SchemaTree - Maximum-Likelihood Property Recommendation for Wikidata.
ESWC(2020)
摘要
Wikidata is a free and open knowledge base which can be read and edited by both humans and machines. It acts as a central storage for the structured data of several Wikimedia projects. To improve the process of manually inserting new facts, the Wikidata platform features an association rule-based tool to recommend additional suitable properties. In this work, we introduce a novel approach to provide such recommendations based on frequentist inference. We introduce a trie-based method that can efficiently learn and represent property set probabilities in RDF graphs. We extend the method by adding type information to improve recommendation precision and introduce backoff strategies which further increase the performance of the initial approach for entities with rare property combinations. We investigate how the captured structure can be employed for property recommendation, analogously to the Wikidata PropertySuggester. We evaluate our approach on the full Wikidata dataset and compare its performance to the state-of-the-art Wikidata PropertySuggester, outperforming it in all evaluated metrics. Notably we could reduce the average rank of the first relevant recommendation by 71%.
更多查看译文
关键词
Wikidata, Recommender systems, Statistical property recommendation, Frequent pattern mining, Knowledge graph editing
AI 理解论文
溯源树
样例
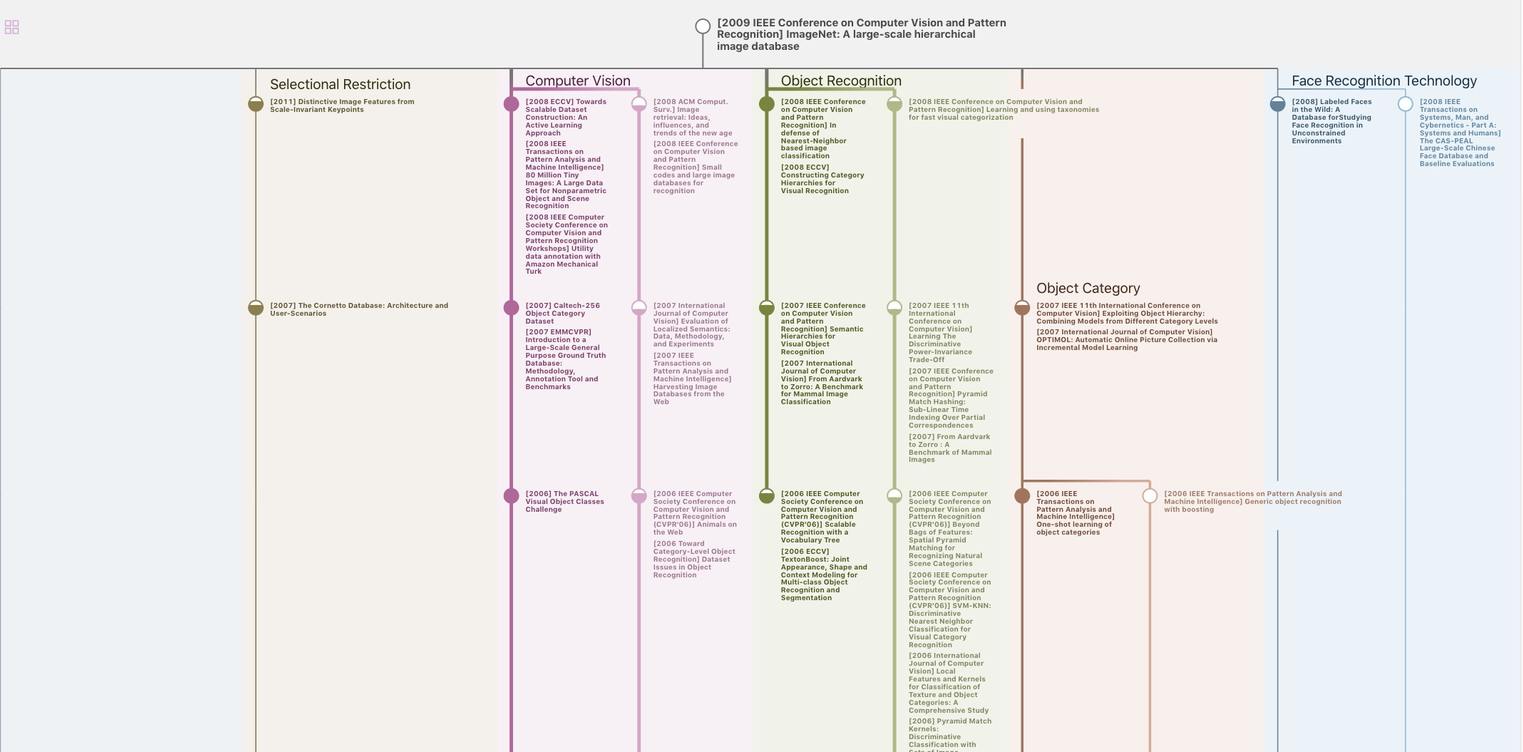
生成溯源树,研究论文发展脉络
Chat Paper
正在生成论文摘要