High-Resolution Image Inpainting with Iterative Confidence Feedback and Guided Upsampling
European Conference on Computer Vision(2020)
摘要
Existing image inpainting methods often produce artifacts when dealing with large holes in real applications. To address this challenge, we propose an iterative inpainting method with a feedback mechanism. Specifically, we introduce a deep generative model which not only outputs an inpainting result but also a corresponding confidence map. Using this map as feedback, it progressively fills the hole by trusting only high-confidence pixels inside the hole at each iteration and focuses on the remaining pixels in the next iteration. As it reuses partial predictions from the previous iterations as known pixels, this process gradually improves the result. In addition, we propose a guided upsampling network to enable generation of high-resolution inpainting results. We achieve this by extending the Contextual Attention module [39] to borrow high-resolution feature patches in the input image. Furthermore, to mimic real object removal scenarios, we collect a large object mask dataset and synthesize more realistic training data that better simulates user inputs. Experiments show that our method significantly outperforms existing methods in both quantitative and qualitative evaluations. More results and Web APP are available at https://zengxianyu.github.io/iic.
更多查看译文
关键词
high-resolution high-resolution,iterative confidence feedback
AI 理解论文
溯源树
样例
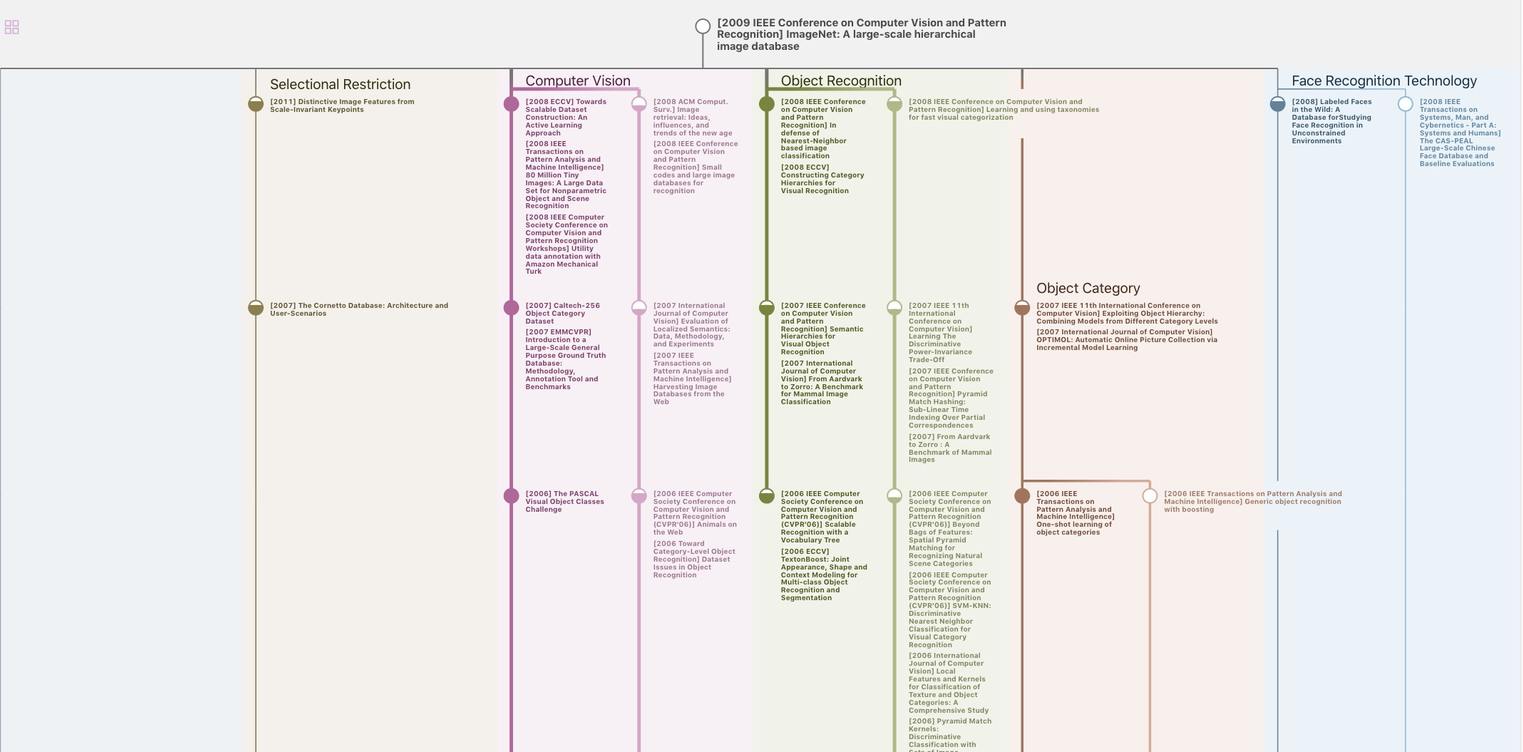
生成溯源树,研究论文发展脉络
Chat Paper
正在生成论文摘要