Inference with Nearly-Linear uncertainty models
Fuzzy Sets and Systems(2021)
摘要
Several simplified uncertainty models are derived from a given probability P0 of which they are a perturbation. Among these, we introduced in previous work Nearly-Linear (NL) models. They perform a linear affine transformation of P0 with barriers, obtaining a couple of conjugate lower/upper probabilities, and generalise several well known neighbourhood models. We classified NL models, partitioning them into three subfamilies, and established their basic consistency properties in [5]. In this paper we investigate how to extend NL models that avoid sure loss by means of their natural extension, a basic, although operationally not always simple, inferential procedure in Imprecise Probability Theory. We obtain formulae for computing directly the natural extension in a number of cases, supplying a risk measurement interpretation for one of them. The results in the paper also broaden our knowledge of NL models: we characterise when they avoid sure loss, express some of them as linear (or even convex) combinations of simpler models, and explore relationships with interval probabilities.
更多查看译文
关键词
Nearly-Linear models,Pari-Mutuel Model,Total Variation Model,Natural extension,Coherent lower probabilities,Risk measures
AI 理解论文
溯源树
样例
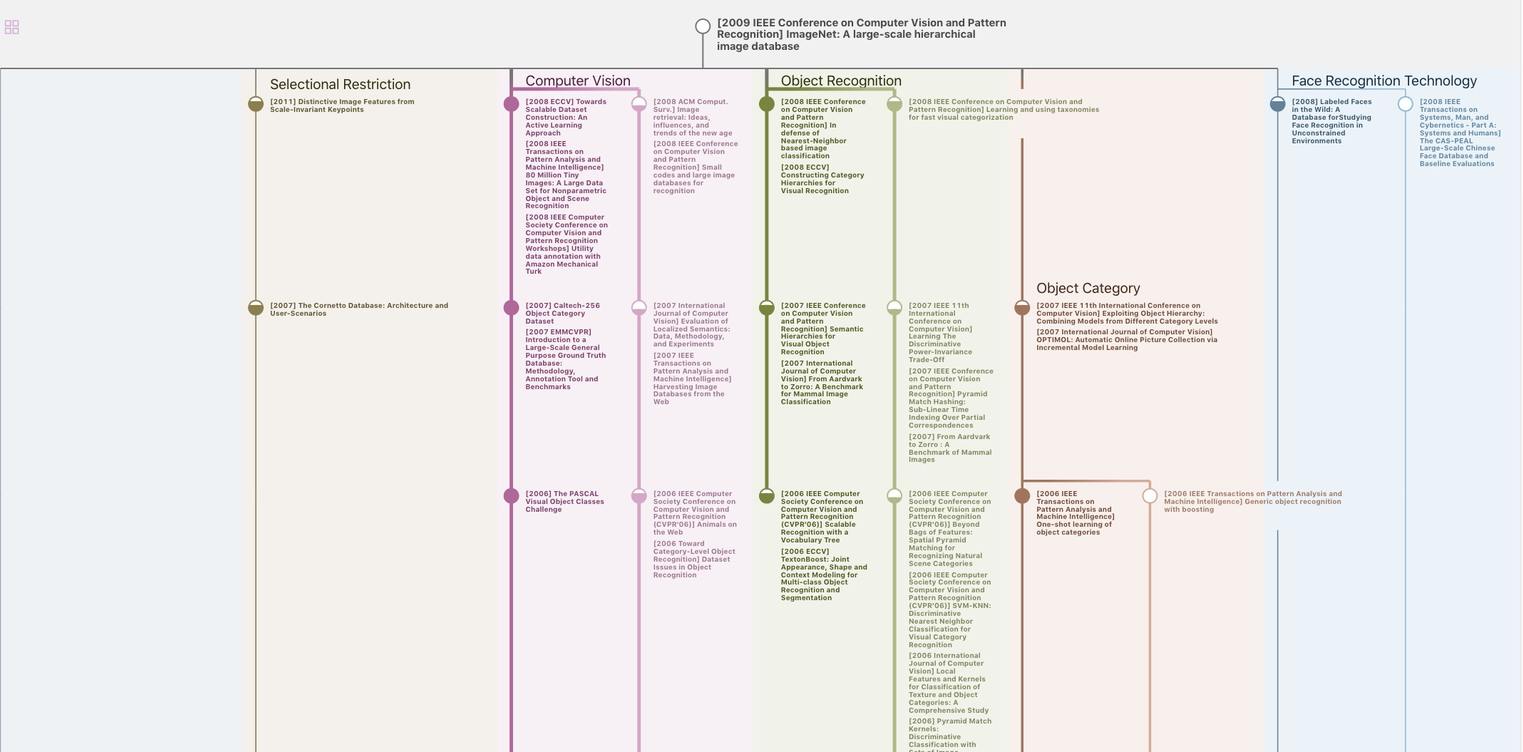
生成溯源树,研究论文发展脉络
Chat Paper
正在生成论文摘要