SSRNet: Scalable 3D Surface Reconstruction Network
IEEE TRANSACTIONS ON VISUALIZATION AND COMPUTER GRAPHICS(2023)
摘要
Learning-based surface reconstruction methods have received considerable attention in recent years due to their excellent expressiveness. However, existing learning-based methods lack scalability in processing large-scale point clouds. This paper proposes a novel scalable learning-based 3D surface reconstruction method based on octree, called SSRNet. SSRNet works in a scalable reconstruction pipeline, which divides oriented point clouds into different local parts and then processes them in parallel. Accommodating this scalable design pattern, SSRNet constructs local geometric features for octree vertices. Such features comprise the relation between the vertices and the implicit surface, ensuring geometric perception. Focusing on local geometric information also enables the network to avoid the overfitting problem and generalize well on different datasets. Finally, as a learning-based method, SSRNet can process large-scale point clouds in a short time. And to further solve the efficiency problem, we provide a lightweight and efficient version that is about five times faster while maintaining reconstruction performance. Experiments show that our methods achieve state-of-the-art performance with outstanding efficiency.
更多查看译文
关键词
Surface reconstruction,Point cloud compression,Octrees,Surface treatment,Learning systems,Three-dimensional displays,Reconstruction algorithms,Surface reconstruction,implicit function,oriented point clouds,large-scale point clouds
AI 理解论文
溯源树
样例
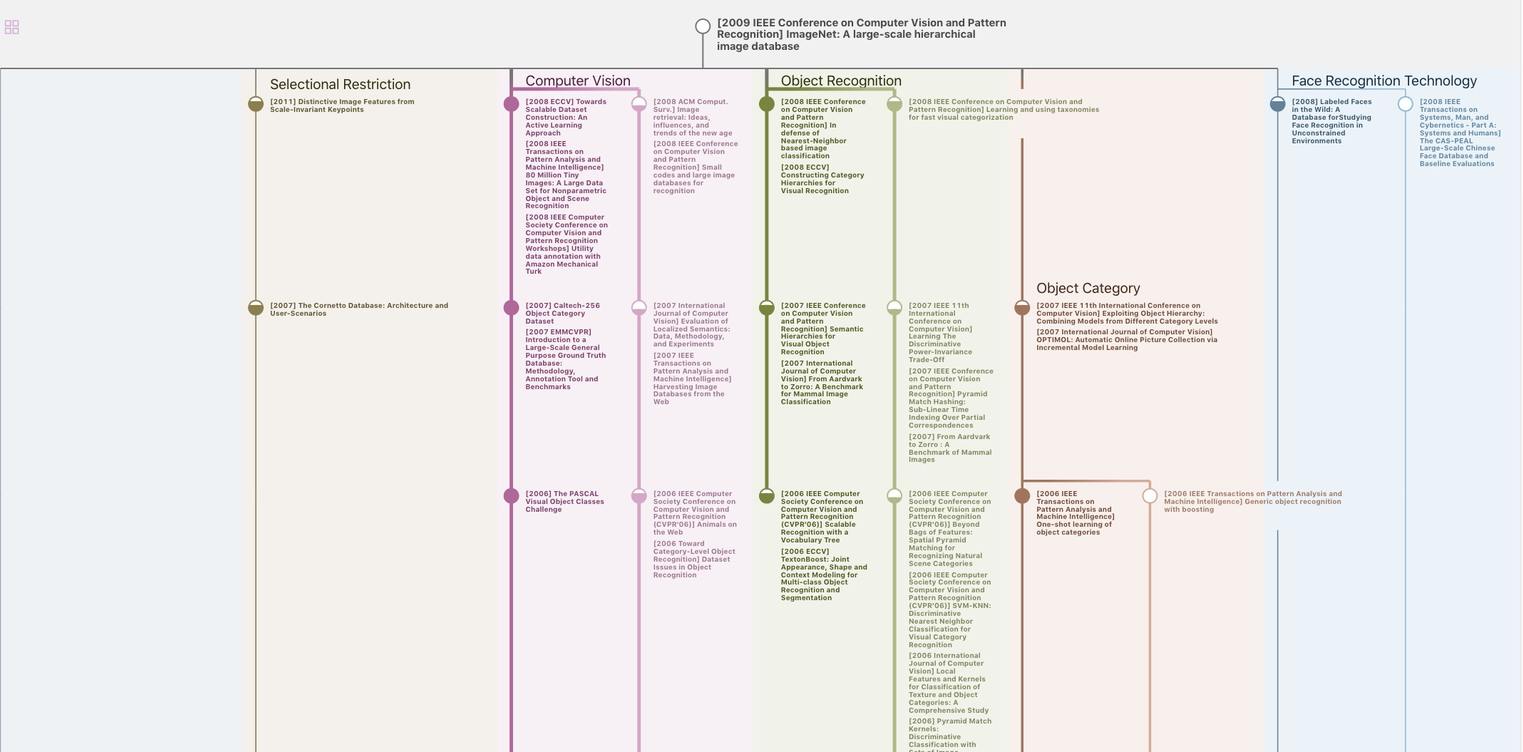
生成溯源树,研究论文发展脉络
Chat Paper
正在生成论文摘要