Disentanglement Then Reconstruction: Learning Compact Features for Unsupervised Domain Adaptation
arxiv(2020)
摘要
Recent works in domain adaptation always learn domain invariant features to mitigate the gap between the source and target domains by adversarial methods. The category information are not sufficiently used which causes the learned domain invariant features are not enough discriminative. We propose a new domain adaptation method based on prototype construction which likes capturing data cluster centers. Specifically, it consists of two parts: disentanglement and reconstruction. First, the domain specific features and domain invariant features are disentangled from the original features. At the same time, the domain prototypes and class prototypes of both domains are estimated. Then, a reconstructor is trained by reconstructing the original features from the disentangled domain invariant features and domain specific features. By this reconstructor, we can construct prototypes for the original features using class prototypes and domain prototypes correspondingly. In the end, the feature extraction network is forced to extract features close to these prototypes. Our contribution lies in the technical use of the reconstructor to obtain the original feature prototypes which helps to learn compact and discriminant features. As far as we know, this idea is proposed for the first time. Experiment results on several public datasets confirm the state-of-the-art performance of our method.
更多查看译文
关键词
unsupervised domain adaptation,compact features,learning
AI 理解论文
溯源树
样例
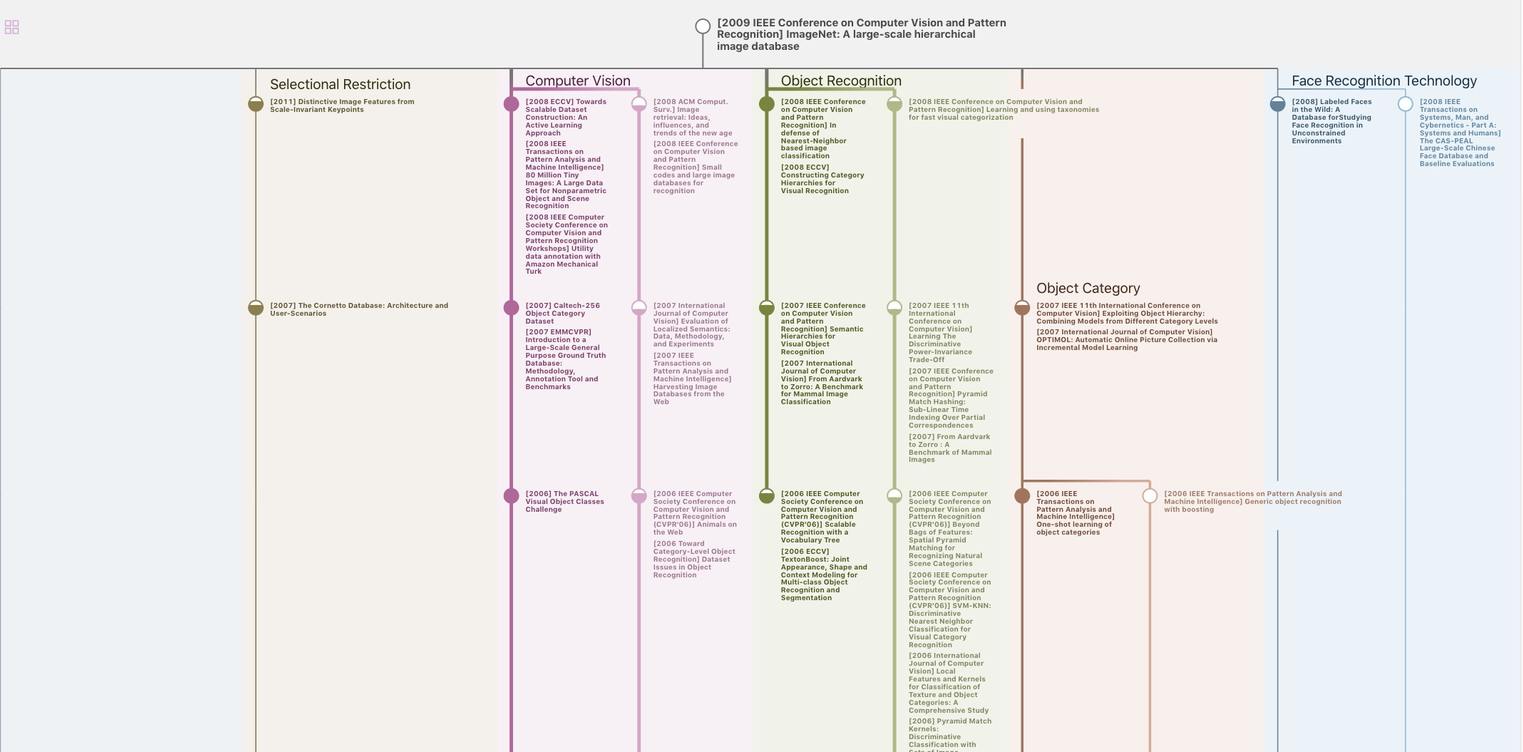
生成溯源树,研究论文发展脉络
Chat Paper
正在生成论文摘要