Multi-Domain Learning For Accurate And Few-Shot Color Constancy
2020 IEEE/CVF CONFERENCE ON COMPUTER VISION AND PATTERN RECOGNITION (CVPR)(2020)
摘要
Color constancy is an important process in camera pipeline to remove the color bias of captured image caused by scene illumination. Recently, significant improvements in color constancy accuracy have been achieved by using deep neural networks (DNNs). However, existing DNN-based color constancy methods learn distinct mappings for different cameras, which require a costly data acquisition process for each camera device. In this paper, we start a pioneer work to introduce multi-domain learning to color constancy area. For different camera devices, we train a branch of networks which share the same feature extractor and illuminant estimator, and only employ a camera-specific channel re-weighting module to adapt to the camera-specific characteristics. Such a multi-domain learning strategy enables us to take benefit from cross-device training data. The proposed multi-domain learning color constancy method achieved state-of-the-art performance on three commonly used benchmark datasets. Furthermore, we also validate the proposed method in a few-shot color constancy setting. Given a new unseen device with limited number of training samples, our method is capable of delivering accurate color constancy by merely learning the camera-specific parameters from the few-shot dataset. Our project page is publicly available at https://github.com/msxiaojin/MDLCC.
更多查看译文
关键词
camera pipeline,scene illumination,deep neural networks,DNNbased color constancy methods,data acquisition process,camera-specific channel re-weighting module,crossdevice training data,multidomain learning color constancy method
AI 理解论文
溯源树
样例
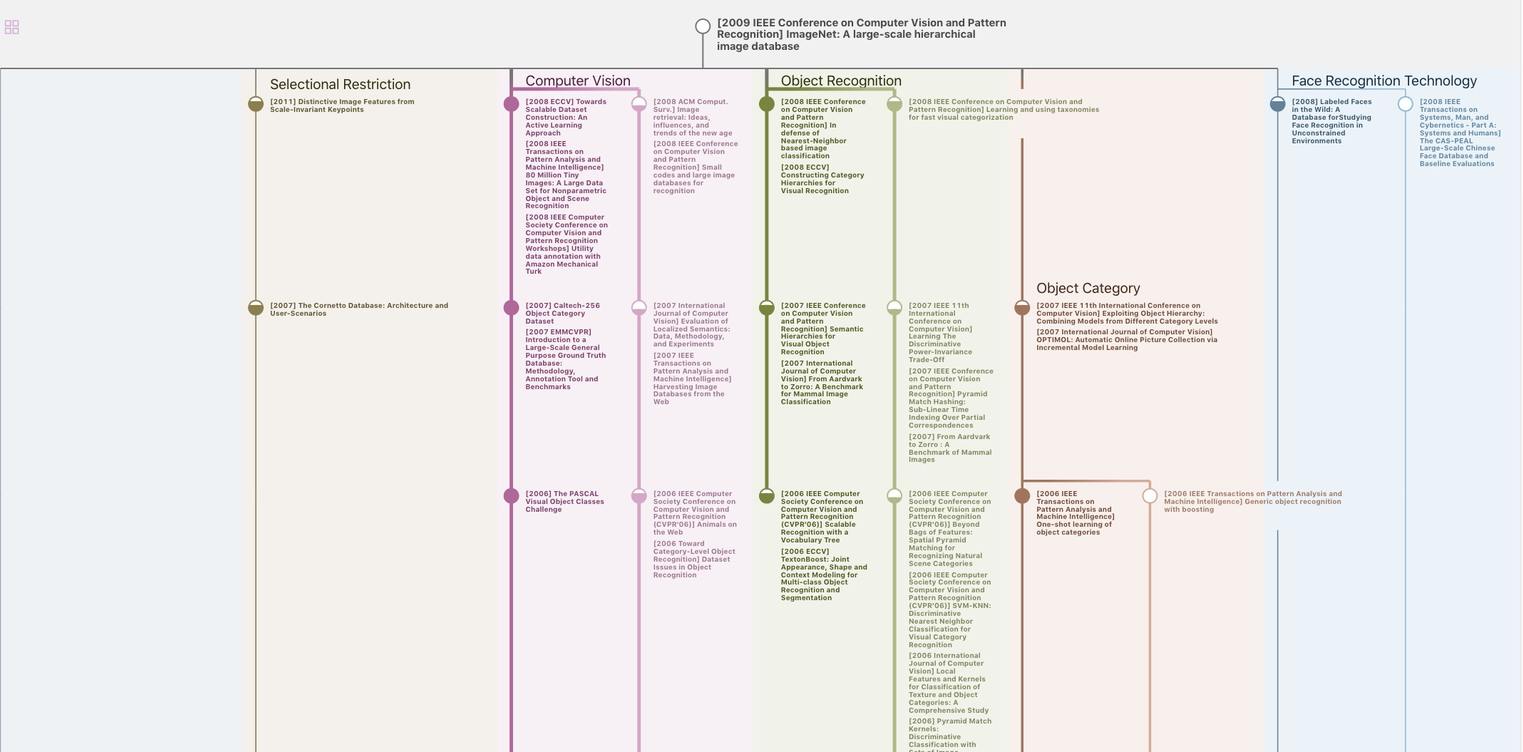
生成溯源树,研究论文发展脉络
Chat Paper
正在生成论文摘要