BEBLID: Boosted efficient binary local image descriptor
Pattern Recognition Letters(2020)
摘要
Efficient matching of local image features is a fundamental task in many computer vision applications. However, the real-time performance of top matching algorithms is compromised in computationally limited devices, such as mobile phones or drones, due to the simplicity of their hardware and their finite energy supply. In this paper we introduce BEBLID, an efficient learned binary image descriptor. It improves our previous real-valued descriptor, BELID, making it both more efficient for matching and more accurate. To this end we use AdaBoost with an improved weak-learner training scheme that produces better local descriptions. Further, we binarize our descriptor by forcing all weak-learners to have the same weight in the strong learner combination and train it in an unbalanced data set to address the asymmetries arising in matching and retrieval tasks. In our experiments BEBLID achieves an accuracy close to SIFT and better computational efficiency than ORB, the fastest algorithm in the literature.
更多查看译文
关键词
Local image descriptors,Binary descriptors,Real-time,Efficient matching,Boosting
AI 理解论文
溯源树
样例
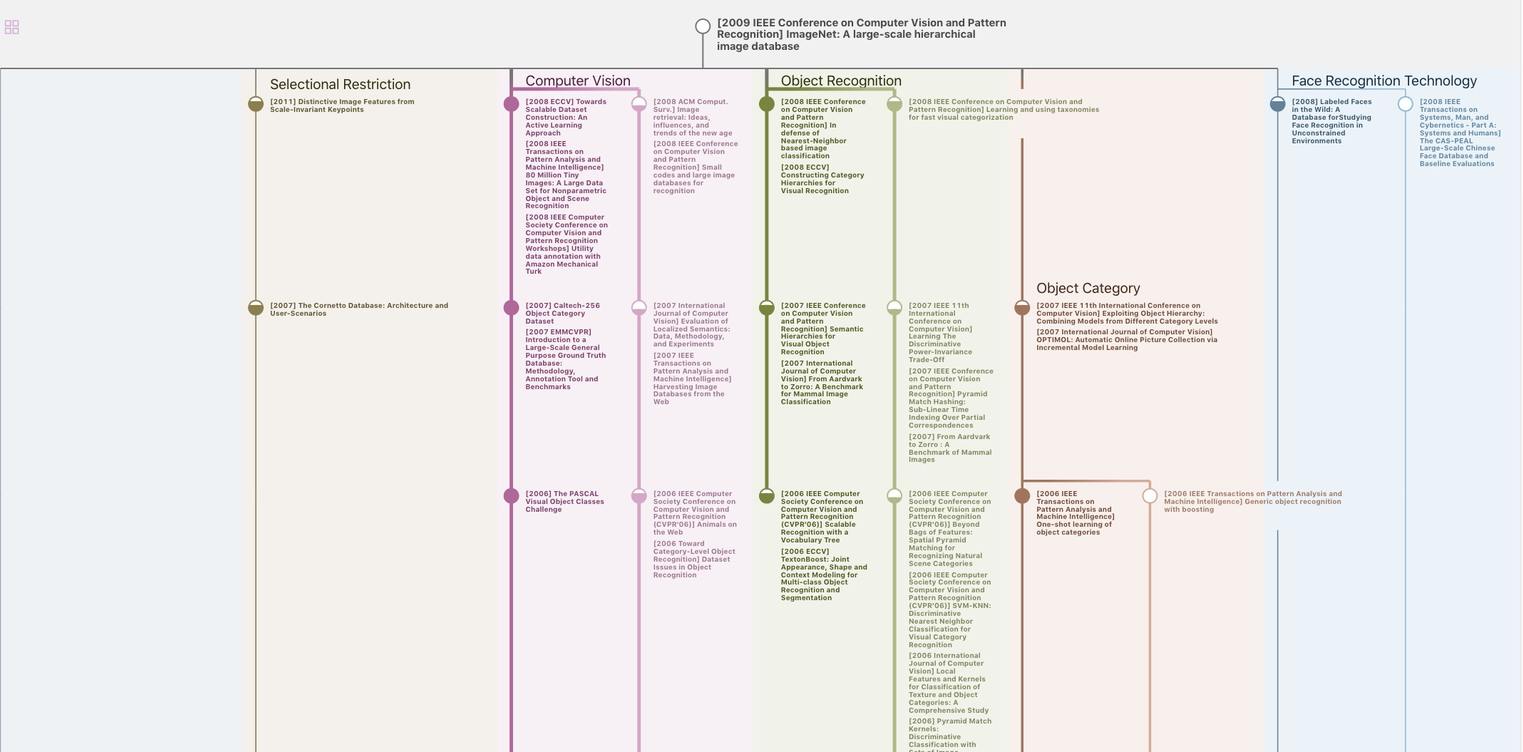
生成溯源树,研究论文发展脉络
Chat Paper
正在生成论文摘要