SLV: Spatial Likelihood Voting for Weakly Supervised Object Detection
CVPR(2020)
摘要
Based on the framework of multiple instance learning (MIL), tremendous works have promoted the advances of weakly supervised object detection (WSOD). However, most MIL-based methods tend to localize instances to their discriminative parts instead of the whole content. In this paper, we propose a spatial likelihood voting (SLV) module to converge the proposal localizing process without any bounding box annotations. Specifically, all region proposals in a given image play the role of voters every iteration during training, voting for the likelihood of each category in spatial dimensions. After dilating alignment on the area with large likelihood values, the voting results are regularized as bounding boxes, being used for the final classification and localization. Based on SLV, we further propose an end-to-end training framework for multi-task learning. The classification and localization tasks promote each other, which further improves the detection performance. Extensive experiments on the PASCAL VOC 2007 and 2012 datasets demonstrate the superior performance of SLV.
更多查看译文
关键词
multitask learning,localization tasks,SLV,weakly supervised object detection,multiple instance learning,MIL,spatial likelihood voting module,proposal localizing process,spatial dimensions,likelihood values,bounding boxes,image classification
AI 理解论文
溯源树
样例
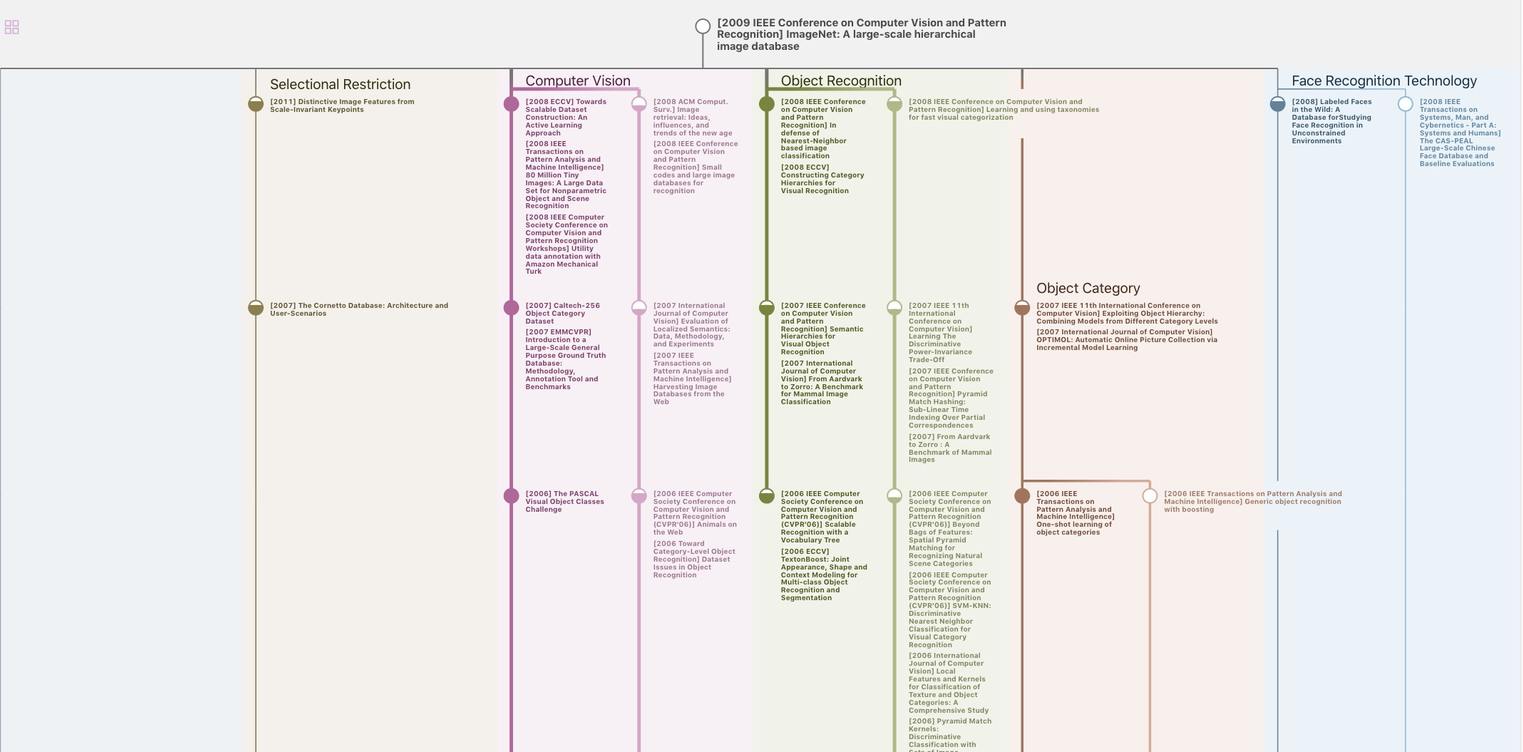
生成溯源树,研究论文发展脉络
Chat Paper
正在生成论文摘要