Active Learning Approaches for Labeling Text: Review and Assessment of the Performance of Active Learning Approaches
POLITICAL ANALYSIS(2020)
摘要
Supervised machine learning methods are increasingly employed in political science. Such models require costly manual labeling of documents. In this paper, we introduce active learning, a framework in which data to be labeled by human coders are not chosen at random but rather targeted in such a way that the required amount of data to train a machine learning model can be minimized. We study the benefits of active learning using text data examples. We perform simulation studies that illustrate conditions where active learning can reduce the cost of labeling text data. We perform these simulations on three corpora that vary in size, document length, and domain. We find that in cases where the document class of interest is not balanced, researchers can label a fraction of the documents one would need using random sampling (or "passive" learning) to achieve equally performing classifiers. We further investigate how varying levels of intercoder reliability affect the active learning procedures and find that even with low reliability, active learning performs more efficiently than does random sampling.
更多查看译文
关键词
active learning,statistical analysis of texts,supervised learning,intercoder reliability
AI 理解论文
溯源树
样例
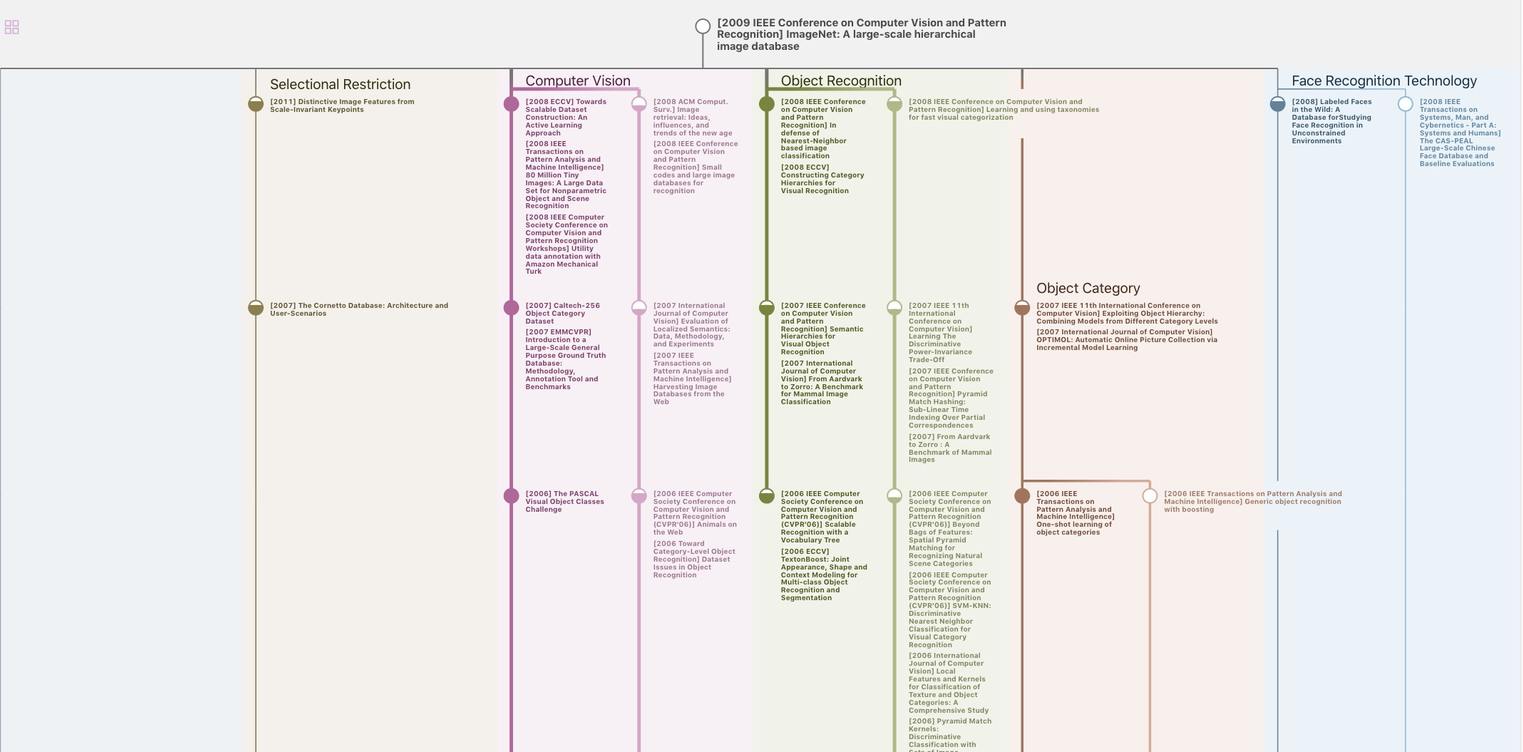
生成溯源树,研究论文发展脉络
Chat Paper
正在生成论文摘要