Covid-19 Infection And Death Rates: The Need To Incorporate Causal Explanations For The Data And Avoid Bias In Testing
JOURNAL OF RISK RESEARCH(2020)
摘要
COVID-19 testing strategies are primarily driven by medical need - focusing on people already hospitalized with significant symptoms or on people most at risk. However, such testing is highly biased because it fails to identify the extent to which COVID-19 is present in people with mild or no symptoms. If we wish to understand the true rate of COVID-19 infection and death, we need to take full account of the causal explanations for the resulting data to avoid highly misleading conclusions about infection and death rates. We describe how causal (Bayesian network) models can provide such explanations and the need to combine these with more random testing in order to achieve reliable data and predictions for the both policy makers and the public.
更多查看译文
关键词
Covid-19, biased testing, causal explanations, infection and death rates, Bayesian network models
AI 理解论文
溯源树
样例
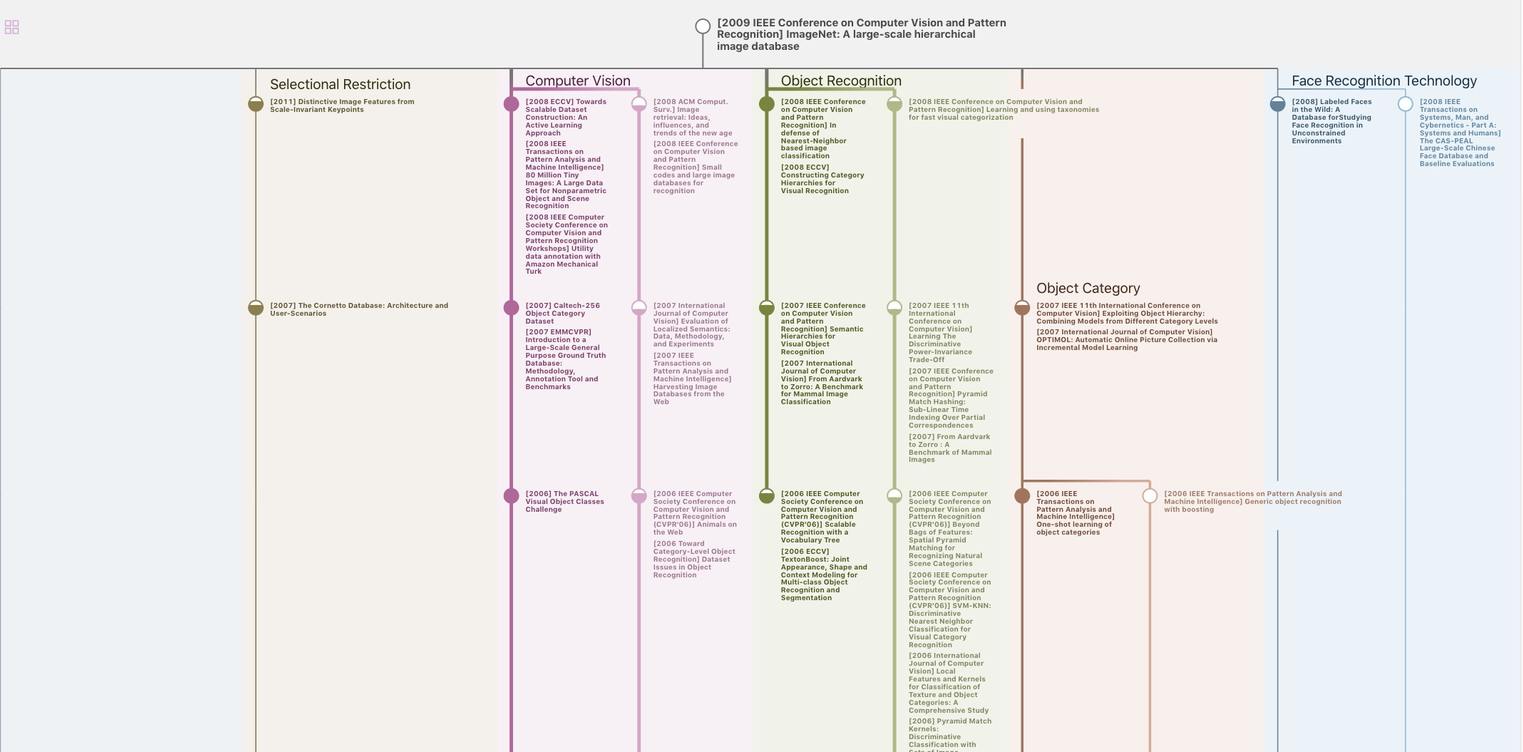
生成溯源树,研究论文发展脉络
Chat Paper
正在生成论文摘要