Understanding Skill Of Seasonal Mean Precipitation Prediction Over California During Boreal Winter And Role Of Predictability Limits
JOURNAL OF CLIMATE(2020)
摘要
Using extensive hindcasts from seasonal prediction systems participating in the North American Multi-Model Ensemble (NMME), possible causes for low skill in predicting seasonal mean precipitation over California during December-February (DJF) are investigated. The analysis focuses on investigating two possibilities for low prediction skill: role model biases or inherent predictability limits. The motivation for the analysis was the seasonal prediction during DJF 2015/16 that called for enhanced probability for above normal precipitation over southern California (which was consistent with expected conditions during an extreme El Nino) while the observed precipitation was below normal. Based on various analysis approaches and using hindcast datasets from multiple seasonal prediction systems, we build up the evidence that low skill in predicting seasonal mean precipitation over California is likely to be due to inherent predictability associated with a low signal-to-noise (SNR) regime. For the same set of seasonal prediction systems, the precipitation variability over California is contrasted with that over the southeast United States where prediction skill, as well as the SNR, is higher. The discussion also notes that building a knowledge base that goes beyond the well-known response to ENSO (based on the linear regression or composite techniques) has proven to be difficult and a systematic approach to reaching resolution to some of the overarching questions is required, and toward that end, a pathway is suggested.
更多查看译文
AI 理解论文
溯源树
样例
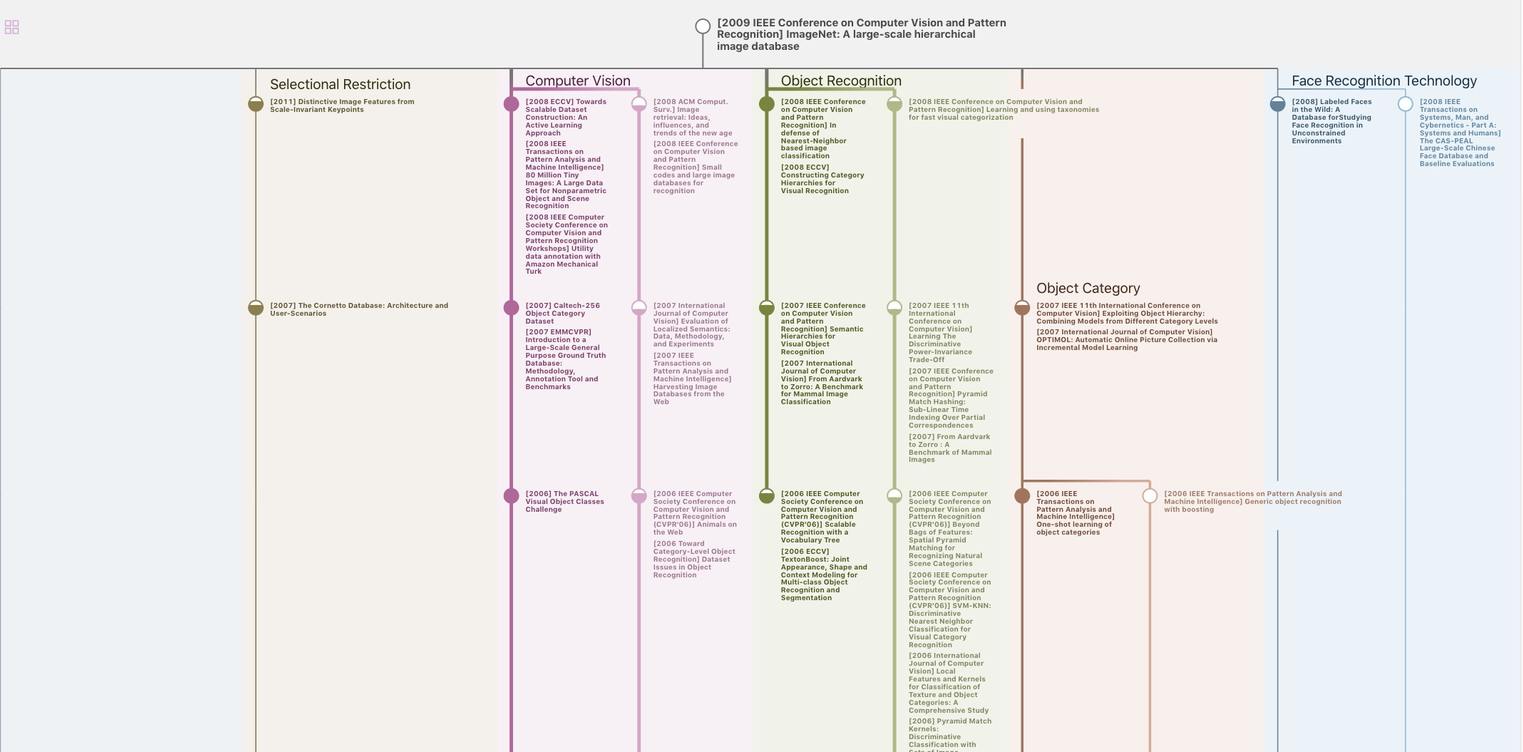
生成溯源树,研究论文发展脉络
Chat Paper
正在生成论文摘要