Robotic Table Tennis with Model-Free Reinforcement Learning
IROS(2020)
摘要
We propose a model-free algorithm for learning efficient policies capable of returning table tennis balls by controlling robot joints at a rate of 100Hz. We demonstrate that evolutionary search (ES) methods acting on CNN-based policy architectures for non-visual inputs and convolving across time learn compact controllers leading to smooth motions. Furthermore, we show that with appropriately tuned curriculum learning on the task and rewards, policies are capable of developing multi-modal styles, specifically forehand and backhand stroke, whilst achieving 80% return rate on a wide range of ball throws. We observe that multi-modality does not require any architectural priors, such as multi-head architectures or hierarchical policies.
更多查看译文
关键词
reinforcement learning,table,model-free
AI 理解论文
溯源树
样例
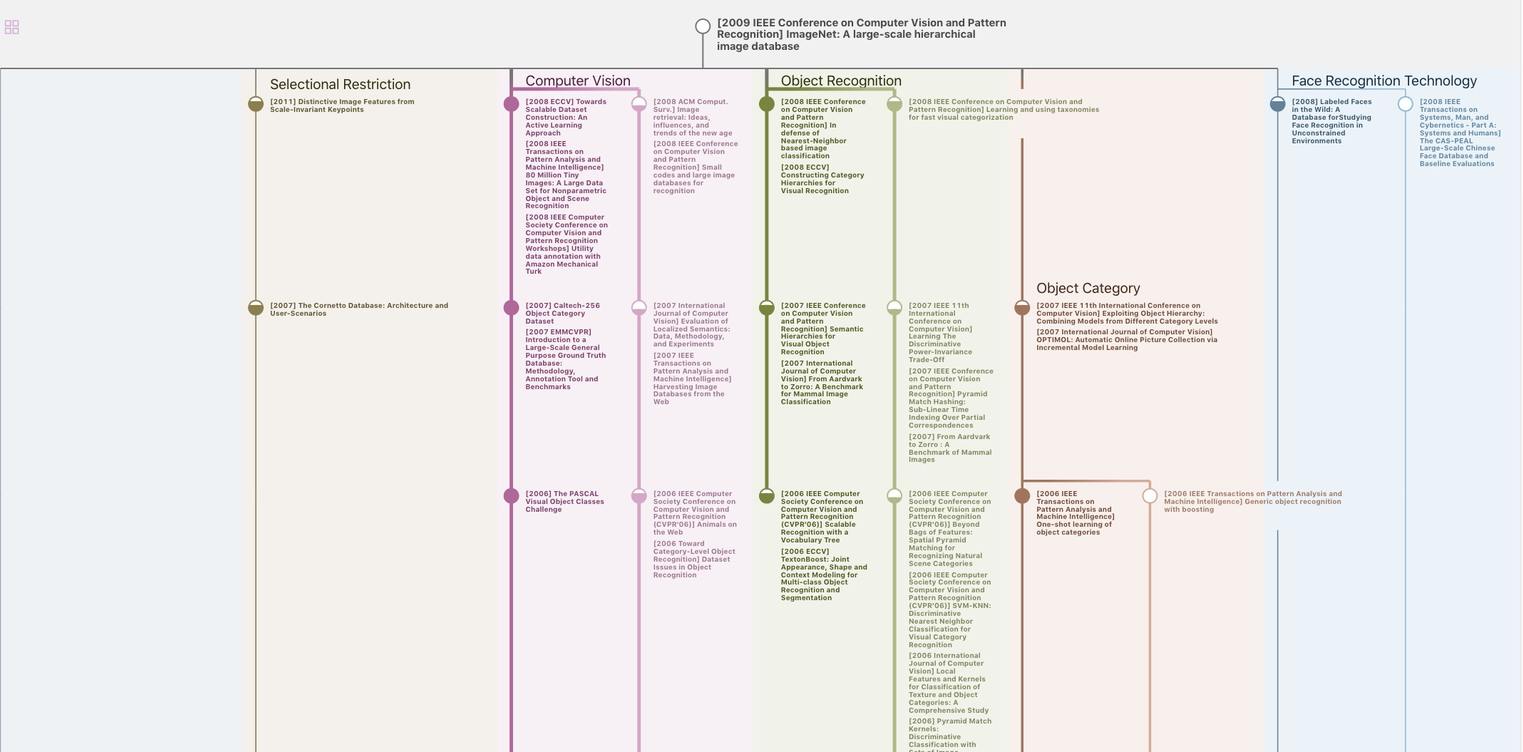
生成溯源树,研究论文发展脉络
Chat Paper
正在生成论文摘要