End-to-End Hierarchical Text Classification with Label Assignment Policy
EMNLP 2019(2018)
摘要
We present an end-to-end reinforcement learning approach to hierarchical text classification where documents are labeled by placing them at the right positions in a given hierarchy. While existing “global” methods construct hierarchical losses for model training, they either make “local” decisions at each hierarchy node or ignore the hierarchy structure during inference. To close the gap between training/inference and optimize holistic metrics in an end-to-end manner, we propose to learn a label assignment policy to determine where to place the documents and when to stop. The proposed method, HiLAP, optimizes holistic metrics over the hierarchy, makes inter-dependent decisions during inference, and can be combined with different text encoding models for end-to-end training. Experiments on three public datasets show that HiLAP yields an average improvement of 33.4% in Macro-F1 and 5.0% in Samples-F1, outperforming state-of-the-art methods by a large margin.
更多查看译文
AI 理解论文
溯源树
样例
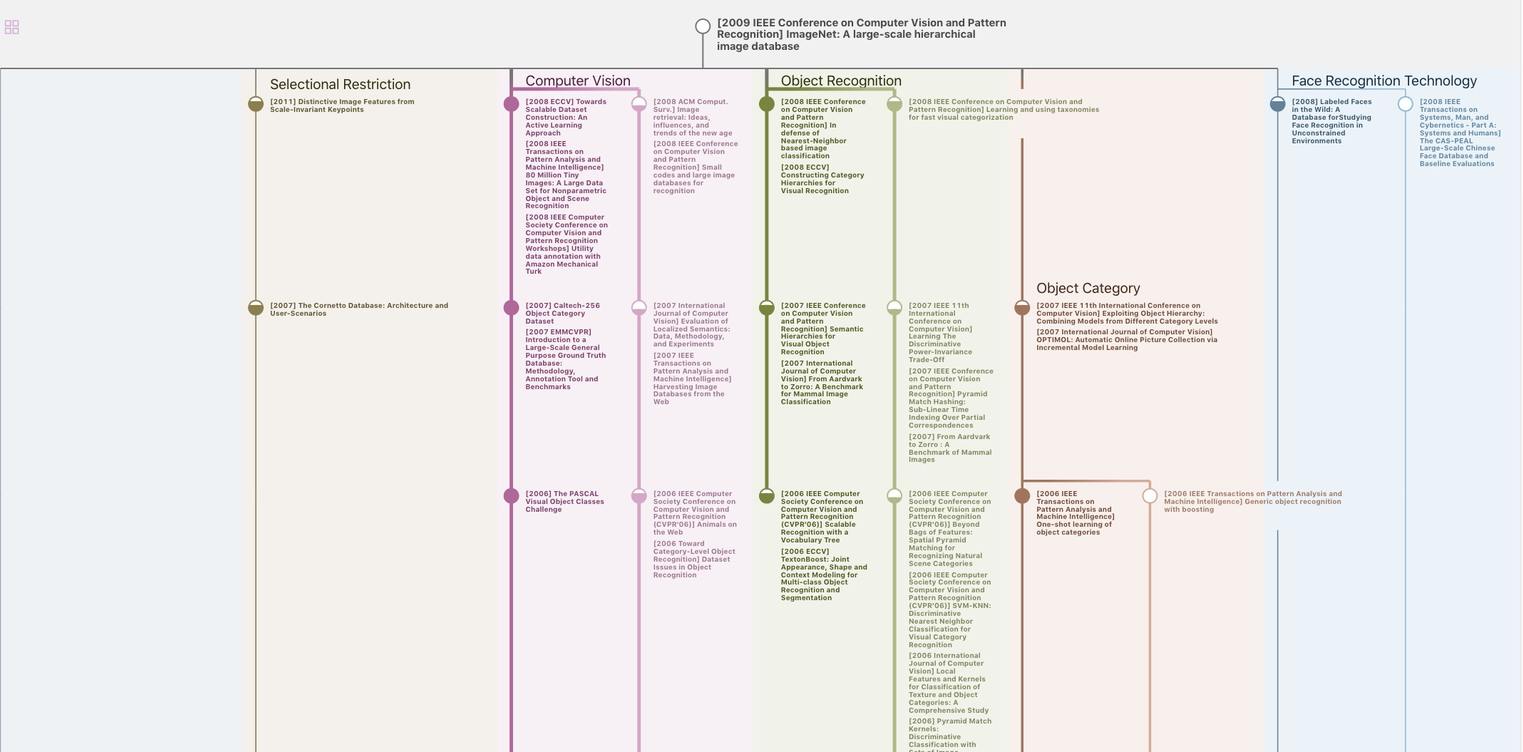
生成溯源树,研究论文发展脉络
Chat Paper
正在生成论文摘要