CACNet: Salient Object Detection via Context Aggregation and Contrast Embedding
Neurocomputing(2020)
摘要
Recently, how to adaptively exploring the most useful context information in Convolutional Neural Networks (CNNs) has been one of the most pressing problems facing the saliency detection task. In this paper, we propose a novel Context Feature Aggregation Network with Boundary Contrast Embedding (CACNet) to flexibly integrate context information without being affected by the fixed geometric structures of convolution filters. We adopt a Detail Enhancement Module (DEM) to make the network pay greater attention to the changes of image structure. A Context Adaptive Aggregation Module (CA2M) is also employed to selectively integrate the context information of the feature map. Moreover, a Boundary Contrast Loss function (BCL) enhances the discriminability of learned features by maximizing feature differences between boundary pixels. Extensive experiments on five benchmark datasets demonstrate that the proposed method outperforms other state-of-the-art methods under different evaluation metrics. During the testing stage, the network can run at about 36 FPS and does not need any post-processing.
更多查看译文
关键词
Saliency detection,Context feature aggregation,Boundary contrast loss
AI 理解论文
溯源树
样例
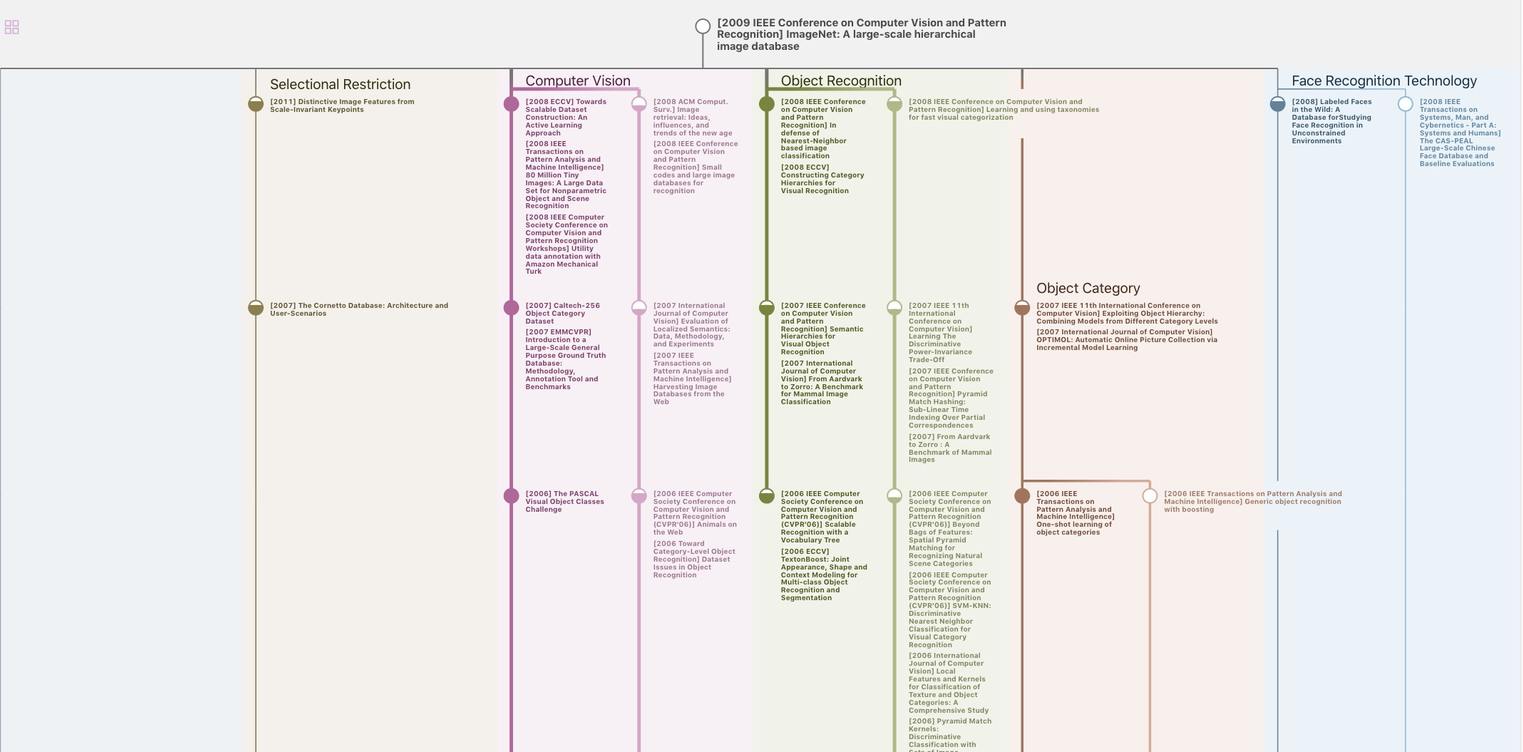
生成溯源树,研究论文发展脉络
Chat Paper
正在生成论文摘要