Stochastic Classifiers for Unsupervised Domain Adaptation
CVPR(2020)
摘要
A common strategy adopted by existing state-of-the-art unsupervised domain adaptation (UDA) methods is to employ two classifiers to identify the misaligned local regions between source and target domain. Following the \u0027wisdom of the crowd\u0027 principle, one has to ask: why stop at two? Indeed, we find that using more classifiers leads to better performance, but also introduces more model parameters, therefore risking overfitting. In this paper, we introduce a novel method called STochastic clAssifieRs (STAR) for addressing this problem. Instead of representing one classifier as a weight vector, STAR models it as a Gaussian distribution with its variance representing the inter-classifier discrepancy. With STAR, we can now sample an arbitrary number of classifiers from the distribution, whilst keeping the model size the same as having two classifiers. Extensive experiments demonstrate that a variety of existing UDA methods can greatly benefit from STAR and achieve the state-of-the-art performance on both image classification and semantic segmentation tasks.
更多查看译文
关键词
stochastic classifiers,unsupervised domain adaptation methods,misaligned local regions,target domain,STAR models,UDA methods,weight vector,image classification,semantic segmentation task
AI 理解论文
溯源树
样例
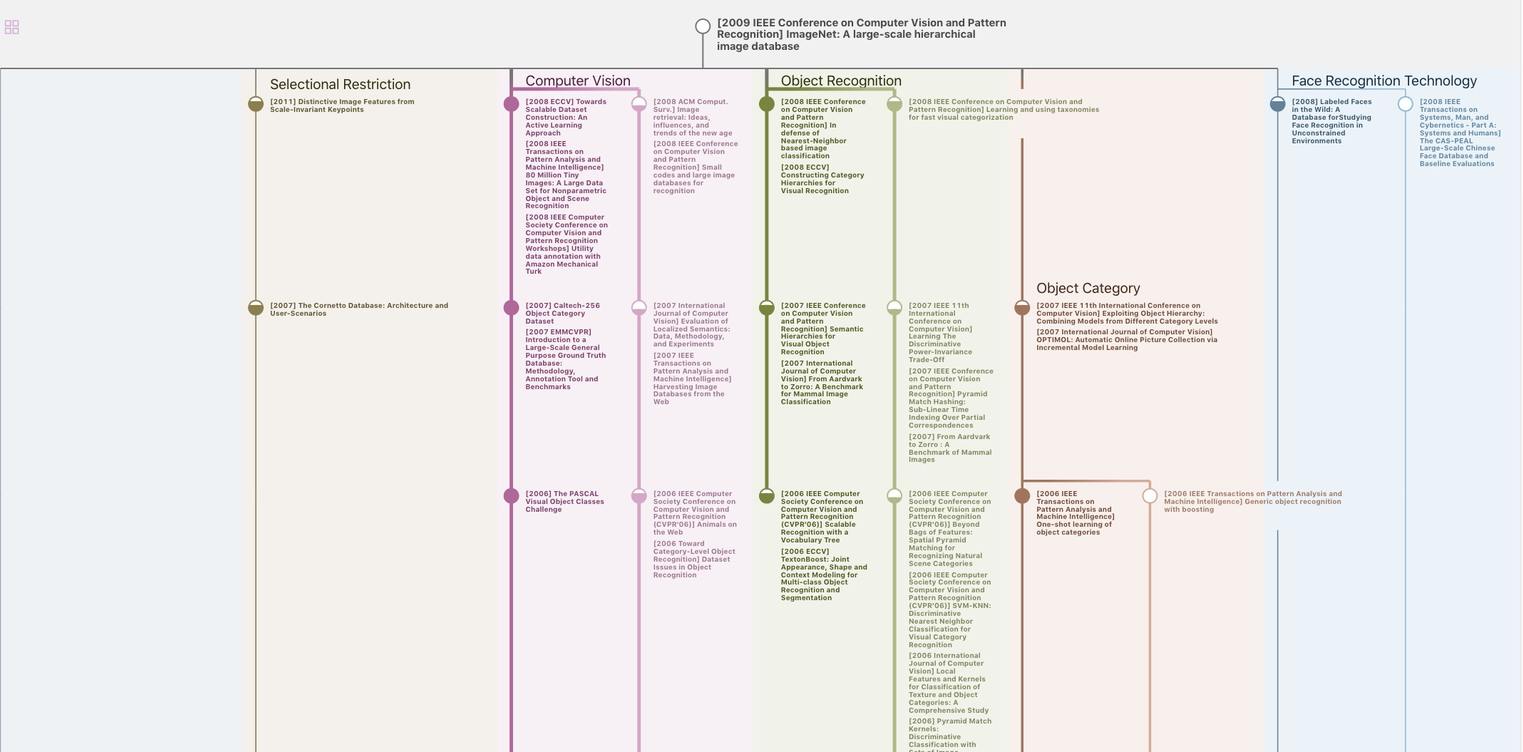
生成溯源树,研究论文发展脉络
Chat Paper
正在生成论文摘要