Interlayer and intralayer scale aggregation for scale-invariant crowd counting
Neurocomputing(2021)
摘要
Crowd counting is an important vision task, which faces challenges on continuous scale variation within a given scene and huge density shift both within and across images. These challenges are typically addressed using multi-column structures in existing methods. However, such an approach does not provide consistent improvement and transferability due to limited ability in capturing multi-scale features, sensitivity to large density shift, and difficulty in training multi-branch models. To overcome these limitations, a Single-column Scalere-invariant Network (ScSiNet) is presented in this paper, which extracts sophisticated scale-invariant features via the combination of interlayer multi-scale integration and a novel intralayer scale-invariant transformation (SiT). Furthermore, in order to enlarge the diversity of densities, a randomly integrated loss is presented for training our single-branch method. Extensive experiments on public datasets demonstrate that the proposed method outperforms state-of-the-art approaches in counting accuracy and achieves remarkable transferability and scale-invariant property.
更多查看译文
关键词
Scale Invariance,Stochastic scale mixer,Transferability,Randomly integrated loss,Crowd counting
AI 理解论文
溯源树
样例
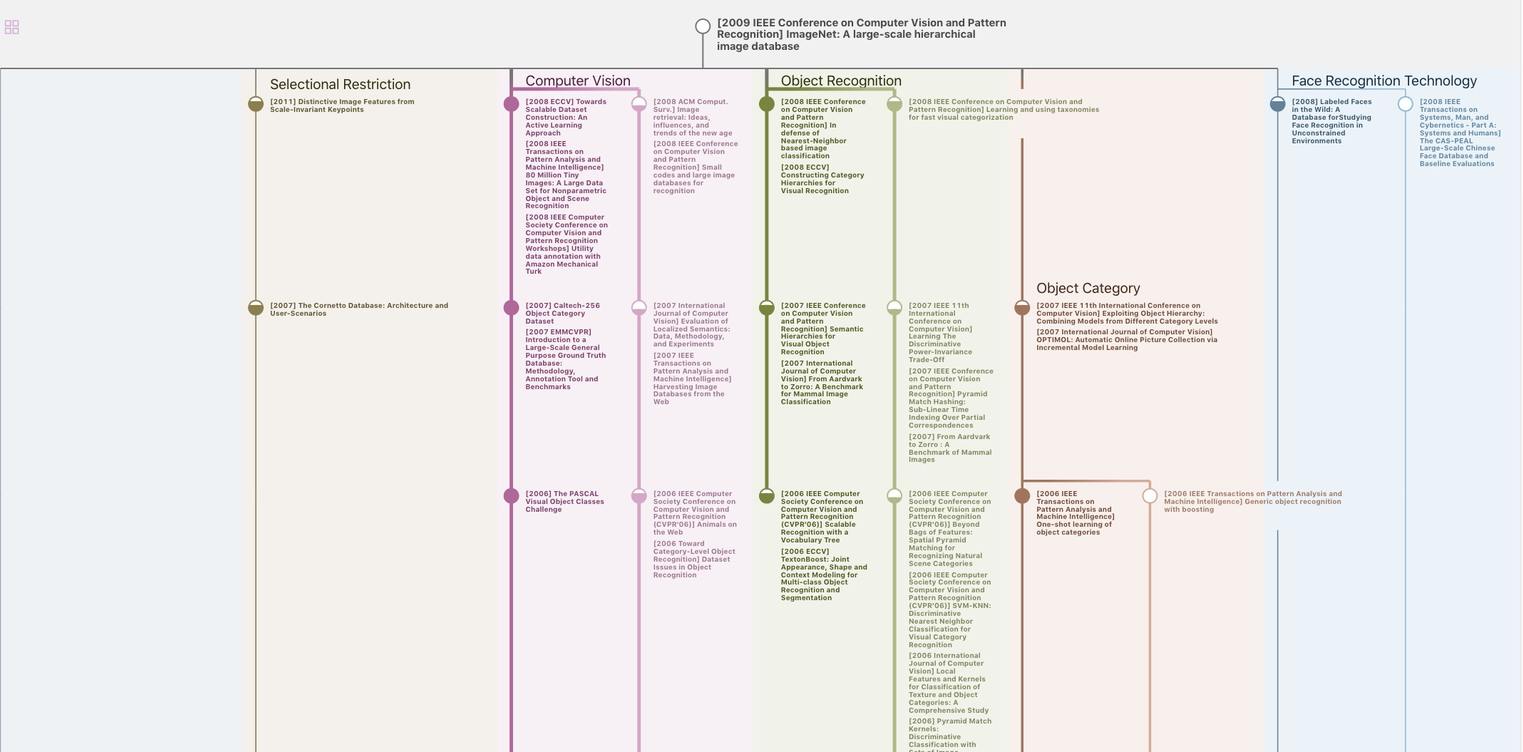
生成溯源树,研究论文发展脉络
Chat Paper
正在生成论文摘要