Entity Typing: A Critical Step for Mining Structures from Massive Unstructured Text
Proc. KDD2016 Workshop on Mining and Learning with Graphs(2016)
摘要
We have been studying learning and mining graphs or networks. However, where do most real networks come from? Although some networks come from well-structured and explicitly connected nodes and links, a majority of networks come from massive unstructured text data, and it takes human efforts to extract them and build them explicitly. Unfortunately, manual data curation and extraction of structures from unstructured data can be costly, unscalable, and error-prone. We have been investigating a data-driven approach to building structured networks from unstructured text data. First, quality phrases can be mined from massive text corpus, serving as basic semantic units, mostly being entities. Second, types can be inferred for such entities from such massive text data with distant supervision and relationships among entities can be uncovered by network embedding as well. Therefore, entity typing is a critical step for mining structures from unstructured text data. In this study, we focus on how to conduct entity typing with a data-driven approach. We show that “rough” entity types can be identified from massive text data with a distant supervision approach via some domain-independent knowledge-bases. However, for refined typing, even the type labels in a knowledge bases can be noisy (ie, incorrect for the entity mention’s local context). We propose a general framework, called PLE, to jointly embed entity mentions, text features and entity types into the same low-dimensional space where, in that space, objects whose types are semantically close have similar representations. Then we estimate the type-path for each training example in a top …
更多查看译文
AI 理解论文
溯源树
样例
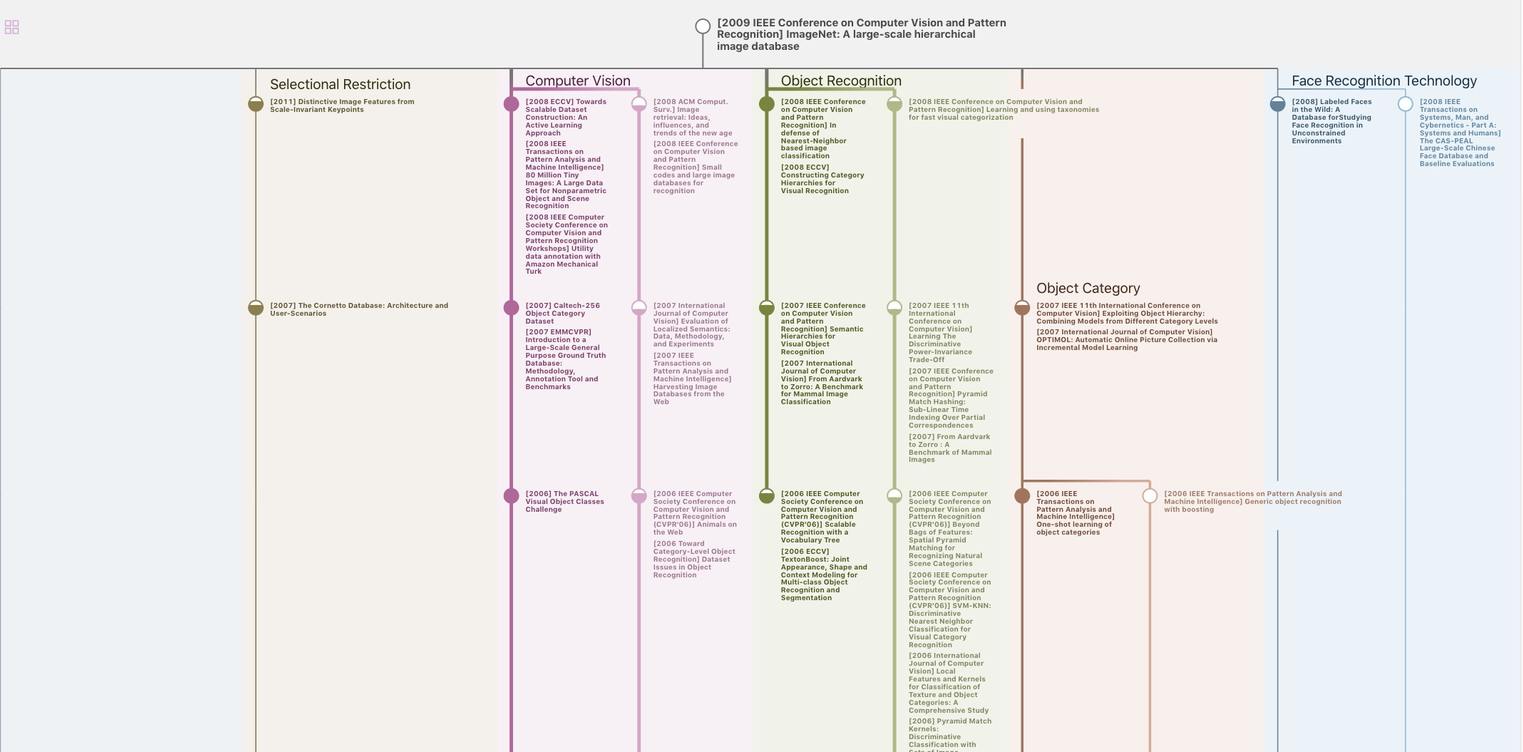
生成溯源树,研究论文发展脉络
Chat Paper
正在生成论文摘要