Using the Literature to Construct Causal Models for Pharmacovigilance
user-5ebe282a4c775eda72abcdce(2018)
摘要
Causal discovery methods provide a means to ascertain causal attribution from observational data. Causal modeling at scale requires a method to populate models with relevant domain knowledge. We propose to use the biomedical literature to perform feature selection for drug/adverse drug event (ADE) models with clinical observational data derived from electronic health records (EHR) as our primary input data source. We reason that spurious (non-causal) drug-ADE associations from co-occurrence-based analyses should diminish conditional on sets of validated confounders identified in the literature. To evaluate this hypothesis, we used a publicly available reference data set to test the proposed methodology with 4 ADEs and 399 drug-ADE pairs. We calculated baseline scores using the rank order regression coefficients each drug-ADE pair. We then identified confounding variable candidates for each drug-ADE pair using relationship constraints based on normalized predicates to search knowledge extracted from the literature in the publicly available SemMedDB repository. To determine eligibility for inclusion, we checked whether or not there were directed edges pointing to both the drug and the ADE. Finally, we tested whether associations from co-occurrence in the clinical data are diminished conditional on sets of permutations of confounders identified in the literature. Confounder yield rate was~ 90%, indicating that our method successfully identified confounders in the observational data. Causal models attained aggregate performance improvements of~ 0.07 area under the curve and reduced the False Discovery Rate from 0.50 to 0 …
更多查看译文
AI 理解论文
溯源树
样例
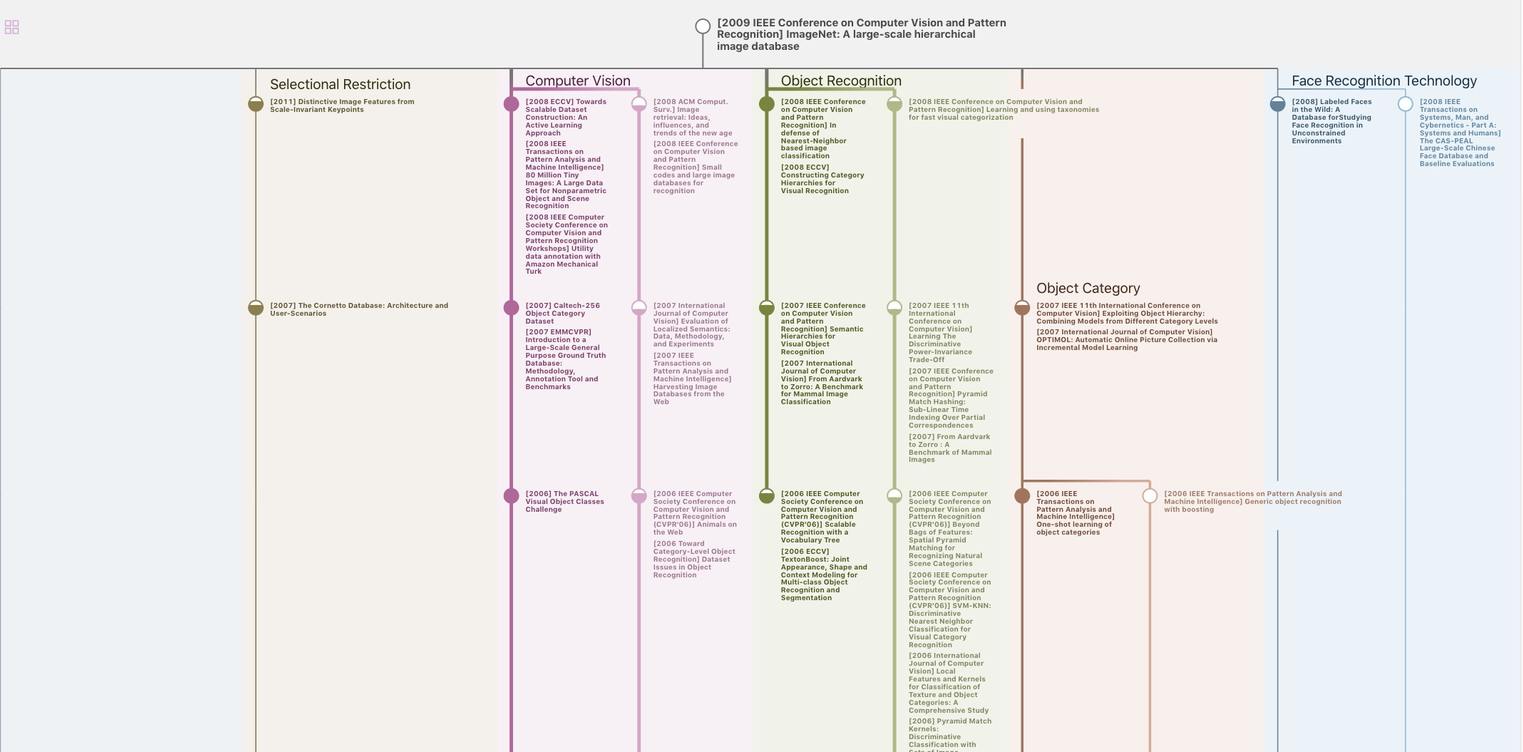
生成溯源树,研究论文发展脉络
Chat Paper
正在生成论文摘要