Deep Matrix Tri-Factorization: Mining Vertex-Wise Interactions In Multi-Space Attributed Graphs
PROCEEDINGS OF THE 2020 SIAM INTERNATIONAL CONFERENCE ON DATA MINING (SDM)(2020)
摘要
Mining vertex-wise interactions in graphs helps reveal useful information in real-world applications, such as bioinformatics networks BioGRID and DrugBank and academic networks DBLP and Arxiv. A main challenge in developing a general learning method for this setting is that each vertex may be associated with features from heterogeneous feature spaces, representing very disparate information. Moreover, features could be raw and low-level, leading to sparse representations. Some solutions in this area treat all feature spaces as equally important and concatenate features from heterogeneous feature spaces into a single feature vector. Others harmonize different feature spaces by respecting their relative significance in mining vertex-wise interactions but requiring construct specialized harmonizing function and/or handcrafting expressive features, both of which entail expert knowledge. Motivated by this observation, we propose a new learning paradigm named Deep Matrix Tri-Factorization (DM3F), which draws insights from deep models: (i) DM3F replaces the linear combination with a neural architecture that can learn an arbitrary harmonizing function from data; and (ii) DM3F allows raw feature inputs and automatically extracts high-level feature representations via a layer-bylayer learning mechanism. These two characteristics of DM3F make it accessible for users without expert knowledge. DM3F includes two orthogonal and complementary models, allowing an ensemble mechanism to optimize its performance during both training and predicting. A theoretical analysis of DM3F reveals that it possesses several desirable properties, including that it strictly generalizes matrix factorization models. We demonstrate the performance of DM3F on two real-world datasets.
更多查看译文
关键词
Attributed graph, Heterogenous feature space, Matrix tri-factorization, Drug-drug interaction, Co-author network
AI 理解论文
溯源树
样例
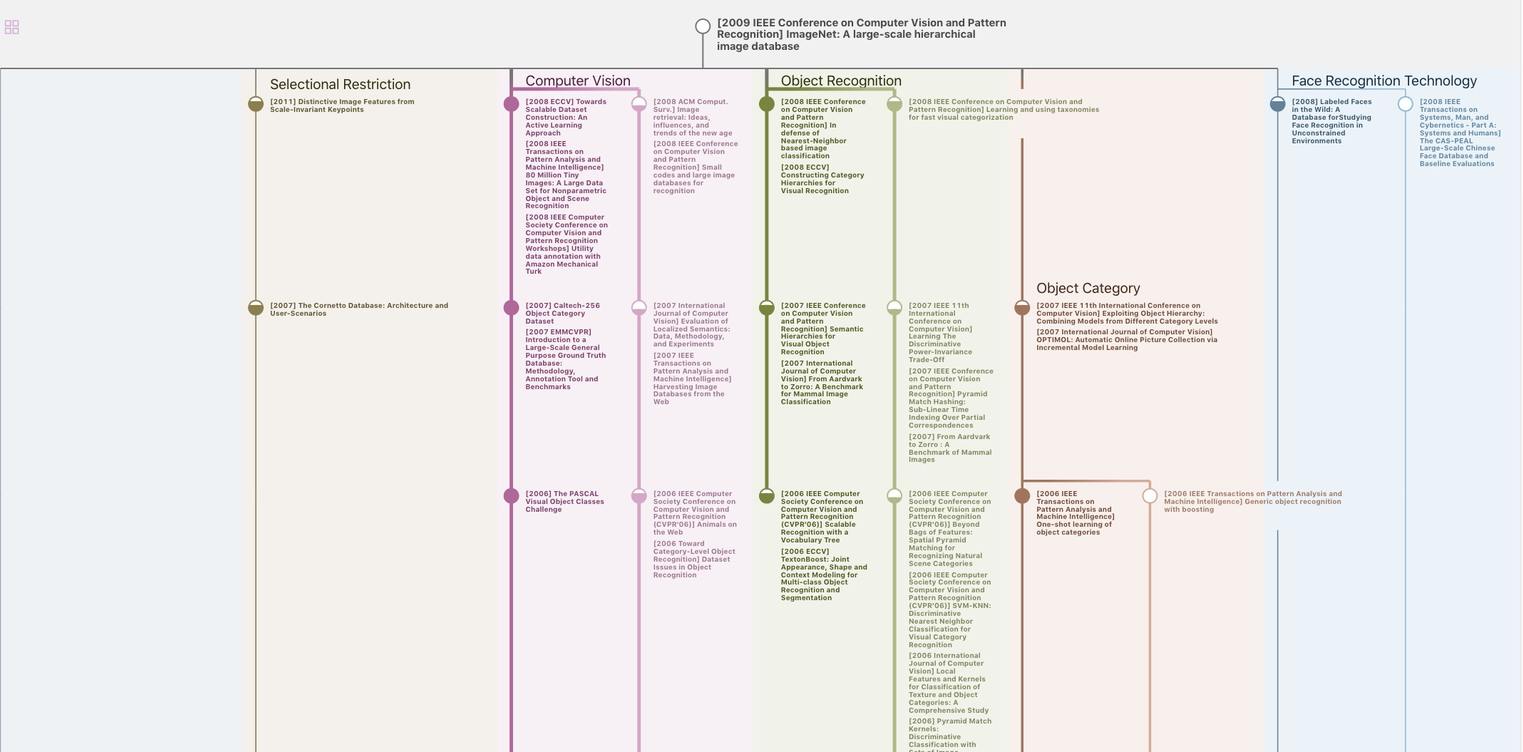
生成溯源树,研究论文发展脉络
Chat Paper
正在生成论文摘要