Entity Summarization with User Feedback.
ESWC(2020)
摘要
Semantic Web applications have benefited from entity summarization techniques which compute a compact summary for an entity by selecting a set of key triples from underlying data. A wide variety of entity summarizers have been developed. However, the quality of summaries they generate are still not satisfying, and we lack mechanisms for improving computed summaries. To address this challenge, in this paper we present the first study of entity summarization with user feedback. We consider a cooperative environment where a user reads the current entity summary and provides feedback to help an entity summarizer compute an improved summary. Our approach represents this iterative process as a Markov decision process where the entity summarizer is modeled as a reinforcement learning agent. To exploit user feedback, we represent the interdependence of triples in the current summary and the user feedback by a novel deep neural network which is incorporated into the policy of the agent. Our approach outperforms five baseline methods in extensive experiments with both real users and simulated users.
更多查看译文
关键词
Entity summarization, User feedback, Reinforcement learning, Deep neural network
AI 理解论文
溯源树
样例
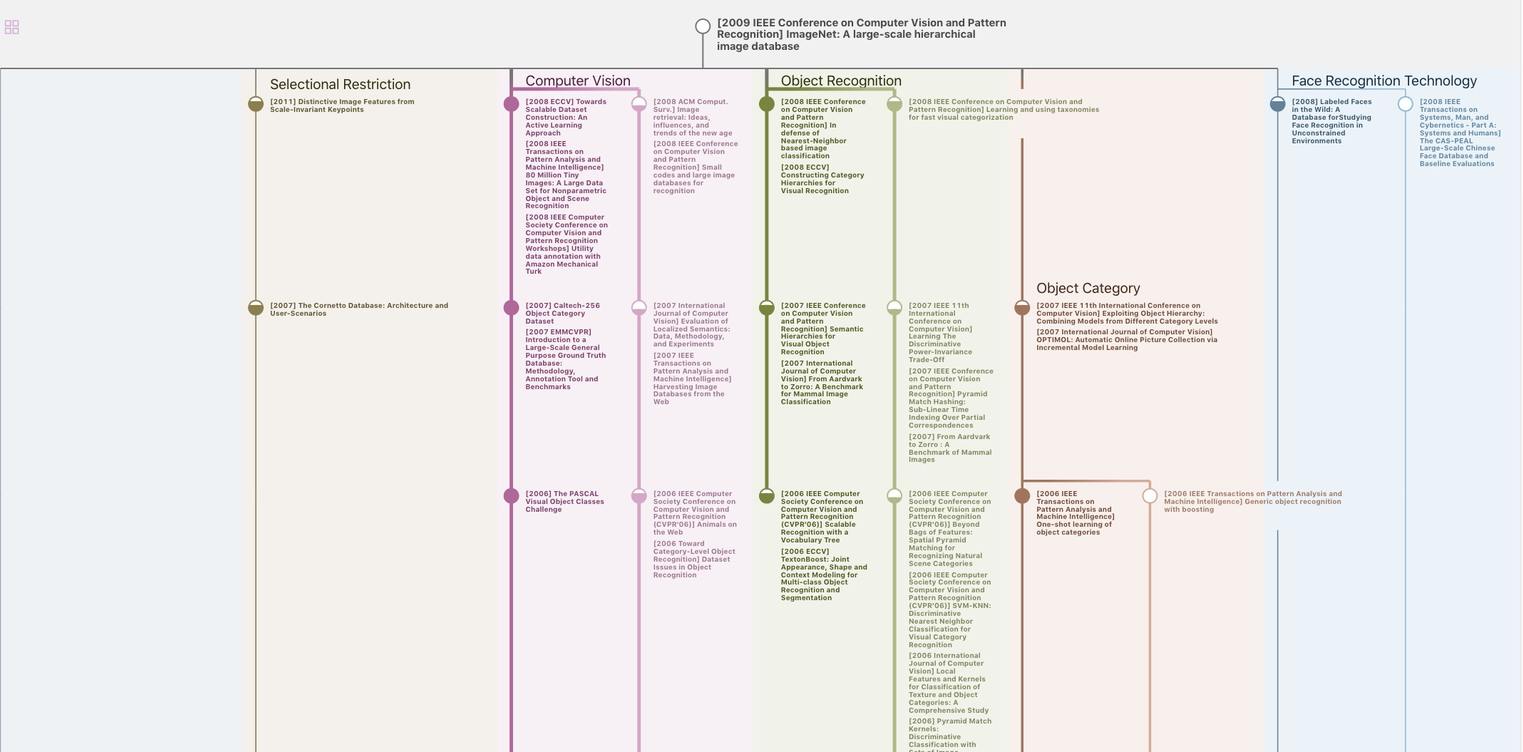
生成溯源树,研究论文发展脉络
Chat Paper
正在生成论文摘要