Get More from Less: A Hybrid Machine Learning Framework for Improving Early Predictions in STEM Education
2019 6TH INTERNATIONAL CONFERENCE ON COMPUTATIONAL SCIENCE AND COMPUTATIONAL INTELLIGENCE (CSCI 2019)(2019)
摘要
This paper builds a Machine Learning based novel classification framework that performs multi-class classification optimally when the features are scarce. It uses the context of undergraduate STEM courses for making periodic predictions of student performance at a fine-grained level. It shows that neither a vanilla multi-class classifier nor an ensemble multi-class classifier perform optimally when the number of features are small (during early predictions). The novel ML based hybrid framework predicts four classes at four equally spaced intervals during the semester: at-risk (grade below C), prone to risk (grade C), ok (grade B), and good (grade A). It uses a pipeline to single out the groups successively in the order of their increasing importance. First, it identifies the most critical group, i.e., the at-risk group. Then, it identifies the other three groups. Experimental results show that the hybrid approach outperforms the other two approaches during early predictions.
更多查看译文
关键词
Predictive model, Machine Learning, Hybrid Classifier, Framework, STEM Education, Early Warning
AI 理解论文
溯源树
样例
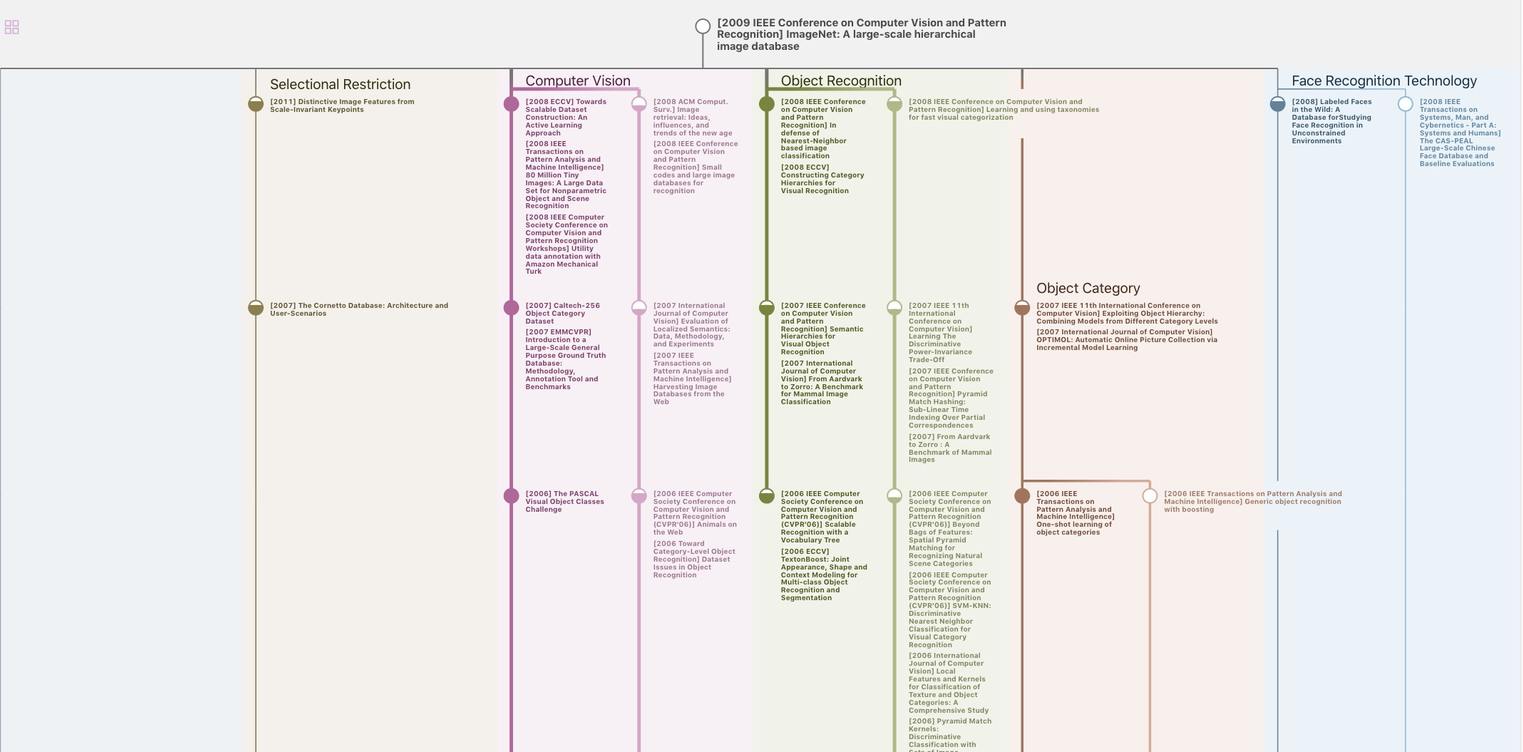
生成溯源树,研究论文发展脉络
Chat Paper
正在生成论文摘要