Select, Supplement And Focus For Rgb-D Saliency Detection
2020 IEEE/CVF CONFERENCE ON COMPUTER VISION AND PATTERN RECOGNITION (CVPR)(2020)
摘要
Depth data containing a preponderance of discriminative power in location have been proven beneficial for accurate saliency prediction. However, RGB-D saliency detection methods are also negatively influenced by randomly distributed erroneous or missing regions on the depth map or along the object boundaries. This offers the possibility of achieving more effective inference by well designed models. In this paper; we propose a new framework for accurate RGB-D saliency detection taking account of global location and local detail complementarities from two modalities. This is achieved by designing a complimentary interaction module (CIM) to discriminatively select useful representation from the RGB and depth data, and effectively integrate cross-modal features. Benefiting from the proposed CIM, the fused features can accurately locate salient objects with fine edge details. Moreover, we propose a compensation-aware loss to improve the network's confidence in detecting hard samples. Comprehensive experiments on six public datasets demonstrate that our method outperforms 18 state-of-the-art methods.
更多查看译文
关键词
RGB-D saliency detection methods,randomly distributed erroneous regions,missing regions,depth map,object boundaries,complimentary interaction model,compensation-aware loss,CIM,fused features,fine edge details,global location,local detail complementarities,cross-modal features
AI 理解论文
溯源树
样例
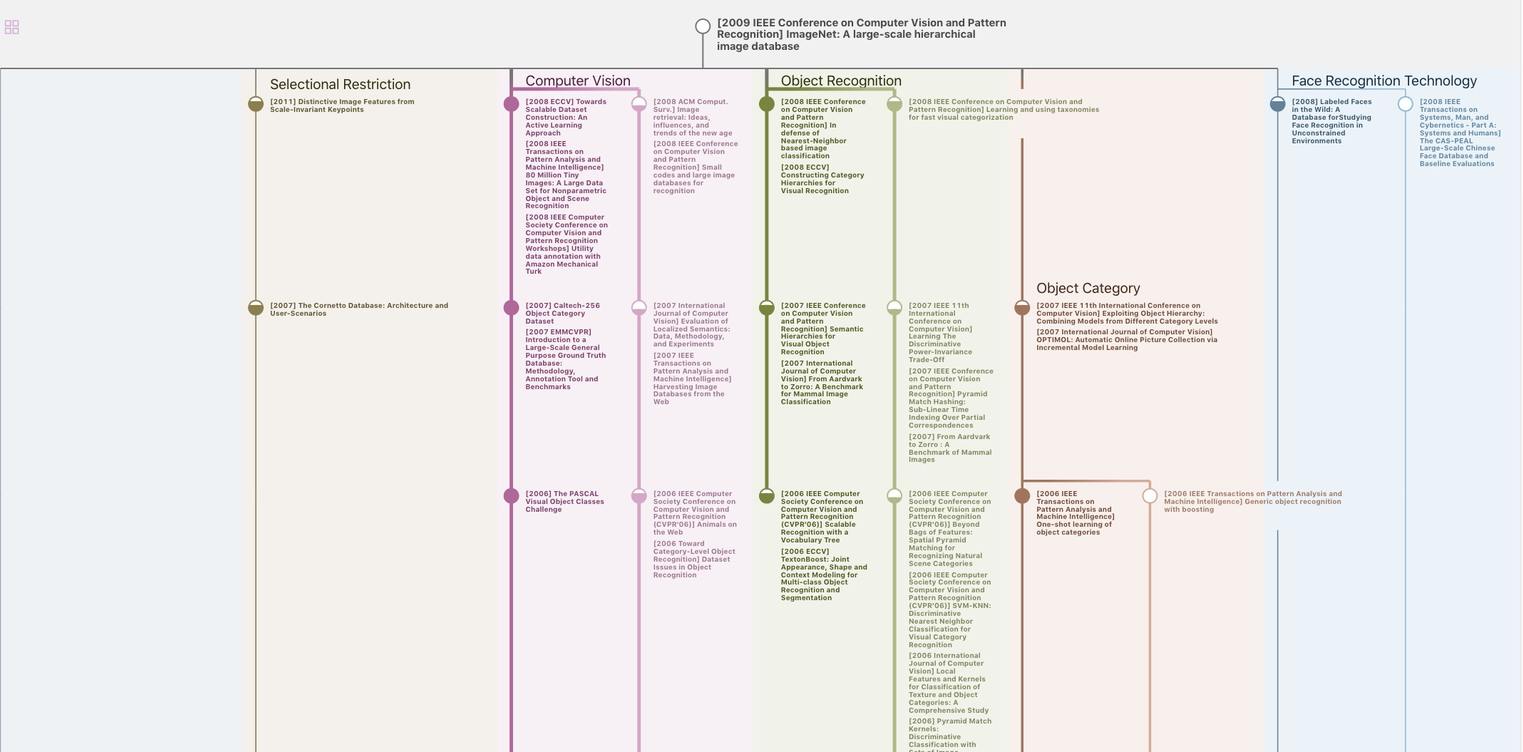
生成溯源树,研究论文发展脉络
Chat Paper
正在生成论文摘要