A Weighted Topic Model Learned From Local Semantic Space For Automatic Image Annotation
IEEE ACCESS(2020)
摘要
Automatic image annotation plays a significant role in image understanding, retrieval, classification, and indexing. Today, it is becoming increasingly important in order to annotate large-scale social media images from content-sharing websites and social networks. These social images are usually annotated by user-provided low-quality tags. The topic model is considered as a promising method to describe these weak-labeling images by learning latent representations of training samples. The recent annotation methods based on topic models have two shortcomings. First, they are difficult to scale to a large-scale image dataset. Second, they can not be used to online image repository because of continuous addition of new images and new tags. In this paper, we propose a novel annotation method based on topic model, namely local learning-based probabilistic latent semantic analysis (LL-PLSA), to solve the above problems. The key idea is to train a weighted topic model for a given test image on its semantic neighborhood consisting of a fixed number of semantically and visually similar images. This method can scale to a large-scale image database, as training samples involved in modeling are a few nearest neighbors rather than the entire database. Moreover, this proposed topic model, online customized for the test image, naturally addresses the issue of continuous addition of new images and new tags in a database. Extensive experiments on three benchmark datasets demonstrate that the proposed method significantly outperforms the state-of-the-art especially in terms of overall metrics.
更多查看译文
关键词
Training, Semantics, Image annotation, Visualization, Computational modeling, Feature extraction, Probabilistic logic, Automatic image annotation, image retrieval, probabilistic latent semantic analysis, topic model
AI 理解论文
溯源树
样例
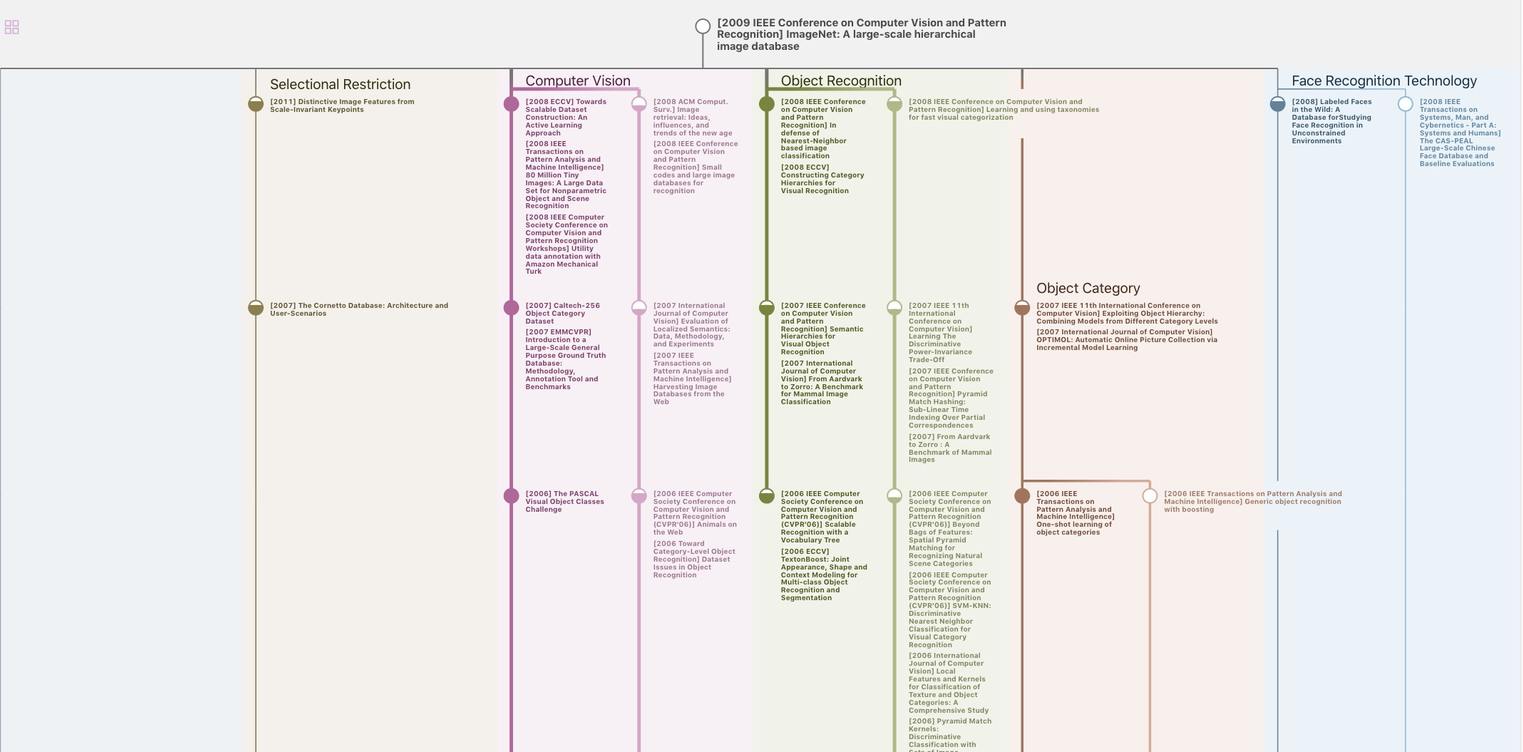
生成溯源树,研究论文发展脉络
Chat Paper
正在生成论文摘要