Vector Based and Neural Models
Human Language: From Genes and Brains to Behavior(2019)
摘要
There is a rich tradition in modeling the meanings of words, phrases, sentences, and discourse, going back, in some cases, thousands of years. For most of that long history, the dominant approach has been an approach based on some form of symbolic logic. This approach has been very successful, in many respects, but it has proven difficult to directly relate such models of semantics to research in (experimental) psychology and cognitive neuroscience. In this chapter, we discuss an alternative approach to modeling meaning based on numerical vectors, using tools from some other branches of mathematics: linear algebra and differential calculus. These models are very attractive from the neural perspective, because numerical vectors are the natural representation for patterns of neural activity, as well as from the learning perspective, because such vectors are compatible with artificial neural network models, and, if certain conditions are met, rich sets of tools for optimization (learning) developed in this paradigm become available. We will get back to these attractive properties in section 1.4.But are numerical vectors not too weak a representation to account for the intricacies of natural language semantics? Hasn’t the rich tradition in lexical and compositional semantics revealed the need to have nontrivial, structured, symbolic models? The goal of this chapter is to show that we can have our cake and eat it: we can use vectorial representations that are compatible with neuroscience and that are optimized using techniques from the neural network toolbox, while still being able to account for nontrivial semantic phenomena. Vectorspace models …
更多查看译文
AI 理解论文
溯源树
样例
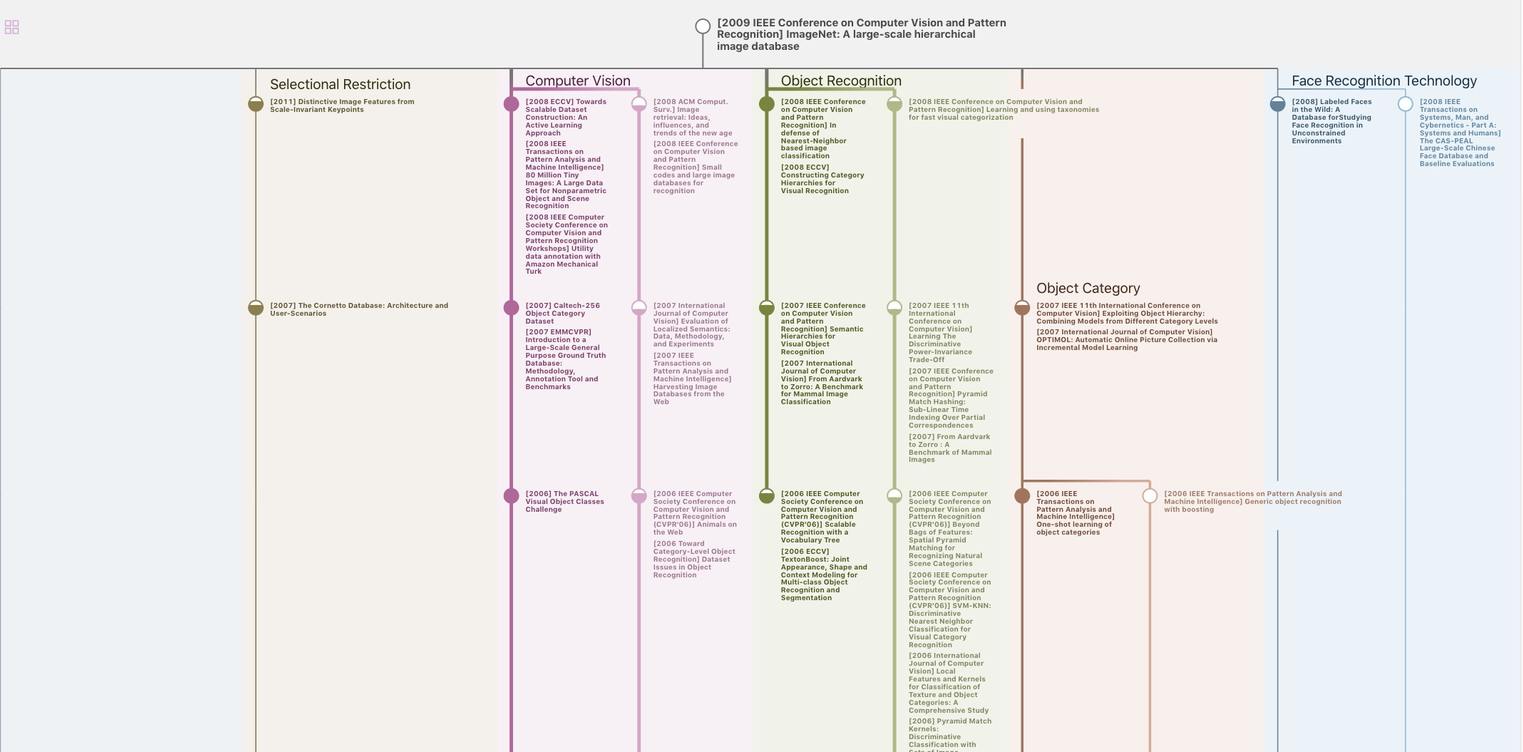
生成溯源树,研究论文发展脉络
Chat Paper
正在生成论文摘要