InfantNet: A Deep Neural Network for Analyzing Infant Vocalizations
arxiv(2020)
摘要
Acoustic analyses of infant vocalizations are valuable for research on speech development as well as applications in sound classification. Previous studies have focused on measures of acoustic features based on theories of speech processing, such spectral and cepstrum-based analyses. More recently, end-to-end models of deep learning have been developed to take raw speech signals (acoustic waveforms) as inputs and convolutional neural network layers to learn representations of speech sounds based on classification tasks. We applied a recent end-to-end model of sound classification to analyze a large-scale database of labeled infant and adult vocalizations recorded in natural settings outside the lab with no control over recording conditions. The model learned basic classifications like infant versus adult vocalizations, infant speech-related versus non-speech vocalizations, and canonical versus non-canonical babbling. The model was trained on recordings of infants ranging from 3 to 18 months of age, and classification accuracy changed with age as speech became more distinct and babbling became more speech-like. Further work is needed to validate and explore the model and dataset, but our results show how deep learning can be used to measure and investigate speech acquisition and development, with potential applications in speech pathology and infant monitoring.
更多查看译文
AI 理解论文
溯源树
样例
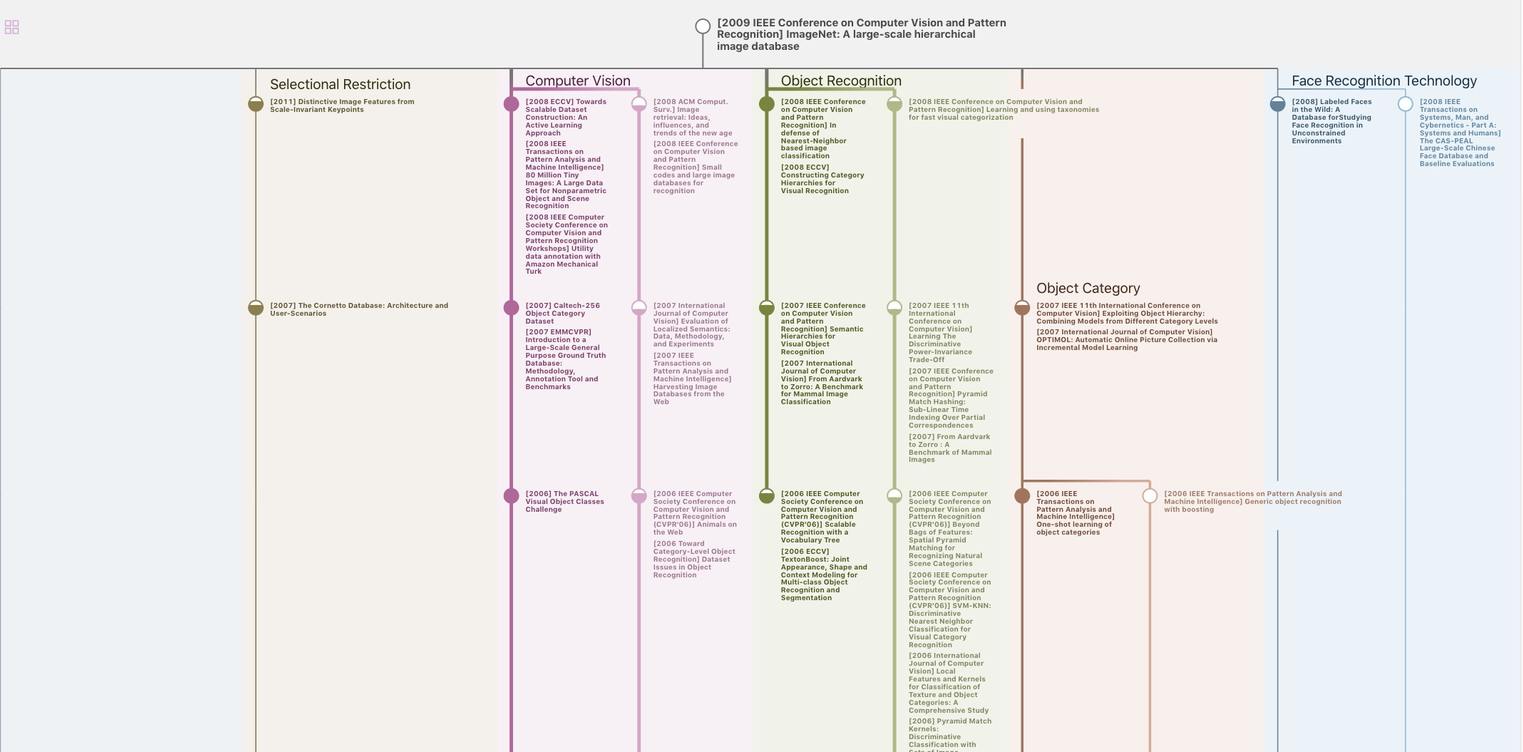
生成溯源树,研究论文发展脉络
Chat Paper
正在生成论文摘要