Modelling Word Associations and Interactiveness for Describer Agents in Word-Guessing Games
29th Benelux Conference on Artificial Intelligence November 8–9, 2017, Groningen(2017)
摘要
In word-guessing games, one player describes a stimulus and his partner should try to guess what the stimulus is. Producing a response to such a stimulus requires an associative mechanism. Additionally, the production of associations that allow the partner to guess what the stimulus is requires modelling a shared context. The Location Taboo Game is such a word-guessing game, in which a describer gives simple textual hints about a target city, and the other player should try to guess this city. The hints given should not contain words from a list of taboo words. In this thesis, an architecture for an artificial describer agent is presented and evaluated through simulation and an empirical study. To be able to elicit a correct guess from a human guesser, the artificial describer agent should mimic associations that humans have with geographical locations. The artificial describer agent extracts word associations from a semantic vector space that has been created with a context-predicting distributional semantic model. Firstly, two methods for extracting word associations are detailed: a nearest neighbours approach that uses the list of taboo words, and an approach that applies clustering and analogical reasoning on a dataset of games played by humans. Secondly, interactiveness is modelled through a rule-based approach for the generation of clues that depend upon the guesser’s response. Different variants of the methods for clue generation are evaluated through simulation and an empirical study. These describer agents could elicit a correct guess for 37.86% of the games and for 50.00% of the games for which the human player knew the target city.
更多查看译文
AI 理解论文
溯源树
样例
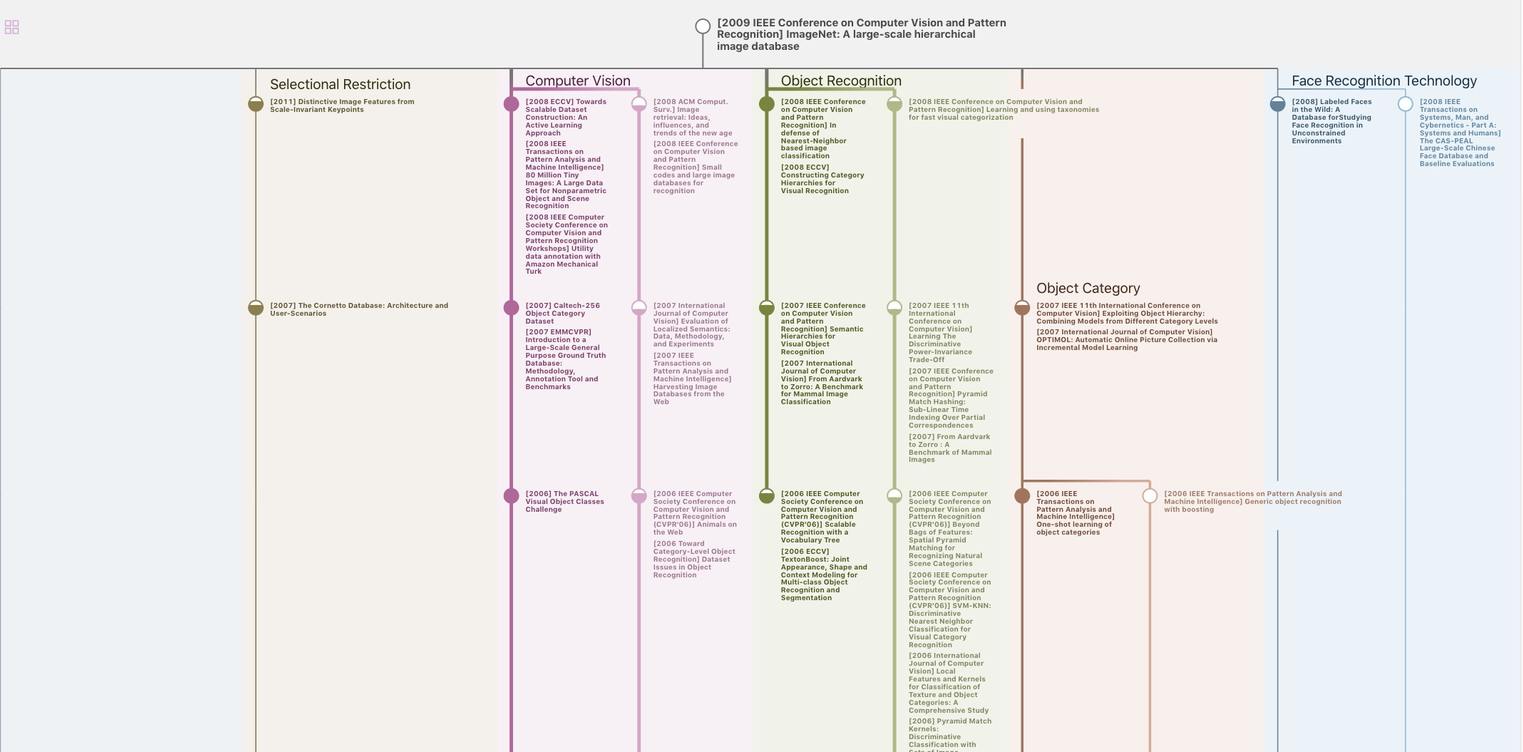
生成溯源树,研究论文发展脉络
Chat Paper
正在生成论文摘要