Spatial-Temporal Graph Convolutional Network For Video-Based Person Re-Identification
2020 IEEE/CVF CONFERENCE ON COMPUTER VISION AND PATTERN RECOGNITION (CVPR)(2020)
摘要
While video-based person re-identification (Re-ID) has drawn increasing attention and made great progress in recent years, it is still very challenging to effectively overcome the occlusion problem and the visual ambiguity problem for visually similar negative samples. On the other hand, we observe that different frames of a video can provide complementary information for each other, and the structural information of pedestrians can provide extra discriminative cues for appearance features. Thus, modeling the temporal relations of different frames and the spatial relations within a frame has the potential for solving the above problems. In this work, we propose a novel Spatial-Temporal Graph Convolutional Network (STGCN) to solve these problems. The STGCN includes two GCN branches, a spatial one and a temporal one. The spatial branch extracts structural information of a human body. The temporal branch mines discriminative cues from adjacent frames. By jointly optimizing these branches, our model extracts robust spatialtemporal information that is complementary with appearance information. As shown in the experiments, our model achieves state-of-the-art results on MARS and DukeMTMC-VideoReID datasets.
更多查看译文
关键词
Re-ID,GCN branches,pedestrian structural information,discriminative cues mining,robust spatial-temporal information model extraction,STGCN,video-based person re-identification,temporal branch,spatial branch extracts structural information,spatial-temporal graph convolutional network,spatial relations,temporal relations,complementary information,visually similar negative samples,visual ambiguity problem,occlusion problem
AI 理解论文
溯源树
样例
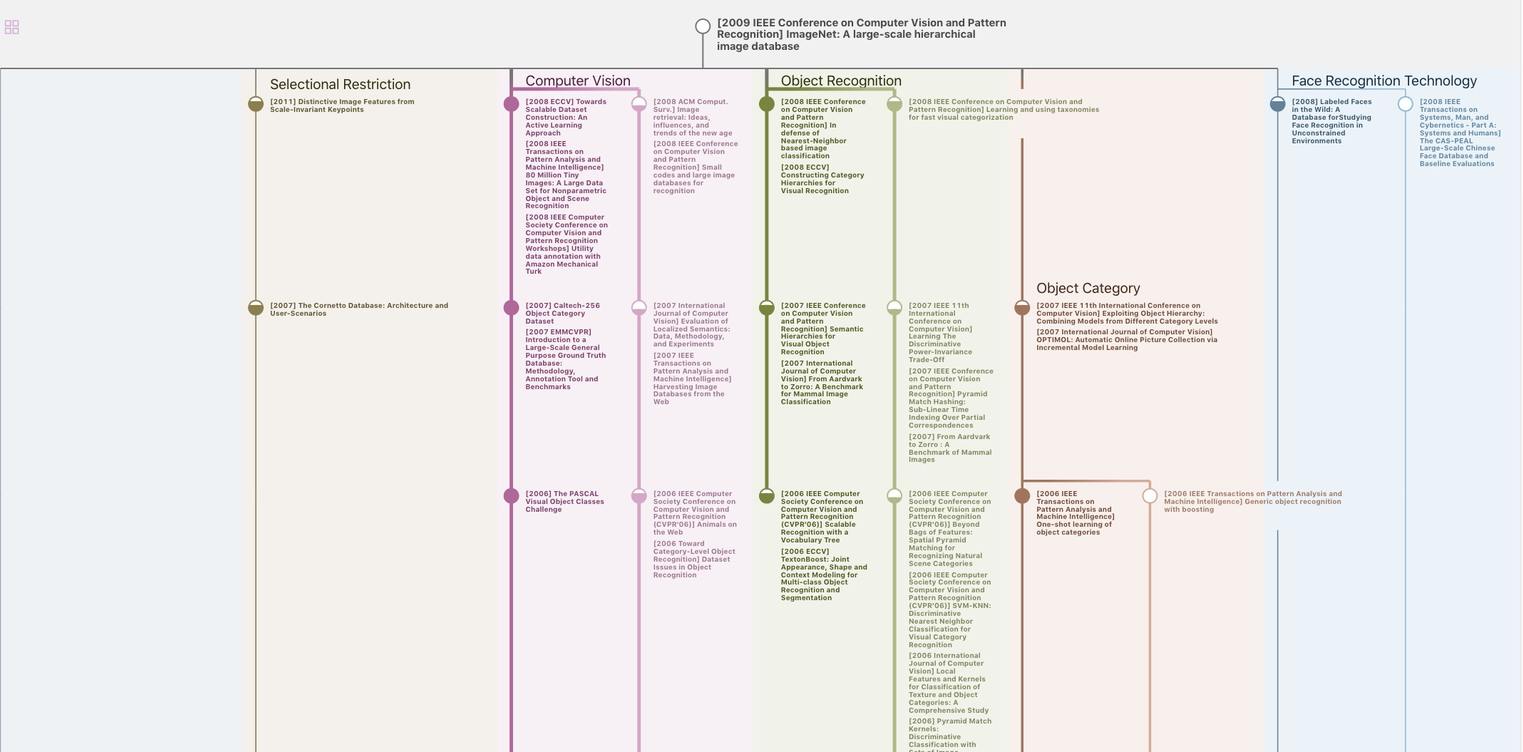
生成溯源树,研究论文发展脉络
Chat Paper
正在生成论文摘要