Select to Better Learn: Fast and Accurate Deep Learning Using Data Selection From Nonlinear Manifolds
CVPR(2020)
Abstract
Finding a small subset of data whose linear combination spans other data points, also called column subset selection problem (CSSP), is an important open problem in computer science with many applications in computer vision and deep learning. There are some studies that solve CSSP in a polynomial time complexity w.r.t. the size of the original dataset. A simple and efficient selection algorithm with a linear complexity order, referred to as spectrum pursuit (SP), is proposed that pursuits spectral components of the dataset using available sample points. The proposed non-greedy algorithm aims to iteratively find K data samples whose span is close to that of the first K spectral components of entire data. SP has no parameter to be fine tuned and this desirable property makes it problem-independent. The simplicity of SP enables us to extend the underlying linear model to more complex models such as nonlinear manifolds and graph-based models. The nonlinear extension of SP is introduced as kernel-SP (KSP). The superiority of the proposed algorithms is demonstrated in a wide range of applications.
MoreTranslated text
Key words
polynomial time complexity,linear complexity order,spectrum pursuit,nongreedy algorithm,spectral components,nonlinear manifolds,nonlinear extension,data selection,data points,column subset selection problem,CSSP,computer science,computer vision,deep learning,kernel-SP,graph-based models
AI Read Science
Must-Reading Tree
Example
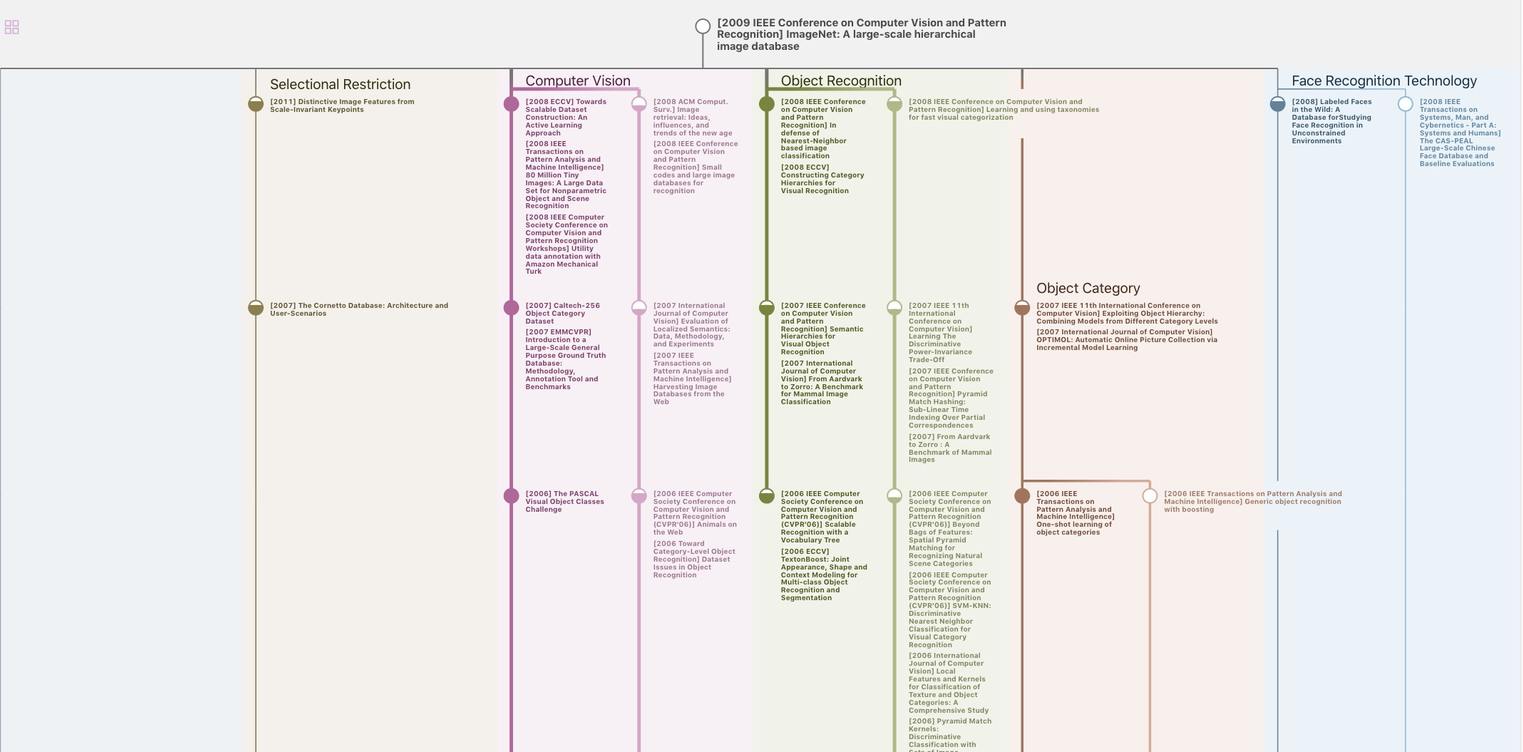
Generate MRT to find the research sequence of this paper
Chat Paper
Summary is being generated by the instructions you defined