S3vae: Self-Supervised Sequential Vae For Representation Disentanglement And Data Generation
2020 IEEE/CVF CONFERENCE ON COMPUTER VISION AND PATTERN RECOGNITION (CVPR)(2020)
摘要
We propose a sequential variational autoencoder to learn disentangled representations of sequential data (e.g., videos and audios) under self-supervision. Specifically, we exploit the benefits of some readily accessible supervisory signals from input data itself or some off-the-shelf functional models and accordingly design auxiliary tasks for our model to utilize these signals. With the supervision of the signals, our model can easily disentangle the representation of an input sequence into static factors and dynamic factors (i.e., time-invariant and time-varying parts). Comprehensive experiments across videos and audios verify the effectiveness of our model on representation disentanglement and generation of sequential data, and demonstrate that, our model with self-supervision performs comparable to, if not better than, the fully-supervised model with ground truth labels, and outperforms state-of-the-art unsupervised models by a large margin.
更多查看译文
关键词
data generation,sequential variational autoencoder,readily accessible supervision signals,off-the-shelf functional models,time-varying part,representation disentanglement,self-supervision performs,fully-supervised model,unsupervised models,S3VAE,self-supervised sequential VAE,time-invariant part
AI 理解论文
溯源树
样例
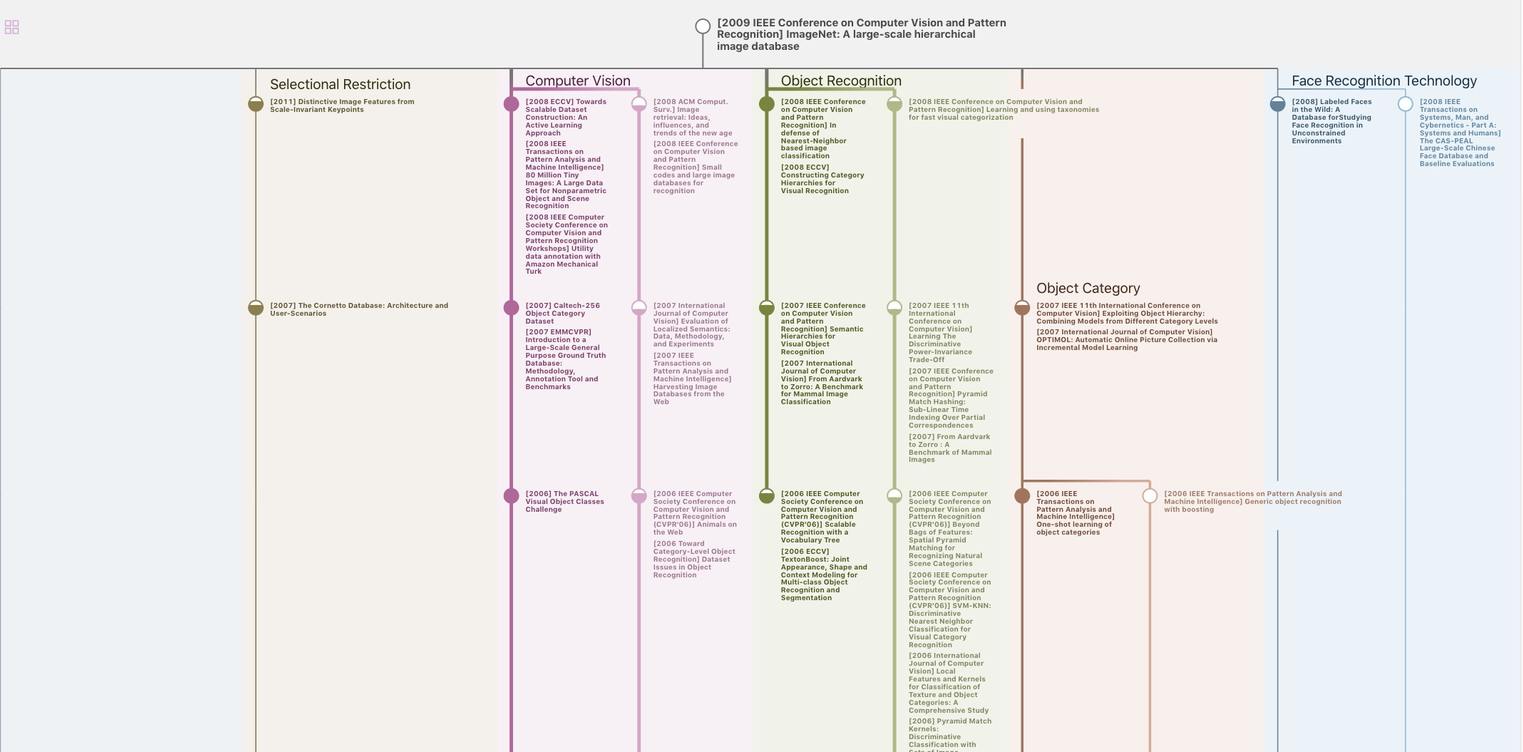
生成溯源树,研究论文发展脉络
Chat Paper
正在生成论文摘要