Learning Neural Knowledge Representations
user-5ebe282a4c775eda72abcdce(2019)
摘要
Much of the collective human knowledge resides on the internet today. Half the world’s population has access to internet, and consequently this knowledge, but none can navigate this wealth of information without the help of technology. Knowledge representation refers to organizing this information in a form such that any piece of it can be easily retrieved when a user asks for it. is involves processing extremely large-scale data, and, at the same time, resolving ne-grained ambiguities inherent in natural language. Further di culties are presented by the heterogeneous mix of structured and unstructured data typically available on the web, and the expensive cost of annotating such representations. is thesis aims to develop e cient, scalable and exible knowledge representations by leveraging recent successes in deep learning. We train neural networks to represent diverse sources of knowledge including unstructured text, linguistic annotations, and curated databases, by answering queries posed over them. To increase the e ciency of learning, we discuss inductive biases for adapting recurrent neural networks to represent text, and graph convolution networks to represent structured data. We also present a semi-supervised technique which exploits unlabeled text documents in addition to labeled question and answer pairs for learning. In the last part of the thesis we propose a distributed text knowledge base for representing very large text corpora, such as the entire Wikipedia. Towards this end, we present preliminary results investigating the applicability of contextual word representation models for indexing large corpora, as well as ne-tuning …
更多查看译文
AI 理解论文
溯源树
样例
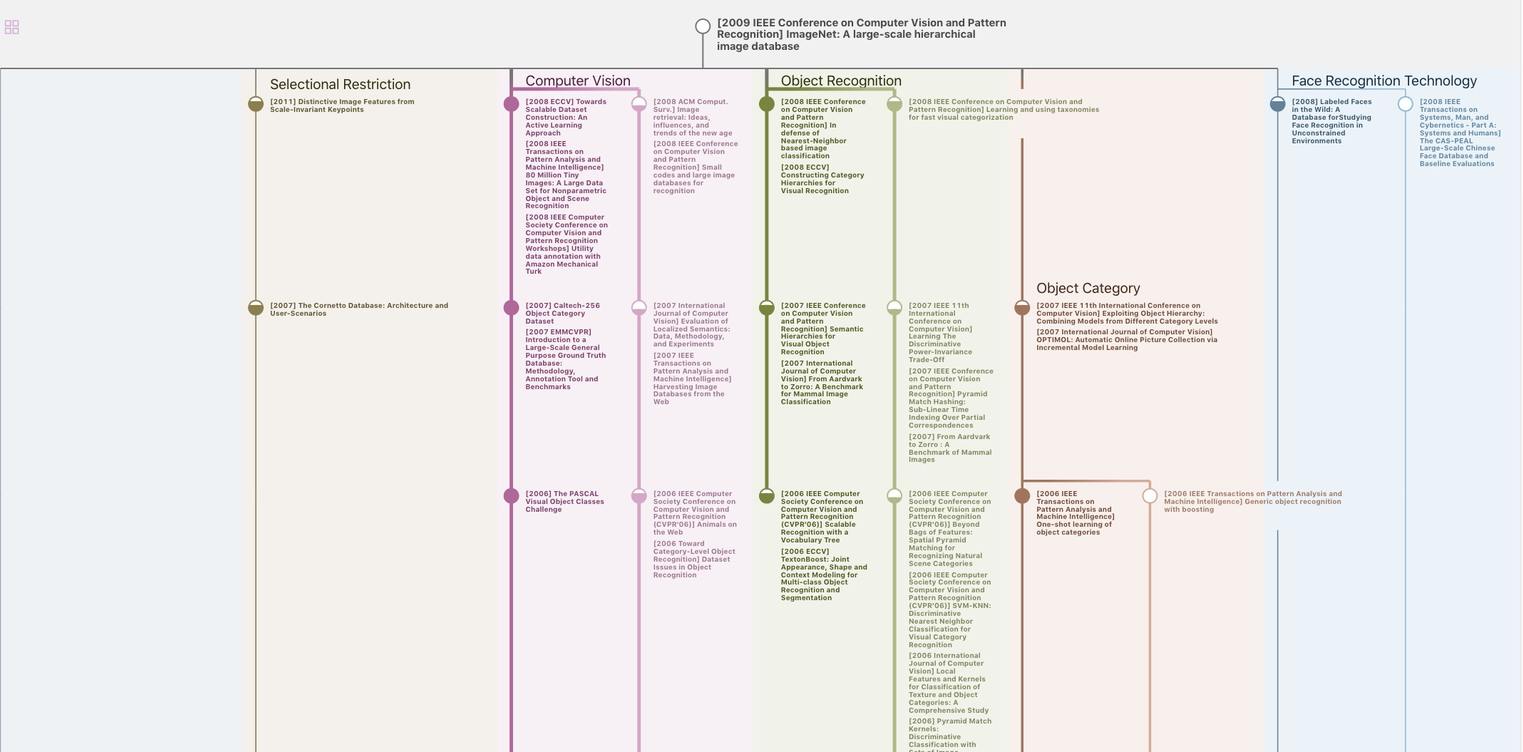
生成溯源树,研究论文发展脉络
Chat Paper
正在生成论文摘要