GreenSea: Visual Soccer Analysis Using Broad Learning System
IEEE Transactions on Cybernetics(2021)
摘要
Modern soccer increasingly places trust in visual analysis and statistics rather than only relying on the human experience. However, soccer is an extraordinarily complex game that no widely accepted quantitative analysis methods exist. The statistics collection and visualization are time consuming which result in numerous adjustments. To tackle this issue, we developed GreenSea, a visual-based assessment system designed for soccer game analysis, tactics, and training. The system uses a broad learning system (BLS) to train the model in order to avoid the time-consuming issue that traditional deep learning may suffer. Users are able to apply multiple views of a soccer game, and visual summarization of essential statistics using advanced visualization and animation that are available. A marking system trained by BLS is designed to perform quantitative analysis. A novel recurrent discriminative BLS (RDBLS) is proposed to carry out long-term tracking. In our RDBLS, the structure is adjusted to have better performance on the binary classification problem of the discriminative model. Several experiments are carried out to verify that our proposed RDBLS model can outperform the standard BLS and other methods. Two studies were conducted to verify the effectiveness of our GreenSea. The first study was on how GreenSea assists a youth training coach to assess each trainee's performance for selecting most potential players. The second study was on how GreenSea was used to help the U20 Shanghai soccer team coaching staff analyze games and make tactics during the 13th National Games. Our studies have shown the usability of GreenSea and the values of our system to both amateur and expert users.
更多查看译文
关键词
Algorithms,Deep Learning,Humans,Image Processing, Computer-Assisted,Models, Statistical,Soccer,Video Recording
AI 理解论文
溯源树
样例
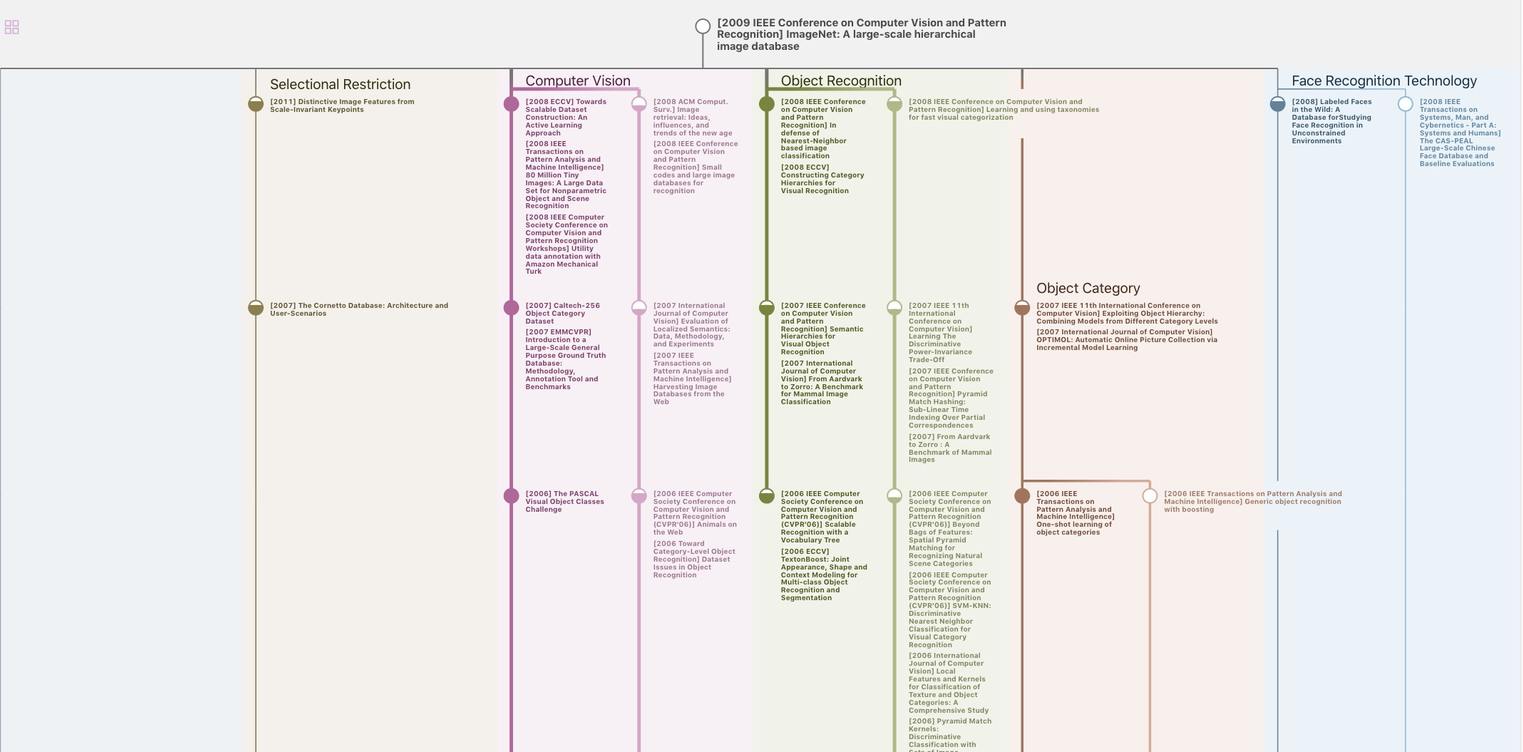
生成溯源树,研究论文发展脉络
Chat Paper
正在生成论文摘要