Semisupervised Change Detection Using Graph Convolutional Network
IEEE Geoscience and Remote Sensing Letters(2021)
摘要
Most change detection (CD) methods are unsupervised as collecting substantial multitemporal training data is challenging. Unsupervised CD methods are driven by heuristics and lack the capability to learn from data. However, in many real-world applications, it is possible to collect a small amount of labeled data scattered across the analyzed scene. Such a few scattered labeled samples in the pool of unlabeled samples can be effectively handled by graph convolutional network (GCN) that has recently shown good performance in semisupervised single-date analysis, to improve change detection performance. Based on this, we propose a semisupervised CD method that encodes multitemporal images as a graph via multiscale parcel segmentation that effectively captures the spatial and spectral aspects of the multitemporal images. The graph is further processed through GCN to learn a multitemporal model. Information from the labeled parcels is propagated to the unlabeled ones over training iterations. By exploiting the homogeneity of the parcels, the model is used to infer the label at a pixel level. To show the effectiveness of the proposed method, we tested it on a multitemporal Very High spatial Resolution (VHR) data set acquired by Pleiades sensor over Trento, Italy.
更多查看译文
关键词
Change detection (CD),deep learning,graph convolutional network (GCN),high resolution,semisupervised
AI 理解论文
溯源树
样例
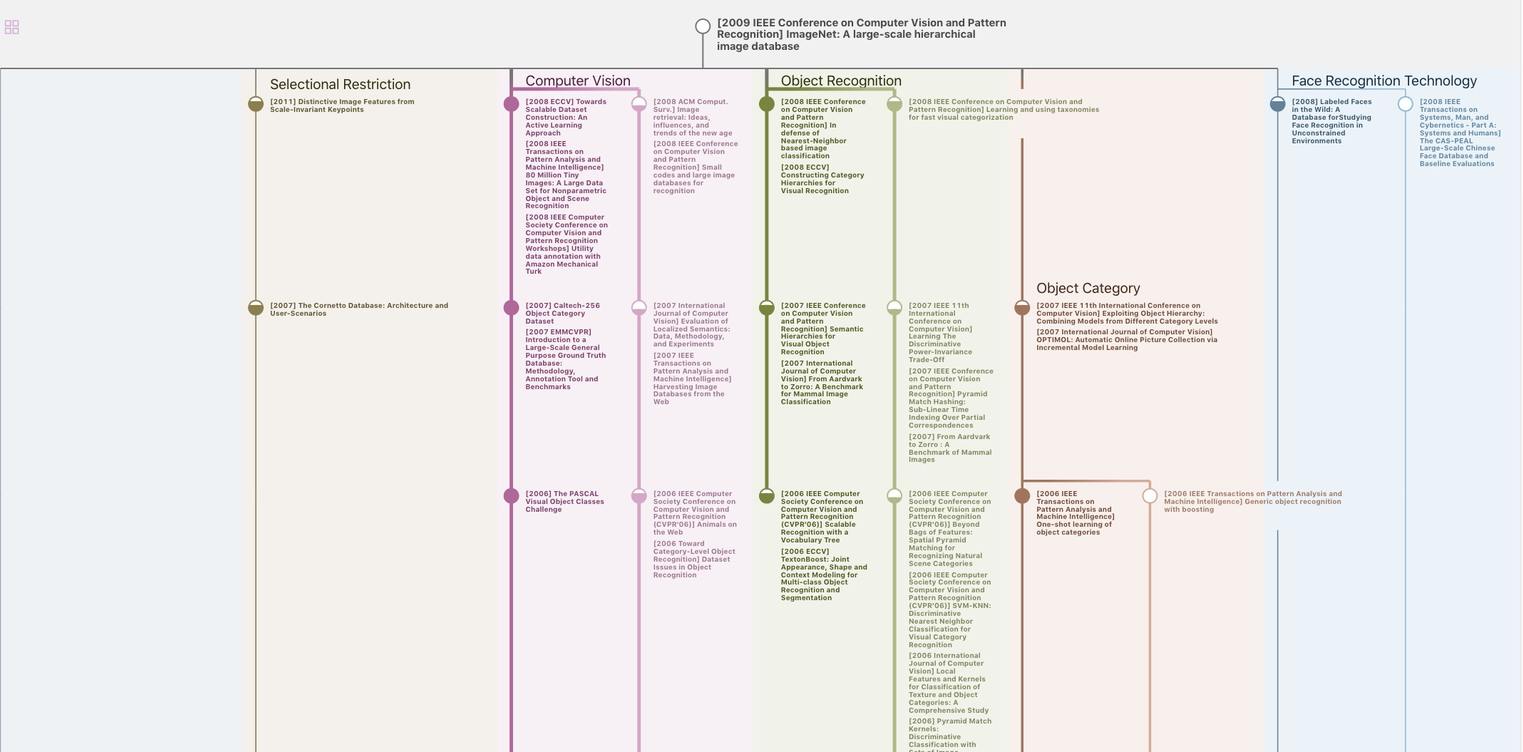
生成溯源树,研究论文发展脉络
Chat Paper
正在生成论文摘要