SharinGAN: Combining Synthetic and Real Data for Unsupervised Geometry Estimation
CVPR(2020)
摘要
We propose a novel method for combining synthetic and real images when training networks to determine geometric information from a single image. We suggest a method for mapping both image types into a single, shared domain. This is connected to a primary network for end-to-end training. Ideally, this results in images from two domains that present shared information to the primary network. Our experiments demonstrate significant improvements over the state-of-the-art in two important domains, surface normal estimation of human faces and monocular depth estimation for outdoor scenes, both in an unsupervised setting.
更多查看译文
关键词
unsupervised geometry estimation,real data
AI 理解论文
溯源树
样例
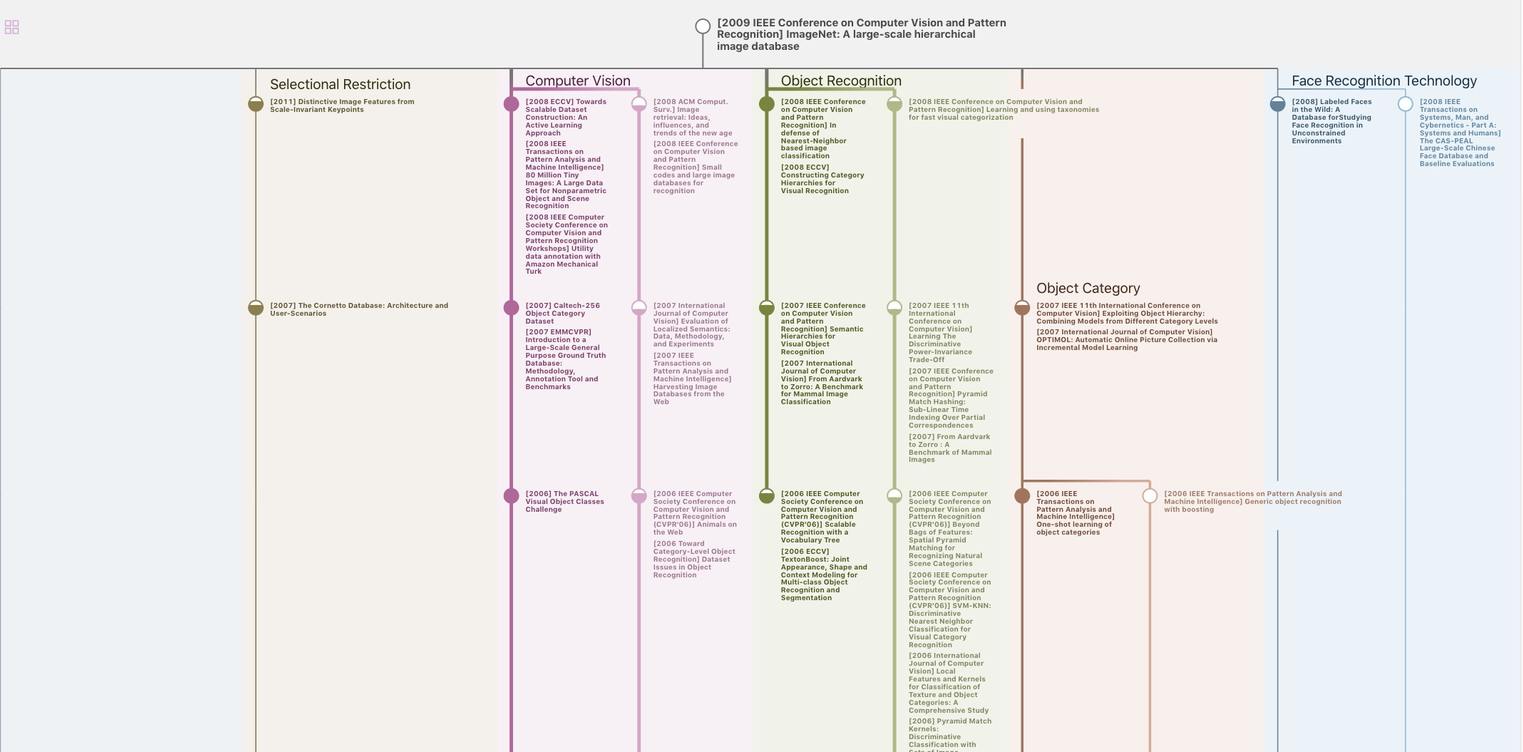
生成溯源树,研究论文发展脉络
Chat Paper
正在生成论文摘要