Joint Spatial-Temporal Optimization For Stereo 3d Object Tracking
2020 IEEE/CVF CONFERENCE ON COMPUTER VISION AND PATTERN RECOGNITION (CVPR)(2020)
摘要
Directly learning multiple 3D objects motion from sequential images is difficult, while the geometric bundle adjustment lacks the ability to localize the invisible object centroid. To benefit from both the powerful object understanding skill from deep neural network meanwhile tackle precise geometry modeling for consistent trajectory estimation, we propose a joint spatial-temporal optimization-based stereo 3D object tracking method. From the network, we detect corresponding 2D bounding boxes on adjacent images and regress an initial 3D bounding box. Dense object cues (local depth and local coordinates) that associating to the object centroid are then predicted using a region-based network. Considering both the instant localization accuracy and motion consistency, our optimization models the relations between the object centroid and observed cues into a joint spatial-temporal error function. All historic cues will be summarized to contribute to the current estimation by a per-frame marginalization strategy without repeated computation. Quantitative evaluation on the KITTI tracking dataset shows our approach outperforms previous image-based 3D tracking methods by significant margins. We also report extensive results on multiple categories and larger datasets (KITTI raw and Argoverse Racking) for future benchmarking.
更多查看译文
关键词
stereo 3d object,tracking,spatial-temporal
AI 理解论文
溯源树
样例
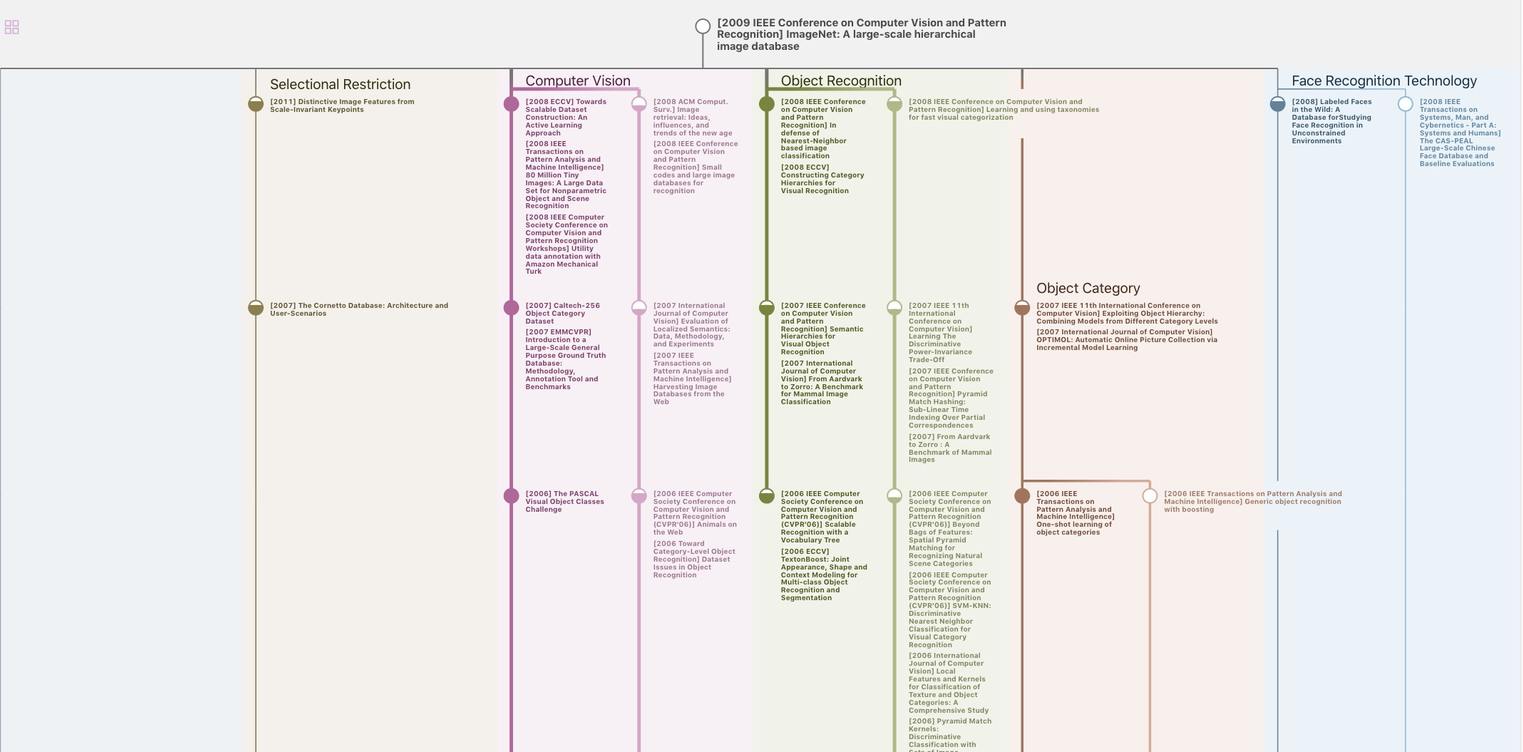
生成溯源树,研究论文发展脉络
Chat Paper
正在生成论文摘要