Spherical Space Domain Adaptation With Robust Pseudo-Label Loss
CVPR(2020)
摘要
Adversarial domain adaptation (DA) has been an effective approach for learning domain-invariant features by adversarial training. In this paper, we propose a novel adversarial DA approach completely defined in spherical feature space, in which we define spherical classifier for label prediction and spherical domain discriminator for discriminating domain labels. To utilize pseudo-label robustly, we develop a robust pseudo-label loss in the spherical feature space, which weights the importance of estimated labels of target data by posterior probability of correct labeling, modeled by Gaussian-uniform mixture model in spherical feature space. Extensive experiments show that our method achieves state-of-the-art results, and also confirm effectiveness of spherical classifier, spherical discriminator and spherical robust pseudo-label loss.
更多查看译文
关键词
spherical space domain adaptation,adversarial domain adaptation,domain-invariant features,adversarial training,spherical feature space,spherical classifier,label prediction,spherical domain discriminator,domain labels,spherical robust pseudolabel loss,adversarial DA approach,Gaussian-uniform mixture model,probability
AI 理解论文
溯源树
样例
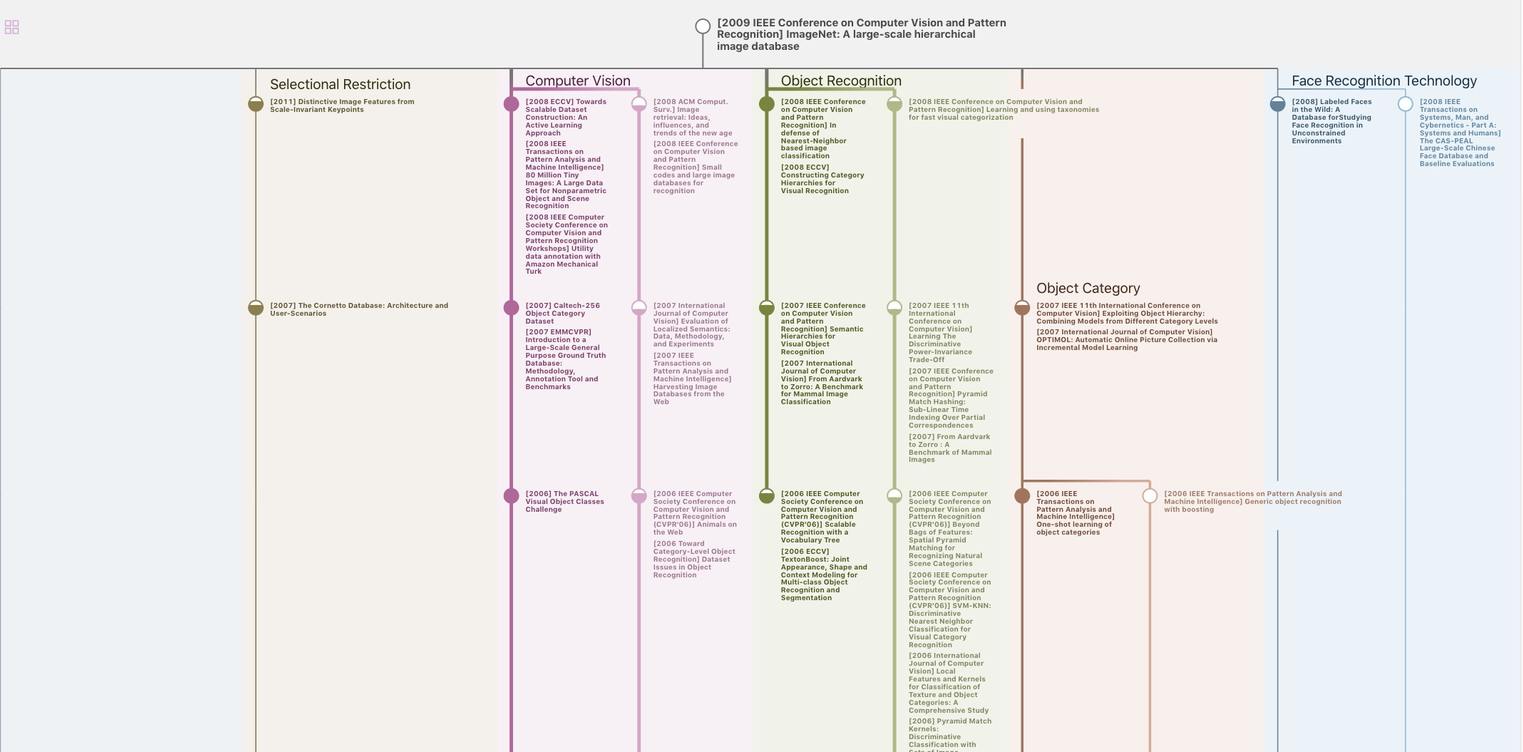
生成溯源树,研究论文发展脉络
Chat Paper
正在生成论文摘要