Blindly Assess Image Quality In The Wild Guided By A Self-Adaptive Hyper Network
2020 IEEE/CVF CONFERENCE ON COMPUTER VISION AND PATTERN RECOGNITION (CVPR)(2020)
摘要
Blind image quality assessment (BIQA) for authentically distorted images has always been a challenging problem, since images captured in the wild include varies contents and diverse types of distortions. The vast majority of prior BIQA methods focus on how to predict synthetic image quality, but fail when applied to real-world distorted images. To deal with the challenge, we propose a self-adaptive hyper network architecture to blind assess image quality in the wild. We separate the IQA procedure into three stages including content understanding, perception rule learning and quality predicting. After extracting image semantics, perception rule is established adaptively by a hyper network, and then adopted by a quality prediction network. In our model, image quality can be estimated in a self-adaptive manner, thus generalizes well on diverse images captured in the wild. Experimental results verify that our approach not only outperforms the state-of-the-art methods on challenging authentic image databases but also achieves competing performances on synthetic image databases, though it is not explicitly designed for the synthetic task.
更多查看译文
关键词
blind assess image quality,quality prediction network,diverse images,authentic image databases,synthetic image databases,blind image quality assessment,authentically distorted images,BIQA,synthetic image quality,real-world distorted images,self-adaptive hyper network architecture,image in the wild,perception rule learning,image semantics extraction
AI 理解论文
溯源树
样例
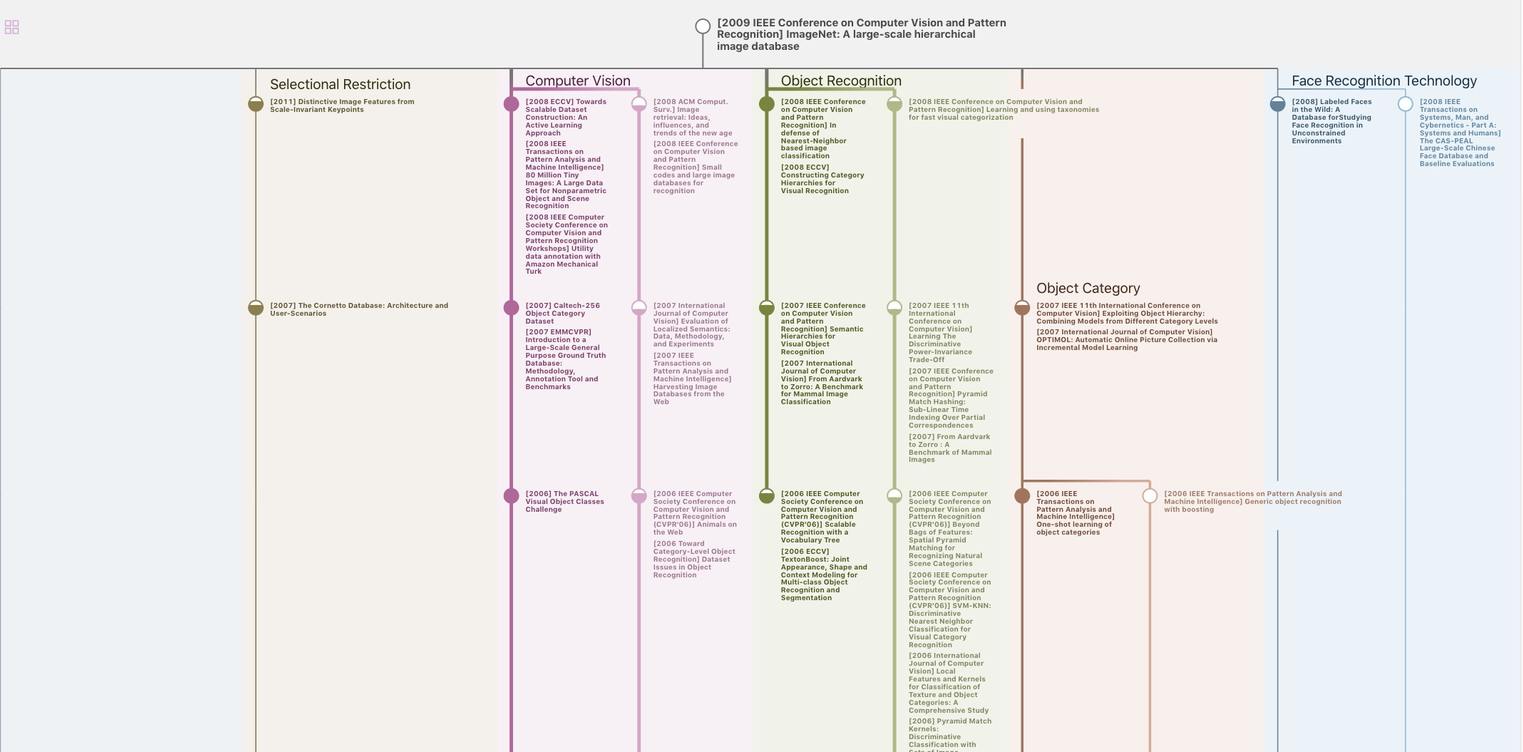
生成溯源树,研究论文发展脉络
Chat Paper
正在生成论文摘要