AI-based Reference Ankle Joint Torque Trajectory Generation for Robotic Gait Assistance: First Steps
2020 IEEE International Conference on Autonomous Robot Systems and Competitions (ICARSC)(2020)
摘要
Robotic-based gait rehabilitation and assistance have been growing to augment and to recover motor function in subjects with lower limb impairments. There is interest in developing user-oriented control strategies to provide personalized assistance. However, it is still needed to set the healthy user-oriented reference joint trajectories, namely, reference ankle joint torque, that would be desired under healthy conditions. Considering the potential of Artificial Intelligence (AI) algorithms to model nonlinear relationships of the walking motion, this study implements and compares two offline AI-based regression models (Multilayer Perceptron and Long-Short Term Memory-LSTM) to generate healthy reference ankle joint torques oriented to subjects with a body height ranging from 1.51 to 1.83 m, body mass from 52.0 to 83.7 kg and walking in a flat surface with a walking speed from 1.0 to 4.0 km/h. The best results were achieved for the LSTM, reaching a Goodness of Fit and a Normalized Root Mean Square Error of 79.6 % and 4.31 %, respectively. The findings showed that the implemented LSTM has the potential to be integrated into control architectures of robotic assistive devices to accurately estimate healthy user-oriented reference ankle joint torque trajectories, which are needed in personalized and Assist-As-Needed conditions. Future challenges involve the exploration of other regression models and the reference torque prediction for remaining lower limb joints, considering a wider range of body masses, heights, walking speeds, and locomotion modes.
更多查看译文
关键词
Ankle Joint Torque Prediction,Artificial Intelligence,Control Strategies,Regression Models,Robotic Gait Rehabilitation
AI 理解论文
溯源树
样例
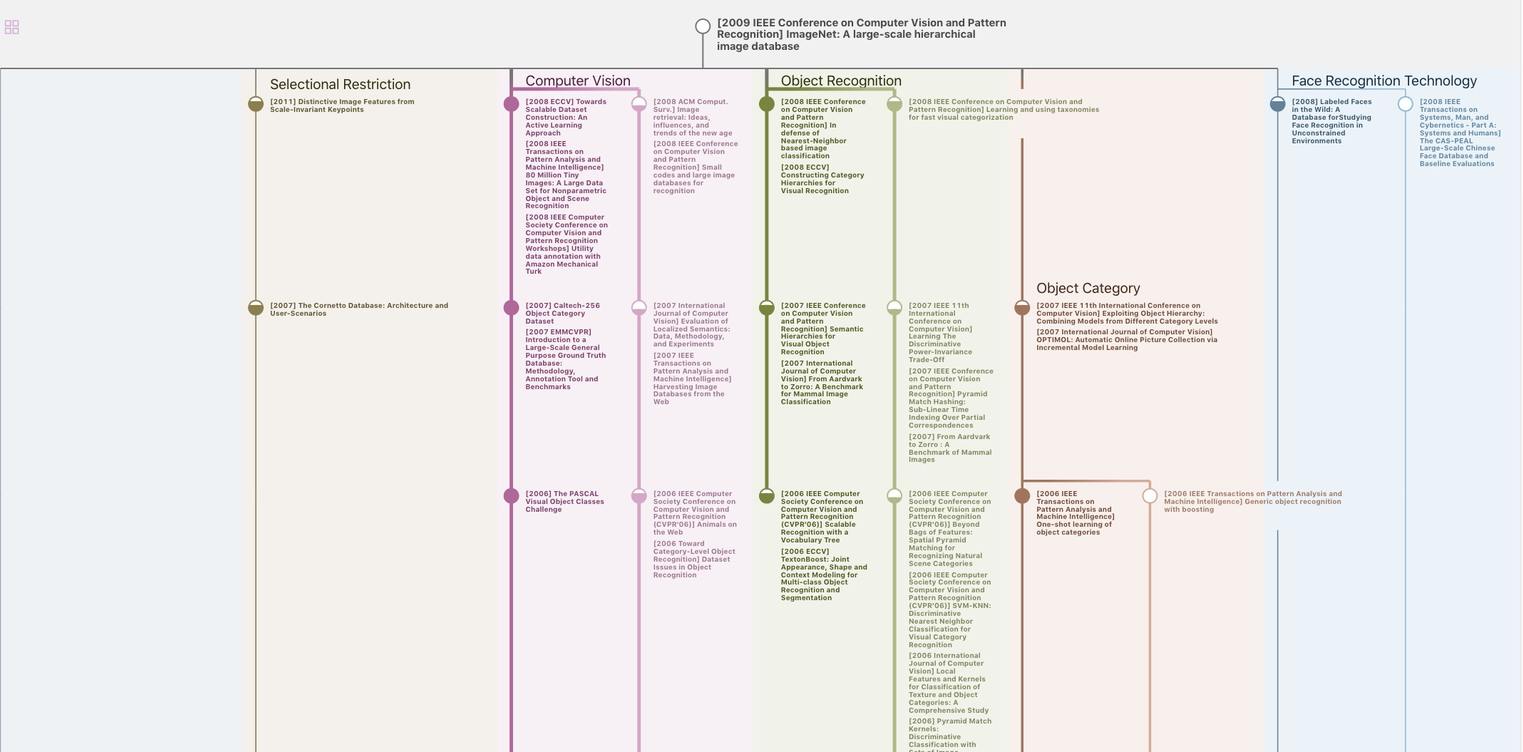
生成溯源树,研究论文发展脉络
Chat Paper
正在生成论文摘要