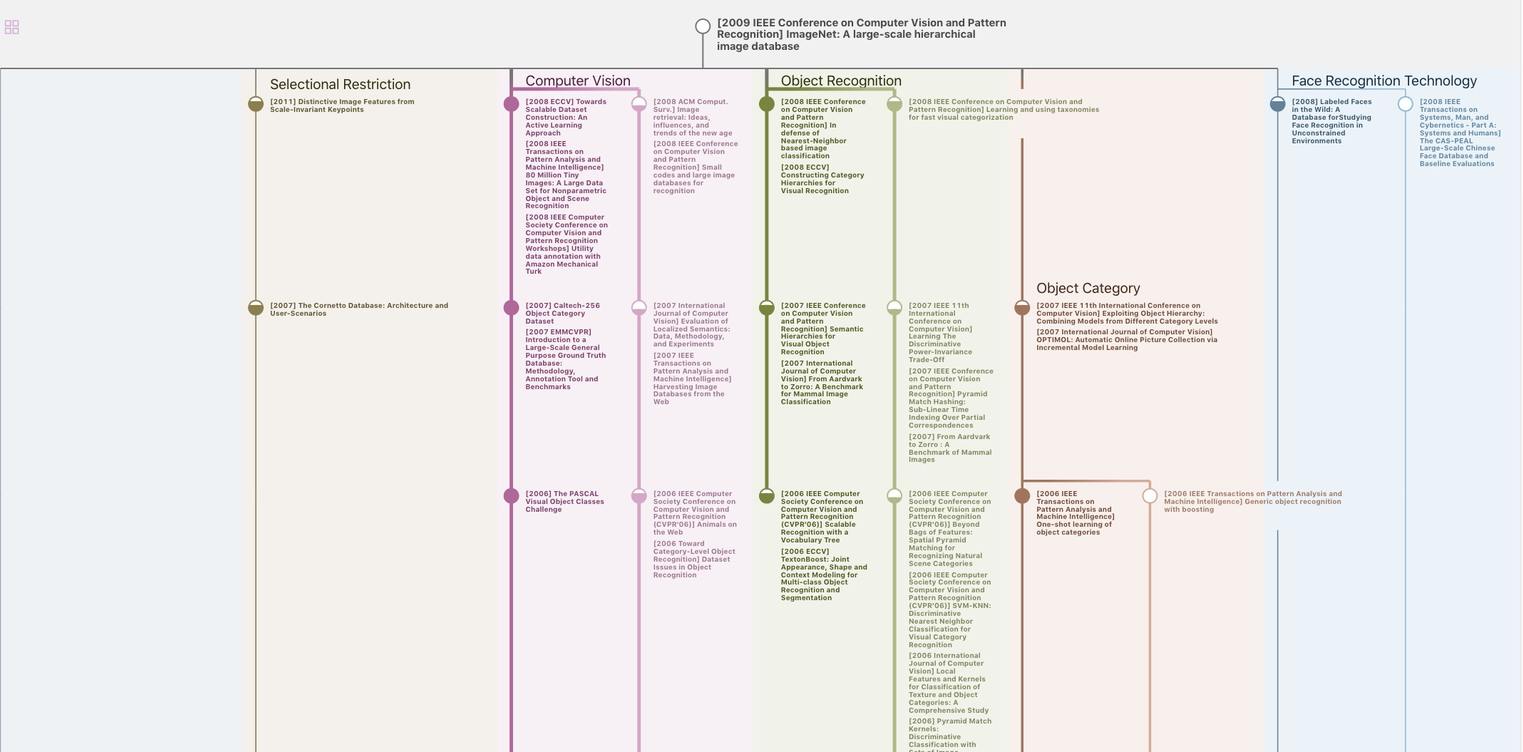
生成溯源树,研究论文发展脉络
Chat Paper
正在生成论文摘要