Reda:Reinforced Differentiable Attribute For 3d Face Reconstruction
2020 IEEE/CVF CONFERENCE ON COMPUTER VISION AND PATTERN RECOGNITION (CVPR)(2020)
摘要
The key challenge for 3D face shape reconstruction is to build the correct dense face correspondence between the deformable mesh and the single input image. Given the ill-posed nature, previous works heavily rely on prior knowledge (such as 3DMM [2]) to reduce depth ambiguity. Although impressive result has been made recently [42, 14, 8] there is still a large room to improve the correspondence so that projected face shape better aligns with the silhouette of each face region (i.e, eye, mouth, nose, cheek, etc.) on the image. To further reduce the ambiguities, we present a novel framework called "Reinforced Differentiable Attributes" ("ReDA") which is more general and effective than previous Differentiable Rendering ("DR"). Specifically, we first extend from color to more broad attributes, including the depth and the face parsing mask. Secondly, unlike the previous Z-buffer rendering, we make the rendering to be more differentiable through a set of convolution operations with multi-scale kernel sizes. In the meanwhile, to make "ReDA" to be more successful for 3D face reconstruction, we further introduce a new free form deformation layer that sits on top of 3DMM to enjoy both the prior knowledge and out-of-space modeling. Both techniques can be easily integrated into existing 3D face reconstruction pipeline. Extensive experiments on both RGB and RGB-D datasets show that our approach outperforms prior arts.
更多查看译文
关键词
multiscale kernel sizes,projected face shape,dense face correspondence,3D face reconstruction pipeline,Differentiable Rendering,broad attributes,face parsing mask,broad attributes,Reinforced Differentiable Attributes,face region,depth ambiguity,single input image,deformable mesh,3D face shape reconstruction,3DMM,free-form deformation layer
AI 理解论文
溯源树
样例
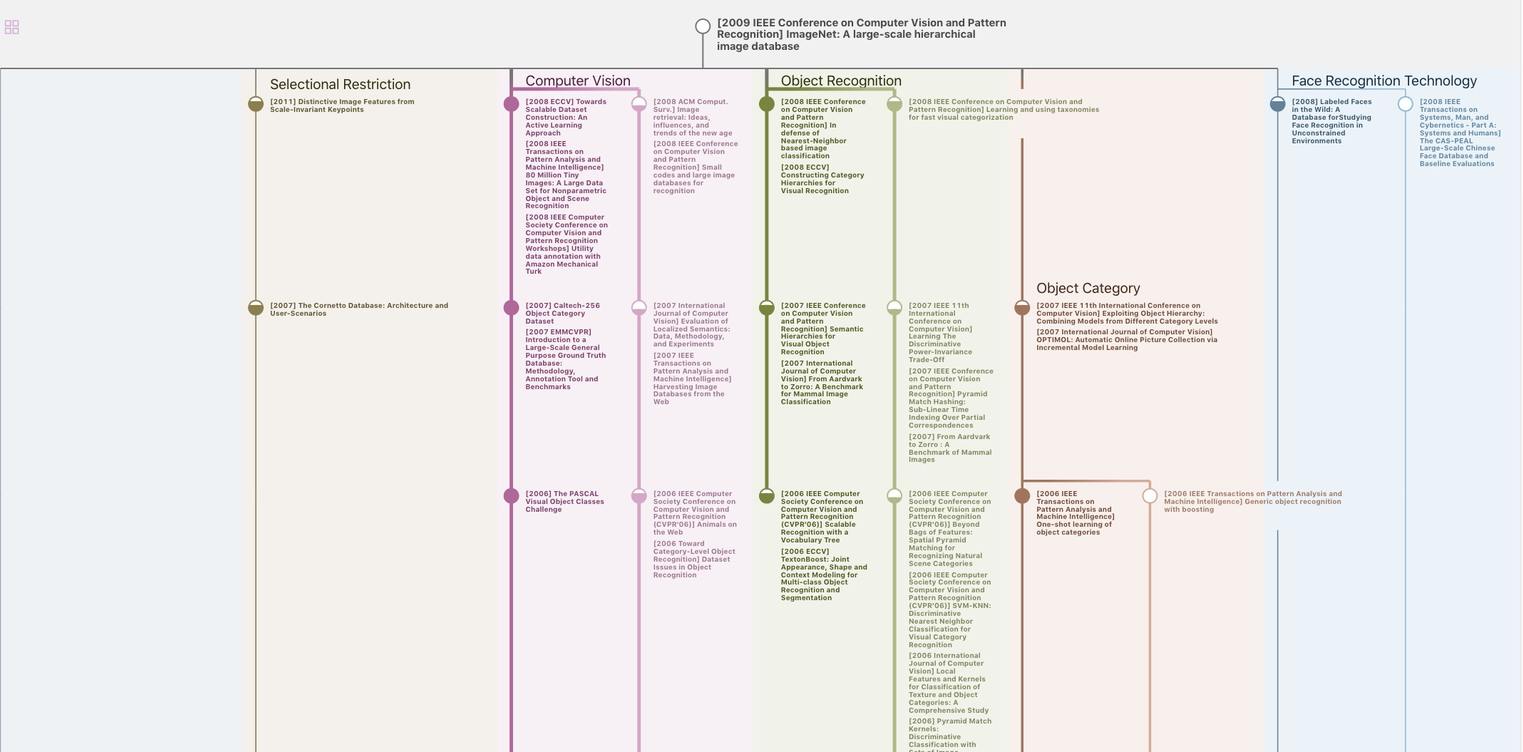
生成溯源树,研究论文发展脉络
Chat Paper
正在生成论文摘要