Training neural networks for aspect extraction using descriptive keywords only
user-5ebe28444c775eda72abcdcf(2019)
摘要
Aspect extraction in online product reviews is a key task in sentiment analysis and opinion mining. Training supervised neural networks for aspect extraction is not possible when ground truth aspect labels are not available, while the unsupervised neural topic models fail to capture the particular aspects of interest. In this work, we propose a weakly supervised approach for training neural networks for aspect extraction in cases where only a small set of seed words, ie, keywords that describe an aspect, are available. Our main contributions are as follows. First, we show that current weakly supervised networks fail to leverage the predictive power of the available seed words by comparing them to a simple bag-of-words classifier. Second, we propose a distillation approach for aspect extraction where the seed words are considered by the bag-of-words classifier (teacher) and distilled to the parameters of a neural network (student). Third, we show that regularization encourages the student to consider non-seed words for classification and, as a result, the student outperforms the teacher, which only considers the seed words. Finally, we empirically show that our proposed distillation approach outperforms (by up to 34.4% in F1 score) previous weakly supervised approaches for aspect extraction in six domains of Amazon product reviews.
更多查看译文
AI 理解论文
溯源树
样例
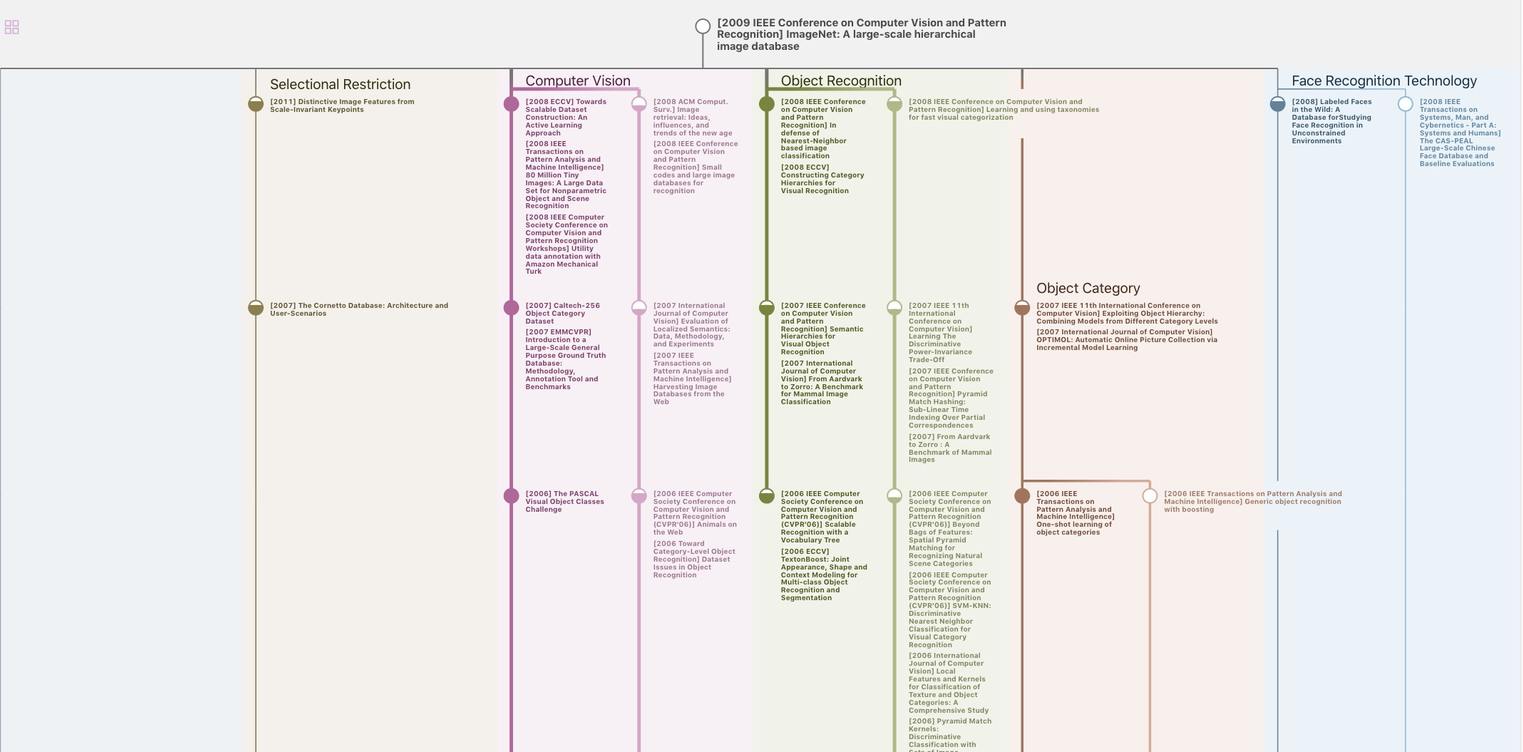
生成溯源树,研究论文发展脉络
Chat Paper
正在生成论文摘要