Unsupervised Bootstrapping of Active Learning for Entity Resolution.
ESWC(2020)
摘要
Entity resolution is one of the central challenges when integrating data from large numbers of data sources. Active learning for entity resolution aims to learn high-quality matching models while minimizing the human labeling effort by selecting only the most informative record pairs for labeling. Most active learning methods proposed so far, start with an empty set of labeled record pairs and iteratively improve the prediction quality of a classification model by asking for new labels. The absence of adequate labeled data in the early active learning iterations leads to unstable models of low quality which is known as the cold start problem. In our work we solve the cold start problem using an unsupervised matching method to bootstrap active learning. We implement a thresholding heuristic that considers pre-calculated similarity scores and assigns matching labels with some degree of noise at no manual labeling cost. The noisy labels are used for initializing the active learning process and throughout the whole active learning cycle for model learning and query selection. We evaluate our pipeline with six datasets from three different entity resolution settings using active learning with a committee-based query strategy and show it successfully deals with the cold start problem. Comparing our method against two active learning baselines without bootstrapping, we show that it can additionally lead to overall improved learned models in terms of F-1 score and stability.
更多查看译文
关键词
active learning,unsupervised bootstrapping,resolution
AI 理解论文
溯源树
样例
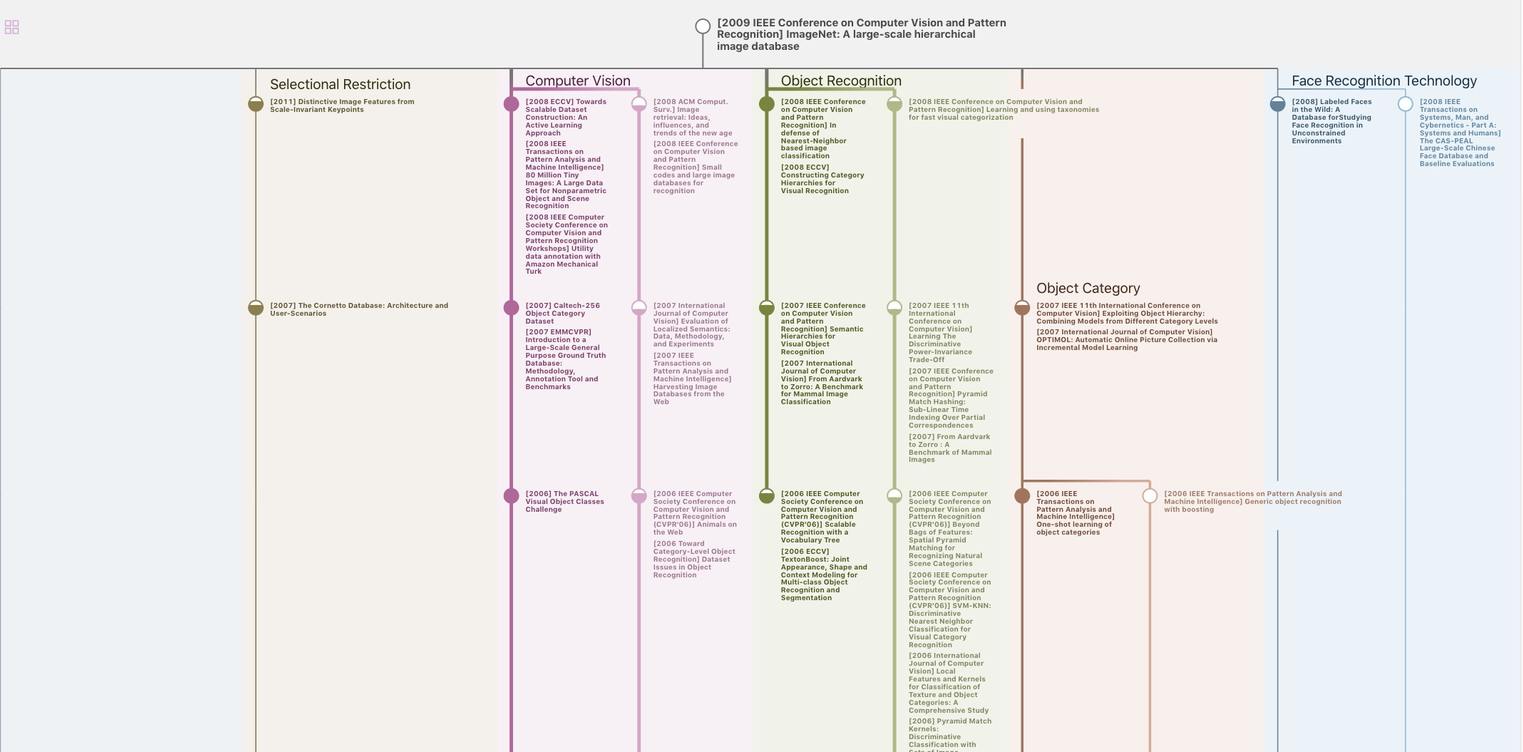
生成溯源树,研究论文发展脉络
Chat Paper
正在生成论文摘要