Attention Mechanism Exploits Temporal Contexts: Real-Time 3d Human Pose Reconstruction
2020 IEEE/CVF CONFERENCE ON COMPUTER VISION AND PATTERN RECOGNITION (CVPR)(2020)
摘要
We propose a novel attention-based framework for 3D human pose estimation from a monocular video. Despite the general success of end-to-end deep learning paradigms, our approach is based on two key observations: (1) temporal incoherence and jitter are often yielded from a single frame prediction; (2) error rate can be remarkably reduced by increasing the receptive field in a video. Therefore, we design an attentional mechanism to adaptively identify significant frames and tensor outputs from each deep neural net layer, leading to a more optimal estimation. To achieve large temporal receptive fields, multi-scale dilated convolutions are employed to model long-range dependencies among frames. The architecture is straightforward to implement and can be flexibly adopted for real-time applications. Any off-the-shelf 2D pose estimation system, e.g. Mocap libraries, can be easily integrated in an adhoc fashion. We both quantitatively and qualitatively evaluate our method on various standard benchmark datasets (e.g. Human3.6M, HumanEva). Our method considerably outperforms all the state-of-the-art algorithms up to 8% error reduction (average mean per joint position error: 34.7) as compared to the best-reported results. Code is available at: (https :// github. com/1rxjason/Attention3DHumanPose)
更多查看译文
关键词
multiscale dilated convolution,ad-hoc fashion,3D human pose estimation,2D pose estimation system,deep neural net layer,jitter,deep learning paradigms,monocular video
AI 理解论文
溯源树
样例
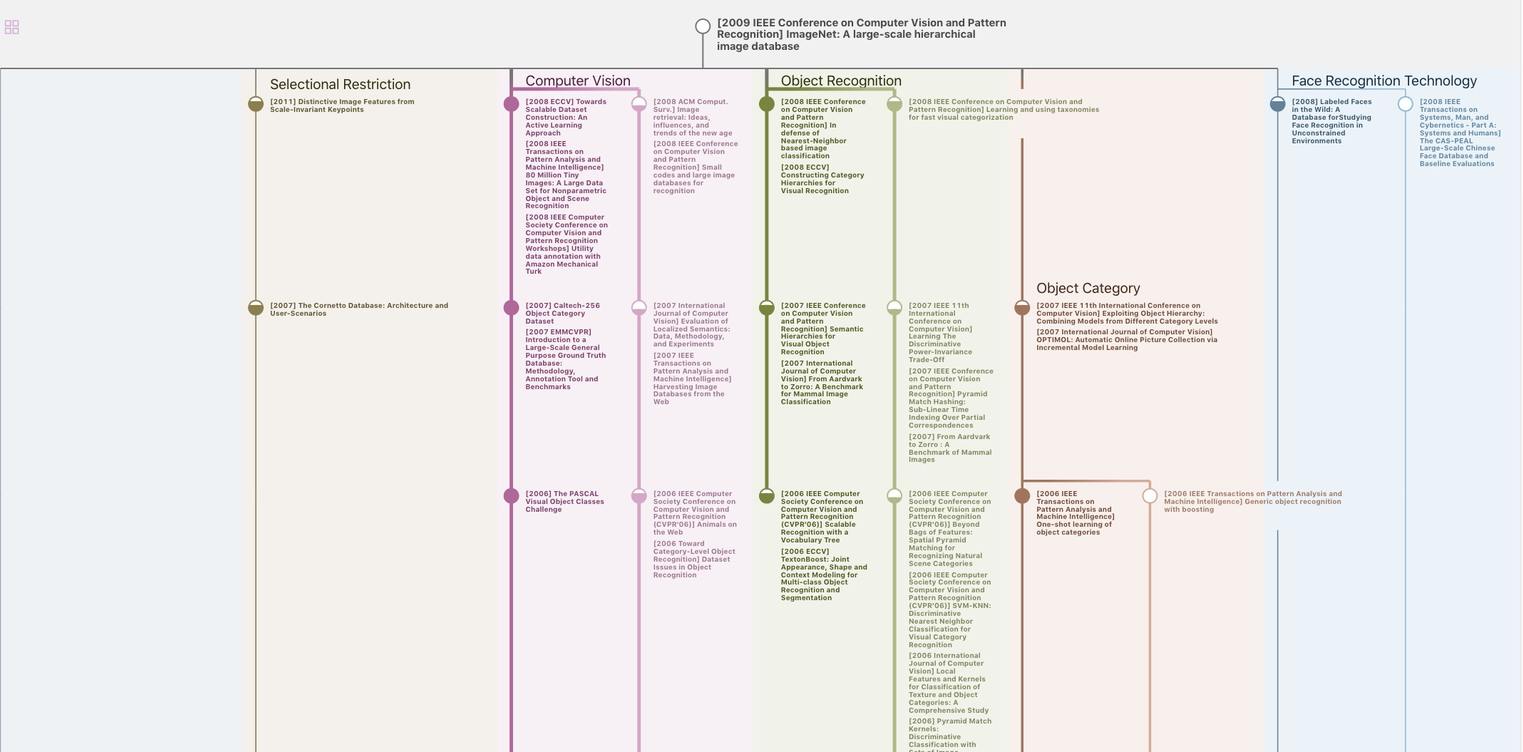
生成溯源树,研究论文发展脉络
Chat Paper
正在生成论文摘要